Teradata and Snowflake are two leading data warehouse platforms that offer different solutions for data storage and analysis. While both of ‘em are at the top of the food chain in data warehousing, they differ greatly in their architecture, use cases, and capabilities. Snowflake has established itself as a best-in-class cloud data warehouse, providing instant elasticity and separation of storage and compute. Teradata, on the other hand, has been a staple in the on-premises data warehousing market for over 4 decades, offering high performance and scalability for complex queries.
As of January 2025, Snowflake currently ranks 6th, whereas Teradata ranks 22nd among the most popular data warehousing platforms as per the DB-Engines ranking. Both of them have large user bases, ranging from startups to enterprises, across various industries.
In this article, we will compare Teradata vs Snowflake across 7 different key criteria—architecture, performance, workload configuration/management, integration/ecosystem, security, machine learning capabilities, programming language support, pricing—and so much more!!
Let's dive right in!!
Teradata vs Snowflake—Which One Is Better Choice for Your Needs?
Before we dive into the details of each feature, let’s start with a high-level summary of Teradata vs Snowflake and how they compare and contrast on some key aspects. The following table illustrates the main differences and similarities between Teradata and Snowflake.
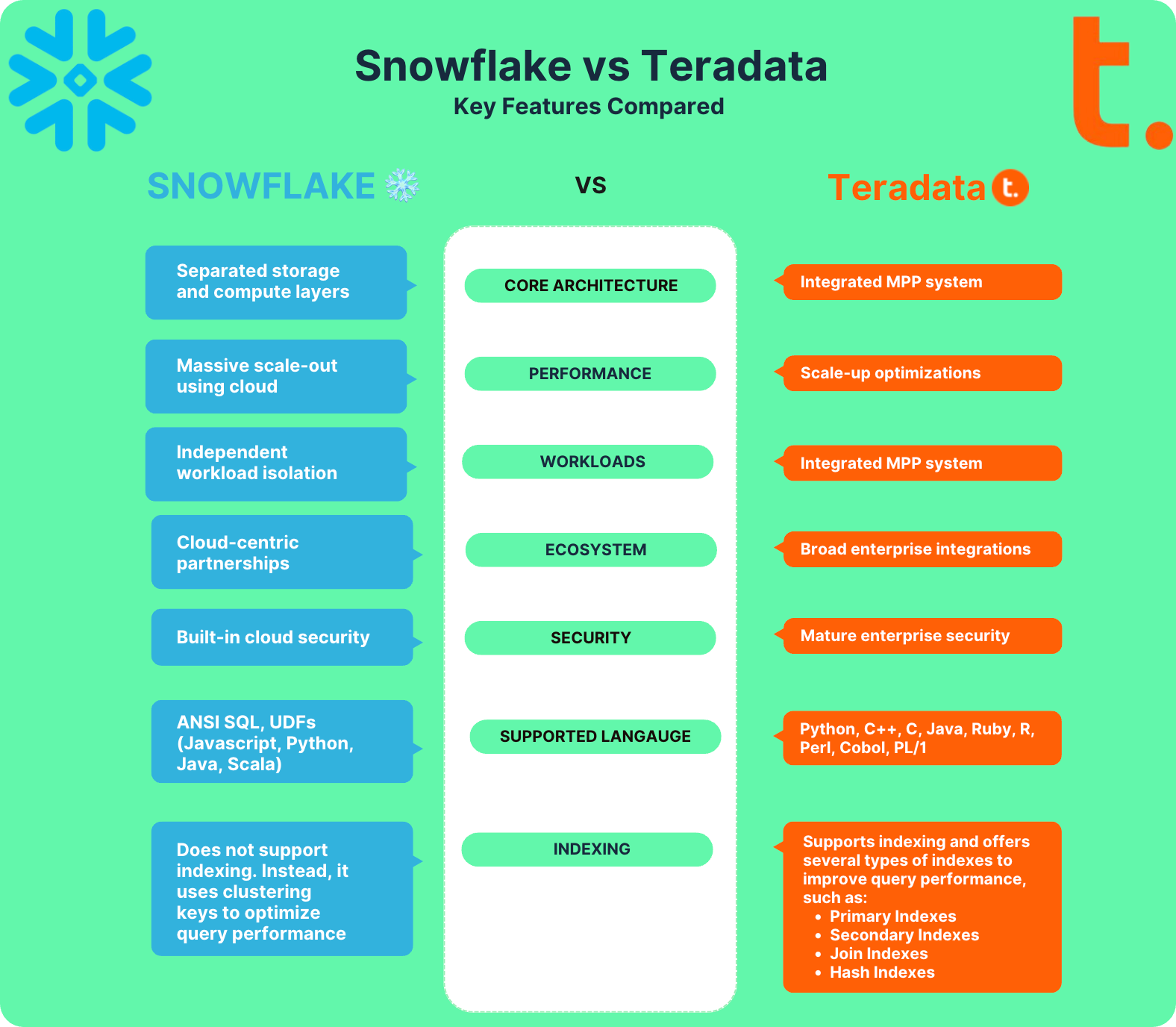
What is Teradata?
Teradata is an enterprise data warehousing solution that provides high-performance analytics and data management on a Massively Parallel Processing (MPP) system. Teradata enables users/organizations to store, process, and analyze large volumes of data from various sources and formats.
Teradata was first founded in 1979 (~45 years ago) by Jack E. Shemer, Philip M. Neches, Walter E. Muir, Jerold R. Modes, William P. Worth, Carroll Reed, and David Hartke. Teradata pioneered the concept of MPP, which distributes data and queries across multiple nodes, each with its own processor, memory, and disk, to achieve high performance, scalability—and reliability. Teradata also introduced the concept of shared-nothing architecture, which minimizes data movement and contention among the nodes and maximizes the parallelism and efficiency of the system.
Teradata has evolved from an on-premises solution to a hybrid cloud solution, offering various deployment options, such as Teradata IntelliFlex, Teradata IntelliBase, Teradata Vantage, which evolved from the Teradata Database, and so much more offerings. Teradata supports various data types and formats, such as structured, semi-structured—and unstructured data, and provides various tools and services, such as Teradata Studio & Studio Express, Teradata Data Fabric, Teradata ClearScape Analytics, and Teradata Data Lab, to enable data manipulation, analysis, integration, and management.

Some of the key features of Teradata are:
- Massively Parallel Processing (MPP) architecture: Teradata uses a Massively Parallel Processing (MPP) system that integrates storage and compute nodes in a single system, allowing for high performance, throughput—and reliability. Teradata also uses a unique hashing algorithm to distribute data evenly and randomly across the nodes, ensuring optimal load balancing and fault tolerance.
