“Data and Analytics” is one of the top priorities for organizations. Every organization is striving hard to get the best out of it and investing more resources. However, the real question is whether we are really getting the most out of it. Most people would say yes, but it can surely be better. While most organizations are waiting to deploy augmented analytics solutions, they remain prohibitively expensive to be deployed on the scale.
One such area of Augmented Analytics is the use of AI-Powered Anomaly Detection to uncover previously undetected deviations in system and business metrics. While tech Goliaths like Amazon have used AI-powered Anomaly Detection for more than a decade now, most organizations are still unaware of the magic of using Anomaly & Outlier Detection to augment their business goals.
The current state of Business Analytics
Most businesses currently use BI dashboards (like PowerBI, Tableau, Metabase, Superset, Looker) to visualize their Key Performance Indicators (KPIs). These dashboards are generally built on top of a unified data source like a database (MySQL, PostgreSQL) or data warehouses (like Snowflake, BigQuery, Redshift). Different teams then select a handful of KPIs most important to them and select different chart types to monitor. Most dashboards are updated once a day and reviewed by teams daily or weekly and in some cases even monthly. Despite screening meticulously, it is a common occurrence to miss a critical irregularity or outage in KPIs across different dimensions by a human brain. The failings & limitations of the current Analytics Stack are leading to increasing frustrations in being able to successfully monitor one's business.
Why is the current Analytics Stack failing?
Dashboards are dead. It’s been long said that BI dashboards are failing to give leaders the level of control that they seek in their business, but it’s important to understand why.
- Multiple Data Sources: Increasingly more and more of a company’s data is sitting with 3rd party tools - CRM tools like Zendesk, Ad platforms like Google Ads, Payment providers like Stripe, Accounting tools like Quickbooks, and more. It requires heavy investment in Data Engineering to perform ETL on these data sets and store them in data warehouses. BI tools do not offer any 3rd party integrations and hence work on limited data sets making it difficult to holistically monitor one's business.
- Limited Metrics: Dashboards allow you to monitor only a handful of metrics e.g. like the performance of your top 15 products or your top 10 cities. However, a simple KPI like Revenue (across 50 products, in 50 zip codes, across 8 channels) results in (50x50x8) 20000 metrics. Relying on static dashboards gives you the top KPIs’ view but you don’t really know what’s happening to parts of your business which you aren’t proactively monitoring.
- Poor Monitoring: Current BI dashboards allow you to set monitoring thresholds (e.g. alert me when my KPI spikes by 30%); however there are two limitations of such static threshold-based alerts - First, you can set proactive alerts on only a handful of metrics (as discussed in #2) but not on all the possible or relevant dimensions. Second, more importantly, these alerts are based on static thresholds. In a dynamic business, these static alerts lead to the generation of a lot of false alerts leading to alert fatigue. It requires constant fine-tuning of these thresholds as the business grows to keep the alerts relevant. This makes it a cumbersome process.
- Manual RCA: Currently dashboards are only designed to tell you WHAT is happening to your KPIs. They do not allow you to find the RCA behind the change which becomes a manual task sometimes taking a few weeks to detect and resolve. These limitations might also lead to unresolved issues going unnoticed that can later cost a big loss or can lead to unnoticed upward trends which could have been worked upon further for topline improvement.
What is Anomaly Detection?
Anomaly is any deviation in your metrics that is abnormal and deviates in a significant way from historical data or other data in the sample set. Anomalies can be Point Anomalies (Single instance which is anomalous) or Pattern Anomalies (where a cluster of data is anomalously leading to a trend shift).
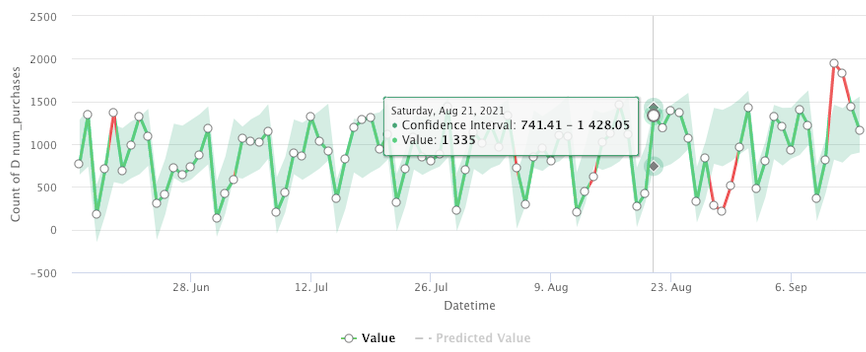
Using Machine Learning models trained on historical data, time-series datasets can be monitored for Anomaly Detection covering various nuances like daily/weekly/monthly/yearly seasonality, trends, and even data quality. Taking into account all the above factors, these models forecast a range of values that is normal. Any data point lying outside the predicted range is considered an Anomaly. The Anomalies are then scored on the basis of a severity score and users can be alerted in real-time, hence reducing the mean-time-to-detect (MTTD) and the mean-time-to-resolve (MTTR) for such outages.
How are businesses using AI-powered Anomaly Detection?
While companies like Amazon have used Anomaly Detection for over a decade now, more and more businesses are starting to adopt Anomaly detection for multiple powerful use cases across all functions like marketing, product analytics, data observability, systems monitoring. Some of the popular use cases being real-time ad campaign monitoring, fraud detection, conversion funnel monitoring, data quality monitoring, and combining systems observability with business observability.
While businesses are realizing the importance of deploying Anomaly Detection tools to enable business observability, the closed-source tools currently available make it prohibitively expensive to be deployed at scale. The future of Business Observability and unlocking the real benefits of Anomaly Detection relies on democratizing access to such tools via open-source.
Chaos Genius is an open-source business observability platform democratizing access to AI-powered Anomaly Detection for businesses around the world. Check out and access our Github Repository here. Give it a spin!