- Workload management: Workload management means managing workload performance by monitoring system activity and acting when pre-defined limits are reached. Teradata Workload Management offers two different strategies:
Teradata Active System Management (TASM): allows you to prioritize workloads, tune performance, and monitor and manage workload and system health.
Teradata Integrated Workload Management (TIWM): TIWM is a subset of TASM features.
- Data federation capabilities: Teradata provides data federation capabilities, which enable users to access and query data from various sources and formats, such as relational, non-relational, and external data, without moving or copying the data. Teradata also supports foreign tables, which allow users to access and query data stored outside of Teradata, such as in Amazon S3, Azure Blob Storage, and Google Cloud storage, as if they were regular tables in the Teradata DBs.
- Shared Nothing Architecture: Each component in Teradata works independently.
- Connectivity: Teradata can connect to Channel-attached systems such as Mainframe or Network attached systems.
- Advanced analytics functions: Teradata provides advanced analytics functions that enable users to perform complex and sophisticated analyses of the data.
- Various programming language support: Teradata also supports various programming languages, such as Python, C++, C, Java, Ruby, R, Perl, Cobol, and PL/I, to interact with the database and perform advanced analytics.
- Linear Scalability: Teradata systems are highly scalable. They can scale up to 2048 Nodes.
- Fault Tolerance: Teradata has many functions and mechanisms to handle fault tolerance. It has the protection mechanism against both hardwaree and software failure.
- Flexible pricing options: Teradata offers flexible pricing options, such as on-premises, cloud, or hybrid, and features such as elastic performance on demand, reserved instances, and pay-as-you-go, enabling users to choose the best deployment option and pricing model for their needs and budget.
To sum up, Teradata was one of the earliest vendors in the data warehousing space and helped pioneer concepts like parallel processing and real-time BI in the year 1980s. Over its 45+ year history, the company has continued to advance its platform with new functionality like in-database analytics, machine learning/AI—and hybrid cloud capabilities.
Teradata is optimized for the most intensive mixed workloads from business intelligence (BI) and reporting to artificial intelligence (AI) and high-performance analytics. Key industries it serves include retail, banking, telecom, and healthcare.
What is Snowflake?
Snowflake is a data cloud platform that enables users/organizations to store, process, and analyze large datasets affordably, rapidly—and at scale. Its unique architecture separates storage, compute, and cloud services, allowing each to scale independently. Snowflake runs on public clouds like Amazon Web Services (AWS), Google Cloud Platform (GCP), Microsoft Azure (Azure). Snowflake is the engine that provides a single, seamless experience to discover, securely share, and unite siloed data. It provides solutions for applications, collaboration, cybersecurity, data engineering, data lakes, data science, data warehousing—and enterprise requirements.
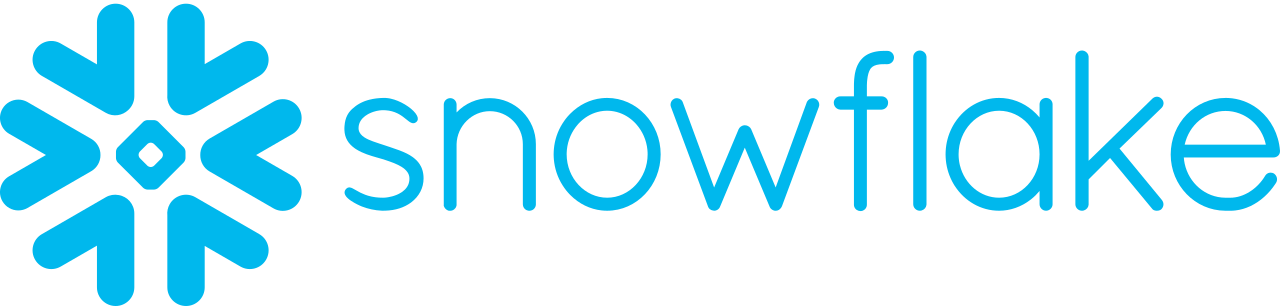
Some key architectural features and capabilities of Snowflake are:
Here are some of the key features of Snowflake:
- Scalability & Performance: Snowflake is incredibly fast and scalable. It's built specifically for the cloud, so it can easily scale up or down on demand. No matter how much data you need to store or analyze, it can handle it without slowing down. Snowflake can control exponentially higher data volumes and concurrent user queries than traditional data warehouses. Performance remains fast even with terabytes or even petabytes of data.
- Flexibility: Snowflake uses a unique multi-cluster shared data architecture. Data is held centrally, while compute is scaled out across multiple cloud regions and cloud providers, which gives you tons of flexibility in how and where you access your data. Snowflake also offers the ability to store and query both structured and semi-structured data together.
- Zero Maintenance: Snowflake fully handles infrastructure, optimization, tuning, backups, etc. You don't need to spend time managing infrastructure or waiting to tune queries. It just works, freeing you up to focus on extracting valuable insights.
- Security: Security is also a top priority for Snowflake. It offers robust security capabilities like role-based access control, network policies, end-to-end encryption—and more.
- Governance and Compliance: When it comes to governance and compliance, Snowflake has outstanding capabilities built-in. Things like replication, failover, time travel, and database cloning help meet regulatory requirements and give you peace of mind.
- Global Availability: Snowflake is deployed across major public cloud platforms (AWS, Azure, GCP) in multiple regions and availability zones for geographic coverage, allowing users to store data near end users to reduce latency.
- Concurrency and Workload Isolation: Snowflake uses a multi-cluster, shared data architecture to handle high concurrency levels and ensure fast query performance. Workloads are isolated to eliminate resource contention.
- Cloud Agnosticism: As we know, Snowflake provides full support for all major cloud platforms; it provides flexibility to operate cross-cloud, move workloads, and avoid vendor lock-in. Snowflake also offers the ability to query data across different cloud storage services.
- Collaboration: Snowflake has extensive capabilities for data sharing, data exchanges, and collaborative development, which helps enable collaboration between users, businesses, and external partners.
- Flexible Business Continuity: Snowflake's architecture ensures zero data loss and zero downtime for maintenance or failures. Automatic failover and recovery are handled transparently. Snowflake also offers configurable time travel for access to historical data.
- Elasticity: Snowflake allows storage and compute to scale up and down independently, allowing optimization for workloads and only paying for resources used. Snowflake also offers per-second billing.
- Marketplace: Snowflake provides access to a marketplace of data, data services, and applications, simplifying data acquisition and integration.
- Snowsight: Snowflake provides Snowsight, an intuitive web user interface for creating charts, dashboards, data validation, and ad-hoc data analysis.
- Time Travel: Snowflake's unique Time Travel feature allows users to query historical snapshots of their data up to 90 days in the past, enabling backfills, corrections, and historical data analysis without affecting the current state of the data.
- Pay-per-usage Model: Snowflake operates on a pay-per-use model, charging only for the resources consumed, eliminating upfront costs, and providing flexibility for scaling consumption based on workload demands.
—and much more!!
Snowflake utilizes a unique hybrid architecture that combines elements of shared disk and shared-nothing Snowflake architectures. In the storage layer, data is stored in a centralized location in the cloud, accessible by all compute nodes, similar to a shared disk architecture. However, the compute layer operates on a shared-nothing model, where independent compute clusters called virtual warehouses process queries independently.
For more details on what Snowflake is and how it works, you can read our previous article here.
Now, let's dive into the next section, where we will compare Teradata vs Snowflake across 7 different features.
Teradata vs Snowflake: 7 Critical Features You Need to Know
Teradata vs Snowflake—Which option best suits your requirements? Let's dive into the specifics and examine their essential features:
1). Teradata vs Snowflake—Architecture Breakdown
One of the most important features that distinguishes Teradata vs Snowflake is their architecture, which determines how they store, process, and access data. In this section, we will explain the architecture of each solution and compare and contrast their pros and cons.
Now, let's explore their architectural differences.
Snowflake Architecture—Teradata vs Snowflake
Snowflake utilizes a unique hybrid cloud architecture that combines elements of shared disk and shared-nothing architectures. In the storage layer, data resides in a central data repository that is accessible to all compute nodes, like a shared disk. But the compute layer uses independent virtual warehouses that process queries in parallel, like a shared-nothing architecture.
The Snowflake architecture has three layers:
1) Storage Layer: Snowflake storage layer is designed to optimize data storage and accessibility. When data is loaded into Snowflake, it is converted into an optimized, compressed, columnar format. Snowflake stores this optimized data in cloud storage. Snowflake manages all aspects of how this data is stored—the organization, file size, structure, compression, metadata, statistics, and other aspects of data storage are handled by Snowflake. The data objects stored by Snowflake are neither directly visible nor accessible by the user; they are only accessible through SQL query operations run using Snowflake.
2) Compute Layer: Snowflake's compute processing layer utilizes virtual warehouses to execute each query. Each virtual warehouse is an MPP (massively parallel processing) compute cluster, consisting of multiple compute nodes allocated by Snowflake from a cloud provider. This distributed computing environment enables Snowflake to process large volumes of data quickly and efficiently. What's cool is that each virtual warehouse operates independently without sharing compute resources with other virtual warehouses.
3) Cloud Services Layer: Snowflake cloud services layer is like the control center that oversees everything in Snowflake. It's the brains connecting all the different pieces to process user requests, ranging from login to query dispatch. This layer manages various services, including authentication, infrastructure management, metadata management, query parsing, optimization—and access control.
Note that the cloud services layer runs on compute instances provisioned by Snowflake from the cloud provider.
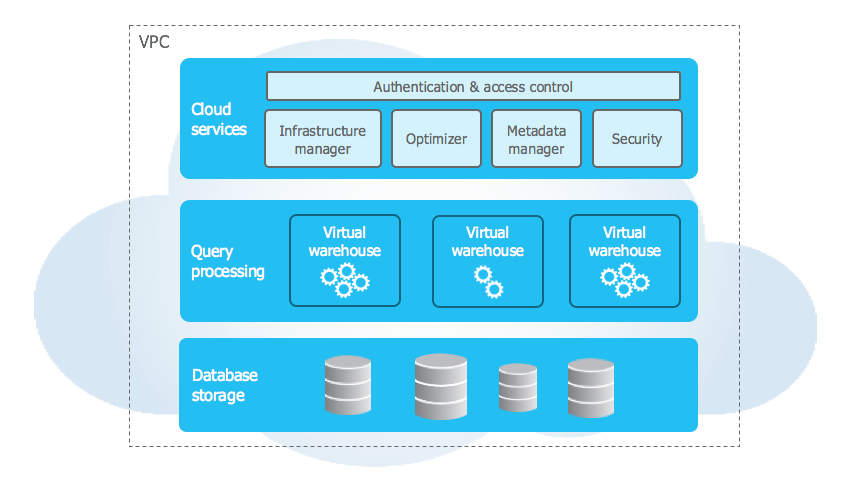
Check out this in-depth article if you want to learn more about the capabilities and architecture of Snowflake.
Teradata Architecture—Teradata vs Snowflake
Teradata’s architecture is based on a massively parallel processing (MPP) system that integrates storage and compute nodes in a single system, meaning that Teradata distributes data and queries across multiple nodes, each with its own processor, memory, and disk, to achieve high performance, throughput, and reliability.
Teradata can handle massive data volumes, support complex workloads, and provide enterprise-grade performance at scale.
Let's do a deep dive into the various components that make up Teradata's robust MPP architecture.
The architecture of Teradata consists of five main components: Teradata Nodes, Parsing Engine BYNET, and AMP. Here is the high-level architecture of the Teradata Node.
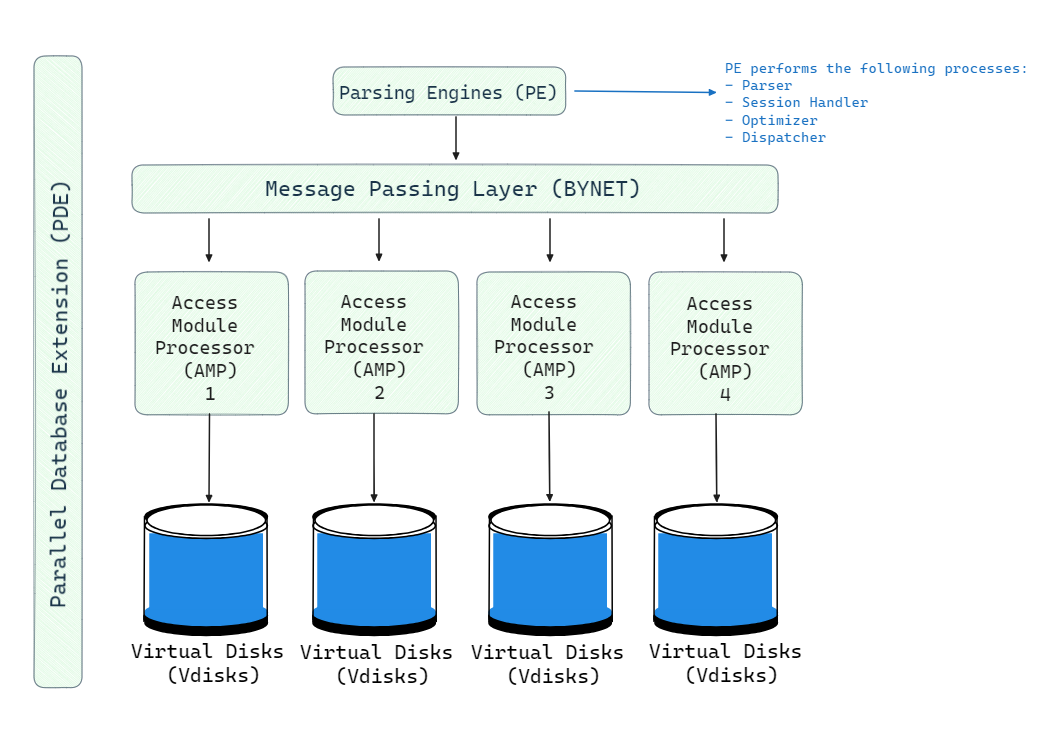
1) Teradata Nodes
The basic building block of a Teradata system is a node. Each node is an individual server consisting of the following elements:
- CPU: The central processing unit provides the computing capability. Each node will have multiple powerful CPUs.
- Memory: Substantial RAM is provisioned for in-memory operations, caching, and temporary storage.
- Storage: Disk storage is directly attached to each node for permanent storage of data.
- Network: An interconnect facilitates high-speed communication between nodes.
- Software: The Teradata database software and operating system is deployed on each node.
Multiple homogeneous nodes are interconnected to form a Teradata system. The number of nodes can scale into the hundreds to provide massive combined computing power and storage capacity.
2) Parsing Engine (PE)
Parsing Engine (PE) also known as a virtual processor (vproc), is a component in a Teradata system that handles communication with client applications and coordination of SQL processing. Each node has a Parsing Engine. The key responsibilities of the Parsing Engine are:
- Receive queries from client applications
- Parse and validate the SQL syntax
- Check user privileges and object access
- Generate an optimized execution plan
- Pass the execution plan to the BYNET for distributed execution
- Receive result rows and return to the client
The Parsing Engine processes the SQL and creates a plan for efficient execution by extracting parallelism in operations like scanning, filtering, aggregations, and joins.
TL;DR:
Parsing Engine acts as a communicator between the client system and AMP via BYNET.
3) BYNET (Message Passing)
BYNET is Teradata's messaging passing layer that connects the various components and facilitates intra-node communication. It routes messages between Parsing Engines and AMPs for query execution and result collection.
BYNET provides a high-speed, reliable, and efficient interconnect for passing requests, execution plans, data rows, and responses between nodes. It tracks the flow of messages across the system. BYNET allows massive parallelism by enabling multiple thousands of AMPs to coordinate.
4) Access Module Processors (AMPs)
Access Module Processors (AMPs) are the workhorses for data storage and processing in Teradata. There can be hundreds of AMPs across multiple nodes working together. AMPs perform the following key functions:
- Store row data across attached disk storage
- Receive execution plans from BYNET and extract operations
- Perform scans, filters, aggregations, sorting, joins and other data operations
- Send result rows back to BYNET for collection.
AMPs run in their own portion of memory and utilize available CPUs for data processing. BYNET distributes incoming queries to relevant AMPs holding the target tables and data involved. Each AMP has dedicated access to its attached storage for read and write operations.
5) Virtual Disks (Vdisks)
Vdisks are units of storage space owned by an AMP. Virtual Disks are used to hold user data (rows within tables). Virtual Disks map to physical space on a disk.
6) Parallel Database Extension (PDE)
Parallel Database Extension (PDE) provides APIs for various OS to interact with Teradata Node. The operating system (OS) can be MP-RAS, Linux, or Microsoft Windows operating systems.
Teradata Storage Architecture
Teradata uses a shared-nothing storage architecture where the entire data is evenly divided into chunks and distributed across all the AMPs. When new data is added through INSERT statements, the Parsing Engine passes the rows to BYNET which allocates them evenly to AMPs.
A hashing algorithm assigns rows to AMPs to ensure uniform distribution. Each AMP stores its rows on its locally attached disks as data blocks. This architecture eliminates storage bottlenecks since large datasets can be divided and stored in parallel across hundreds of AMPs and disks.
During retrieval, BYNET routes incoming queries to the relevant AMPs that contain the target tables. The AMPs scan and process the data blocks in parallel and pipe back qualifying rows through BYNET to the Parsing Engine.
TL;DR:
- Client application issues SQL INSERT statement to load data into a Teradata table
- Parsing Engine parses the statement, creates plan and sends insert request to BYNET
- BYNET routes the incoming rows to appropriate AMPs based on hash of primary index value
- Each AMP inserts its portion of rows into its managed physical storage
- AMPs acknowledge success back to Parsing Engine after commits
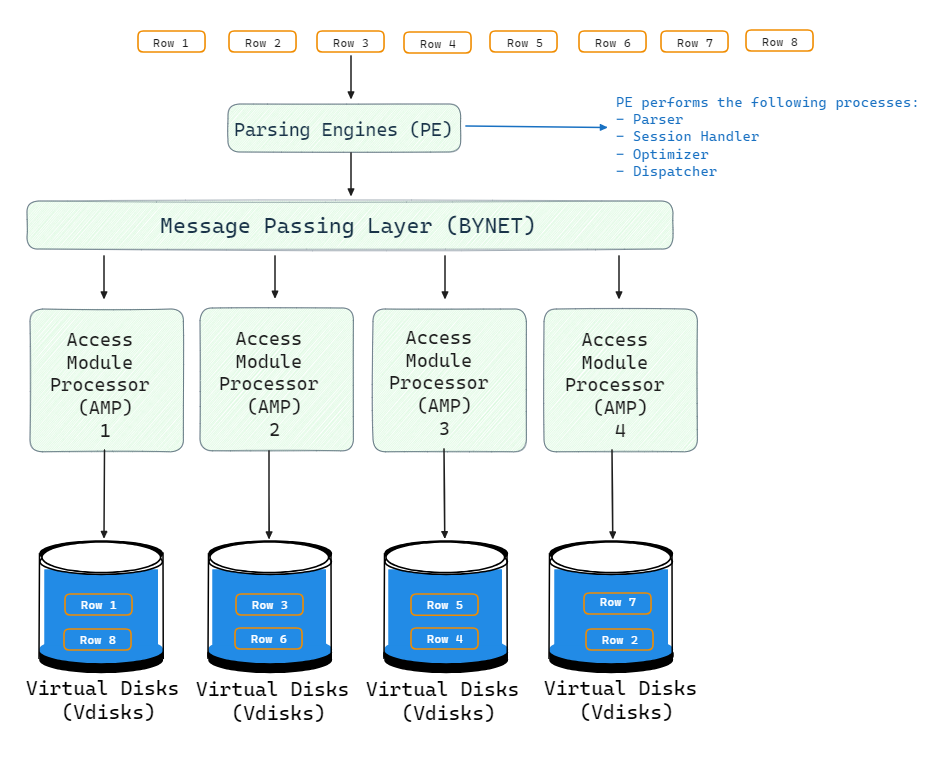
Teradata Query Processing Architecture (Retrieval Architecture)
During query processing, BYNET and AMPs retrieve data in parallel:
- Client sends a query request to Parsing Engine (PE).
- PE generates a plan and passes to BYNET.
- BYNET routes requests to relevant AMPs based on data location.
- AMPs scan their local data in parallel and return qualifying rows.
- BYNET transfers rows back to the PE.
- PE does any final aggregation and returns results.
All AMPs work concurrently on local data partitions. BYNET handles transferring rows between AMPs for additional processing like sorting, joining, aggregating, etc. The parallel architecture minimizes response times for even the most complex queries.
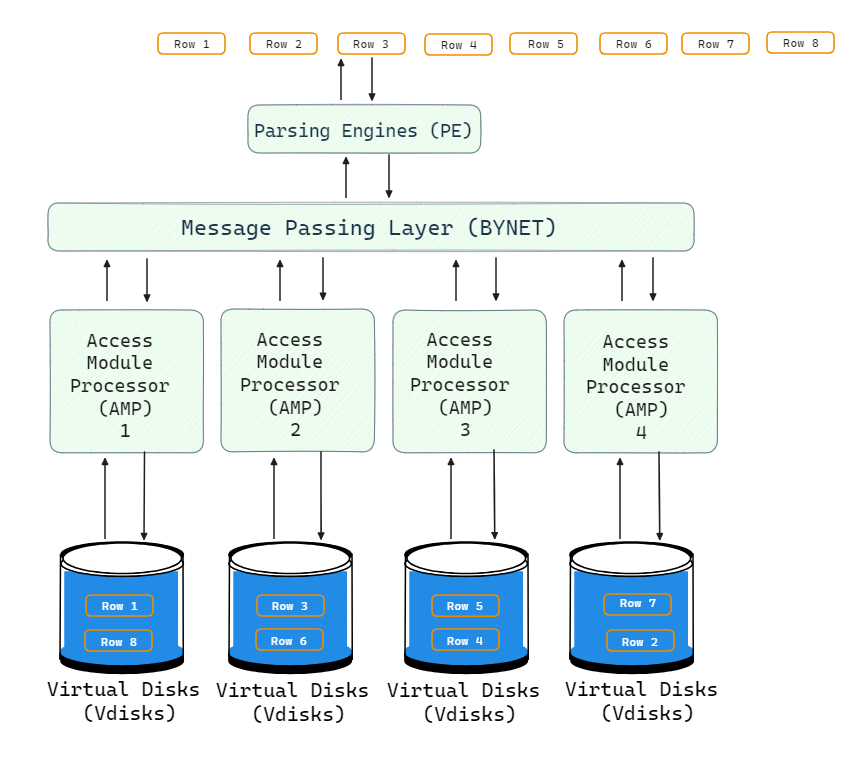
This powerful design can handle heavy workloads, massive data volumes, and concurrency levels that would overwhelm traditional databases.
2). Teradata vs Snowflake—Performance & Scalability
When it comes to sheer query performance, both Snowflake and Teradata can provide extremely fast response times. However, they achieve high performance through different architectural approaches.
Snowflake Performance & Scalability—Teradata vs Snowflake
One of the most revolutionary innovations from Snowflake is the total separation between storage and compute. Snowflake's storage layer runs on low-cost object storage from major cloud providers like AWS, Azure, and GCP. The storage is effectively infinite and decoupled from compute.
Snowflake then uses clusters of virtual warehouses to provide the compute power for running queries. These virtual warehouses can scale up and down instantly. More importantly, they scale independently from storage.
This architecture is the key reason why Snowflake offers such incredible query performance. Users can spin up as many virtual warehouses as needed for massive parallel query processing. Snowflake can literally throw hundreds of servers at a query to return results in seconds!
The scaling is also completely on-demand. Virtual warehouses can be spun up and shut down immediately. So you only pay for the compute you use. Snowflake is designed to leverage the cloud's virtually unlimited resources to deliver performance when you need it.
Teradata Performance & Scalability—Teradata vs Snowflake
Teradata, on the other hand, relies on a more traditional scale-up approach to achieve high performance. It runs on an MPP shared-nothing architecture with intelligent data distribution and parallel processing across multiple nodes.
Each Teradata node contains CPUs, memory, storage, and networking. Queries are decomposed into steps and distributed across nodes for parallel execution. Teradata can achieve excellent performance by scaling up the number of nodes and the specifications of each node.
But there are more upper limits in Teradata vs Snowflake's cloud-native architecture. The network interconnect bandwidth, storage capacity, and number of nodes define the performance boundaries.
That said, Teradata has 45 years of expertise in optimizing complex queries and workloads. It has mature query optimization, indexing, data compression, and workload management capabilities. For many existing EDW use cases, Teradata can match Snowflake's raw speed through sheer engineering prowess.
The Choice Depends on Your Workload.
So to sum it up, both Snowflake and Teradata are capable of top-notch query performance. Snowflake provides virtually unlimited scale-out capabilities, leveraging the cloud. Teradata relies on scale-up and deep optimization.
For newer cloud-based projects with unpredictable workloads, Snowflake is hard to beat. But existing complex EDWs may benefit by staying on Teradata while migrating to the cloud. The optimal choice depends heavily on each organization's specific technical environment, workload patterns, and cost considerations.
3). Teradata vs Snowflake—Workload Configuration & Management
For any large business/organization, the data warehouse needs to handle a diverse mix of workloads—from ad hoc analytics to user dashboards, ETL data loads, query tuning tasks and more. Both of ‘em Teradata vs Snowflake are mature enough to handle multiple concurrent workloads efficiently. However, their approaches reflect their different architectures.
Snowflake Workload Config & Management—Teradata vs Snowflake
Snowflake's architecture sets it apart when it comes to workload management. Its storage layer is centralized but completely separate from compute. The virtual warehouses provide all the query processing power.
Snowflake allows creating multiple virtual warehouses for each logical workload.
Each cluster can be scaled up and down independently based on its usage. New clusters can be spun up instantly to isolate new workloads. Snowflake provides seamless workload isolation and "infinite" processing power through its multi-cluster architecture.
Snowflake also offers Workload Management and monitoring tools. Admins can define workload management rules to control concurrency, queuing policies, and allotted credit limits for different user groups and workloads.
Teradata Workload Config & Management—Teradata vs Snowflake
Unlike Snowflake’s independent workload silos, Teradata uses a holistic workload management approach. All concurrent queries and operations go through a centralized, closed-loop workload manager.
Sophisticated algorithms schedule and prioritize queries to optimize overall throughput and service levels. The workload manager assembles queries into dispatch windows to maximize system resources like AMPs, BYNET, Vdisks, and CPUs.
Admins can define workload rules based on business SLAs. More important workloads get priority in resource allocation. Limits can be set for low-priority workloads to avoid impacting others. Teradata also offers tools like Data Fabric, QueryGrid and Viewpoint to monitor and tune workload performance.
The key difference between Teradata vs Snowflake is that Teradata actively optimizes and balances all workloads globally across the system. There is tighter coordination between workloads to maximize system utilization. It provides more "fine-tuned" management versus brute-force isolation and scaling.
4). Teradata vs Snowflake—Ecosystem & Integration
Teradata vs Snowflake: both of ‘em have extensive partnerships and integrations available.
Snowflake Ecosystem and Integration—Teradata vs Snowflake
Snowflake supports a wide range of integrations with technology partners and cloud services. It enables seamless connectivity to leading business intelligence tools like Tableau, Looker and Power BI for data visualization and reporting.
Snowflake also supports data ingestion and analysis from various SaaS applications through first-party and third-party connectors. Moreover, Snowflake integrates natively with major cloud platforms—AWS, Azure, and GCP—allowing organizations to use their preferred cloud infrastructure and services.
For custom integrations, Snowflake offers a REST API that can be used to interact with the data platform programmatically and build connections with different applications as per business requirements.
To enhance its analytics capabilities, Snowflake has partnered with top data management and governance solutions. For example, it has partnered with Collibra for data cataloging and metadata management, Talend for ETL and data integration, and Alteryx for data blending and preparation.
The Snowflake Marketplace offers various partner applications, connectors, and accelerators that extend Snowflake's core functionalities.
Teradata Ecosystem and Integration—Teradata vs Snowflake
Teradata has been in the data warehousing business much longer than Snowflake and thus naturally has a more mature and larger ecosystem of partners that have supported users for decades. Teradata has over ~110+ ecosystems of partners that offer various integrations and solutions for users. Some notable categories of Teradata partners are:
Technology Alliance Partners
Like Snowflake, Teradata partners with AWS, Azure, and GCP to offer users hybrid deployment flexibility. It has additional OEM partnerships with companies like IBM and more.
Consulting Partners
Large system integrators and Teradata services partners help users with the the implementation, support and managed cloud services on Teradata's platform. Major partners include Accenture, Adastra, s.r.o., Algo, Cognizant, Capgemini etc.
Startups
Teradata actively seeks partnerships with innovative startups. Startups get access to Teradata expertise and users.
Independent Software Vendors
Teradata works with hundreds of ISV partners across marketing, IoT, healthcare, security and other domains. Key partners include Alation, ActionIQ, Alteryx, Astera Data—and so much more.
Academia Partners
Teradata Academic Alliance promotes research and innovation through university partnerships globally. It provides research grants and data platforms to educational institutions.
Check out the list of Teradata ecosystem partners.
Like Snowflake's partner ecosystem, Teradata's partners deliver consulting, technology and domain-focused solutions to help users maximize ROI from analytics. But key differences are that Teradata maintains tightly controlled OEM relationships with hardware vendors and a more proprietary approach compared to Snowflake's open model.
5). Teradata vs Snowflake—Security & Governance
Security and governance are essential aspects of any data platform, as they ensure the protection, quality, and compliance of the data stored and accessed by various users and applications. Both Teradata and Snowflake provide robust features and capabilities to support data security and governance, but there are some differences in their approaches and offerings.
Snowflake Security & Governance—Teradata vs Snowflake
Snowflake provides robust security capabilities to safeguard data and meet compliance requirements. Snowflake utilizes a multi-layered security architecture consisting of network security, access control, and End-to-End encryption.
Network security
Snowflake allows configuring network policies to restrict access to only authorized IP addresses or virtual private cloud (VPC) endpoints. Users can set up private connectivity options like AWS PrivateLink or Azure Private Link to establish private channels between Snowflake and other cloud resources.
Access Control
Snowflake has extensive access control mechanisms built on roles and privileges. Users can create roles aligned to specific job functions and assign privileges like ownership or read-write access accordingly. Granular access control is also possible through Object Access Control, Row Access Control via Secure Views and Column Access Control by masking columns. Multi-factor authentication and federated authentication via OAuth provide additional access security.
Encryption
Encryption is a core part of Snowflake's security posture. All data stored in Snowflake is encrypted at rest using AES-256 encryption by default. Snowflake supports both platform-managed and user-managed encryption keys. For key management, Snowflake provides built-in key rotation and re-keying capabilities. Users can also enable client-side and column-level encryption for enhanced data protection.
Snowflake Governance
Snowflake offers robust governance capabilities through features like column-level security, row-level access policies, object tagging, tag-based masking, data classification, object dependencies, and access history. These built-in controls help secure sensitive data, track usage, simplify compliance, and provide visibility into user activities.
Check out this article to learn more in-depth about implementing strong data governance with Snowflake.
Teradata Security & Governance—Teradata vs Snowflake
Over its 45+ year history, Teradata has built an extensive arsenal of enterprise-grade security and governance practices to protect data and provide visibility into its usage.
Network and Encryption Security
Teradata secures communications via encryption and firewalls. Database traffic can be encrypted with AES, 3DES, or RC4 symmetric algorithms. SSL/TLS connections prevent man-in-the-middle attacks.
To prevent network eavesdropping, Teradata can encrypt specific requests or entire sessions. Data at rest can also be encrypted using solutions like Protegrity Database Protector. Backup archives can be encrypted using tape drive or deduplication system encryption.
Teradata's BYNET provides the secure interchange between different Terabyte nodes. BYNET handles authentication and access controls when transferring requests and data between nodes.
User Authentication
You can't protect your data without knowing who is accessing it. Teradata offers authentication methods to validate user identities:
- LDAP integration allows central user authentication against Active Directory or other LDAP directories.
- Kerberos support enables single sign-on so users don't reauthenticate to Teradata after network login.
- Teradata can integrate with identity providers like SAML and OAuth for SSO across cloud applications.
- Two-factor authentication/MFA adds an extra layer of identity verification for high-security environments.
Once users are authenticated, their access is restricted only to data they are authorized to see.
Authorization Controls
Teradata's access controls allow granular permissions so users only access data necessary for their role. For example:
- Multi-level security roles simplify assigning access privileges across groups of users. Roles can be nested for efficient administration.
- Row-level and column-level access controls restrict data visibility at very granular levels.
- Object privileges precisely control capabilities like SELECT or DELETE on specific database tables and views.
- Context-based rules can dynamically filter data based on session user, application, time, geo-location and other factors.
Leveraging these capabilities enables the enforcement of least privilege and separation of duties policies.
Auditing and Monitoring
To identify suspicious activity, Teradata provides extensive monitoring and auditing capabilities:
- Database activity logging records security events like logins, logouts, user actions, privilege use, account changes, and more.
- Tools like Data fabric and QueryGrid give admins visibility into all query activity.
- Triggers can perform custom logging based on data access or changes.
- Periodic audits validate that access controls, passwords, and log settings adhere to security policies.
These capabilities enable security teams to conduct forensic analysis in case of suspected breaches or policy violations.
Compliance Support
Regulations like GDPR and HIPAA impose strict security and privacy standards that Teradata can help address:
- Data discovery, masking, and right-to-be-forgotten features assist with GDPR compliance.
- Granular access controls, auditing help satisfy HIPAA data security mandates.
- Certifications like ISO 27001 and Common Criteria validate Teradata's security posture.
Teradata also provides documentation, including security white papers and deployment guides, focusing on compliance needs.
Partners in Security: Ecosystem Integrations
Teradata integrates with leading security solutions to enable end-to-end protection:
- SIEM integration sends Teradata alerts to security analytics platforms.
- Tools like Imperva, Protegrity and Thales provide data encryption, masking, and tokenization.
- Micro Focus solutions enable access controls, user provisioning and privileged access management.
These partnerships expand Teradata's security capabilities across the extended technology stack.
Check out all the list of Teradata security ecosystem partners
Teradata offers guidance and best practices on the organizational aspects of data protection. Check out this teradata white paper for an in-depth understanding of Teradata's security and governance features.
6). Teradata vs Snowflake—Supported Programming Languages
Whenever you are working with large-scale data platforms, it is important for data professionals to leverage familiar languages for various automation and analytics tasks. Both Snowflake and Teradata understand this need and support a wide variety of programming languages out of the box. Now, in this section, we will explore the different languages supported by each of these platforms.
Snowflake Programming Language Support—Teradata vs Snowflake
Snowflake supports developing applications using many popular programming languages and development platforms. Using native clients (connectors, drivers, etc.) provided by Snowflake, you can develop applications using any of the following programmatic interfaces:
- ANSI SQL: Snowflake is built for SQL-first data processing and is fully ANSI SQL compliant. You can write complex queries, CTAS statements, temporal queries, array processing and more using standard SQL. You can also use ANSI SQL to interact with Snowflake from various tools and applications, such as SnowSQL (the Snowflake command-line client), Snowflake web interface, and third-party SQL clients.
- User-Defined Functions (UDFs): Snowflake allows you to create and execute user-defined functions (UDFs) that extend the functionality of Snowflake and perform custom logic on your data. You can create UDFs using the following languages:
- JavaScript
- Python
- Java
- Scala
- SQL UDFs
- REST API: Snowflake also exposes a robust REST API allowing HTTP requests for all warehouse operations from any language via simple GET/POST calls.
Teradata Programming Language Support—Teradata vs Snowflake
Unlike Snowflake's more open-ended approach, Teradata takes a pragmatic path:
- Teradata primarily relies on widely used languages like C/C++, Java, Python, Go, and R for stored procedures and UDFs.
- It also supports traditional languages like COBOL, Fortran, Perl, and Ruby, used heavily in enterprises.
- SQL support is ANSI compliant. SQL stored procedures can contain control flow logic.
- JSON and XML data types are also natively supported in Teradata.
- Connectors are also supported which allow accessing Teradata data from outside tools like R, Matlab, SAS, TensorFlow, and PyTorch.
No matter your language preference, both Teradata and Snowflake have you covered.
7). Teradata vs Snowflake—Indexing
Indexes are a fundamental mechanism for optimizing database performance. By creating indexes on one or more columns of a table, queries that filter or sort by those columns can be executed much faster. This is because indexes allow the database to quickly locate and retrieve only the relevant data without having to scan the entire table.
Different db/warehouse technologies take varying approaches to indexing. Let's examine how Teradata and Snowflake each tackle this important performance tuning aspect.
Snowflake Indexing—Teradata vs Snowflake
Snowflake has taken an unconventional stance by choosing not to support traditional indexes. In short, Snowflake does not use indexes to optimize queries. Instead, it leverages techniques/features like micro-partitioning, clustering keys, automatic clustering, and query pruning to deliver fast query performance.
Snowflake stores data in small micro-partitions, typically 50 MB to 500MB in size. Micro-partitions contain a subset of rows stored in a columnar format, which enables parallel processing and scanning only relevant data during queries.
Snowflake utilizes sophisticated query pruning techniques. Using stats on micro-partitions and query predicates, Snowflake determines which micro-partitions can be eliminated from scanning based on the query filter conditions. This minimizes the amount of data required for processing.
For further optimization, Snowflake can automatically cluster data by grouping related rows into the same micro-partitions. A clustering key defines the column(s) to cluster data on. Clustering improves pruning efficiency as related rows exist in fewer partitions.
On top of all this, Snowflake continuously analyzes comprehensive query history and table statistics to adapt and optimize query execution plans over time. It determines the optimal approaches to process different query types and data shapes without manual tuning.
When you combine micro-partitions, intelligent optimization, clustering, and scale-out compute, Snowflake doesn't need traditional database indexes for most workloads!
Teradata Indexing—Teradata vs Snowflake
Given its longer history in data warehousing, Teradata uses a full-fledged indexing system to maximize performance. The main types of indexes it supports are:
1) Primary Indexes: A primary index is a unique, non-null column like a primary key that is used to uniquely identify each row in a table. Teradata uses primary indexes for efficient distribution, retrieval, and locking of data. Each table in Teradata must have at least one column as Primary Index. Primary index cannot be altered or modified.
There are two types of Primary Indexes:
- Unique Primary Index (UPI).
- Non-Unique Primary Index(NUPI)
2) Secondary Indexes: Secondary indexes allow non-unique queries against columns other than the primary key. They improve the access performance of queries using indexed columns. Secondary Index can be created or altered after the creation of the table. There are two types of Secondary Index:
- Unique Secondary Index (USI).
- Non-Unique Secondary Index (NUSI).
3) Join Indexes: These optimize access patterns for common join queries. They store pointers to rows matching join criteria rather than index column values. Note that defining the join index in Teradata does not imply that the parsing engine will always use the join index. It is up to Teradata parsing engine whether to use join index or access from the base table.
There are different types of join index in Teradata:
- Single Table Join Index(STJI)
- Multi Table Join Index(MTJI)
- Aggregate Join Index
- Sparse Join Index
4) Hash Indexes: Hash indexes distribute rows across partitions according to a hashing algorithm for very fast retrieval of exactly matching values. Hash index has been designed to improve the performance of Teradata query in a way similar to the Single Table Join Index, which restricts PE not to access or redistribute the base table.
Bonus: Teradata vs Snowflake—Billing & Pricing Models
Finally, Teradata and Snowflake distinctly vary in their pricing models. In this part, we will dive into the depth of "Teradata vs Snowflake" pricing to help you in making well-informed and cost-effective decisions for your data platform investment.
Snowflake pricing Breakdown—Teradata vs Snowflake
Snowflake uses a pay-per-usage pricing model based on actual usage, rather than fixed hourly or monthly fees. Users are charged by the second for the processing power used based on the size of the virtual warehouses (VW) deployed. Snowflake separates storage charges from compute. Storage is charged based on average monthly storage usage after compression.
Note: Snowflake does not charge data ingress fees to bring data into your account, but does charge for data egress
When it comes to pricing, Snowflake keeps things simple yet flexible. The cost is based on your usage of three key components:
1) Storage Costs
Let's break it down one piece at a time. First up is storage. This refers to how much data you have stored in Snowflake. Your monthly storage cost depends on the average amount of compressed data you use each month. Snowflake compresses your data, so you may put in 1TB but it takes up less space after compression—and you only pay for what's actually stored.
You've got two options for storage pricing.
- On-Demand: With the help of On-Demand, you pay a per GB rate each month based on usage. Rates vary by cloud platform and region. For example, On-Demand storage on AWS in the US East (Northern Virginia) is ~$40 per, whereas it's $45 per TB per month for those deployed in the EU region. Simple! You can calculate your cost yourself from here.
- Capacity Storage: The other option is Capacity pricing. Here you pre-pay for a chunk of storage upfront. This gets you better rates and a long-term price guarantee. The cost depends on your total commitment amount. Once your prepaid capacity runs out, you flip to On-Demand rates. Capacity pricing runs around $23-25 per TB in the US and EU.
2) Compute Costs
Next up is compute power for querying data, also known as “virtual warehouses”. You can choose from sizes like:
- X-Small (extra small)
- Small
- Medium
- Large
- X-Large (extra large)
- 2X-Large (2 times extra large)
- 3X-Large (3 times extra large)
- 4X-Large(4 times extra large)
- 5X-Large(5 times extra large)
- 6X-Large(6 times extra large)
Bigger warehouses pack more punch for complex queries. The credits required for compute scale-up as the warehouse size increases. An X-Small warehouse costs 1 credit per hour, while a 4X-Large is 128 credits per hour.
Smaller warehouses are far cheaper for basic queries. But bigger ones accelerate performance for tougher jobs, though not always proportionally. Scaling may hit diminishing returns. Workload tests help pick the optimal size.
The cool part is that compute billing is per second. When not querying, warehouses auto-stop, avoiding unused idle time charges. They restart instantly when you resume work. This on-demand model saves big versus always-on resources.
Here is the complete breakdown of credits used per warehouse size:
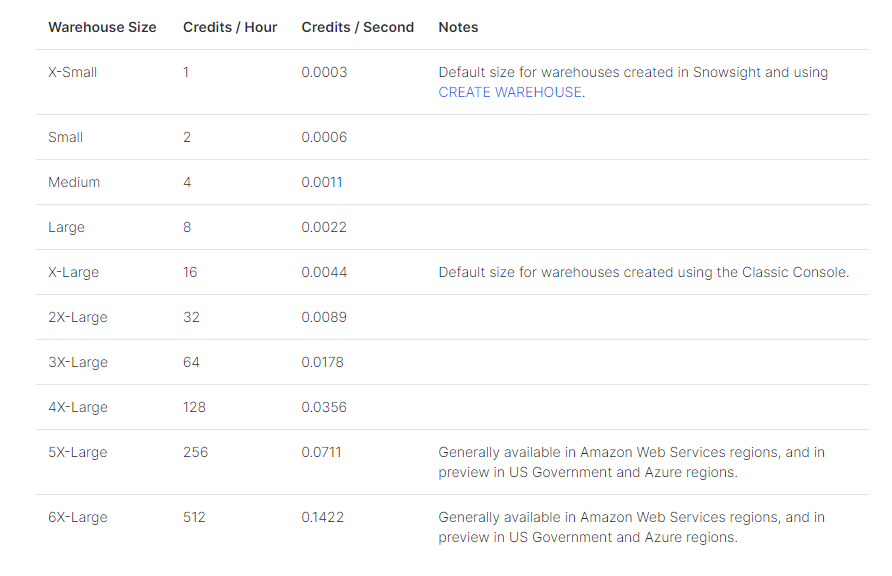
3) Cloud Services
Last but not least are cloud services. Cloud service layers offer multiple services like authentication, authorization, query calculation, SQL API, performance tuning, analytics security features—and much more. Because these features are provided by default, Snowflake does not charge users directly for them. But it will charge based on the specific condition. Snowflake automatically assigns cloud services based on the requirements of the workload. Typical utilization of cloud services, up to 10% of daily compute credits, is included for free, meaning most users and users won't see incremental charges for cloud service usage. Assume you have a virtual warehouse and have spent “A” credits. Consider cloud service layer credit to be “B” credit; if it is within 10% of the “A”, it will not be charged to the user; if it exceeds 10% of the daily computing credit, it will be charged to the user; otherwise, it will be free to use.
Learn more in-depth about Snowflake pricing.
4) Data Transfer
Last but not least is data transfer. Snowflake calculates data transfer costs by considering criteria such as data size, transfer rate—and the region or cloud provider from where the data is being transferred.
Snowflake data transfer cost structure depends on two factors: ingress and egress.
Ingress, means transferring the data into Snowflake. It is free of charge. Data egress, however, or sending the data from Snowflake to another region or cloud platform incurs a certain fee. If you transfer your data within the same region, it's usually free. When transferring externally via external tables, functions, or Data Lake Exports, though, there are per-byte charges associated that can vary among different cloud providers.
Take a look at some Snowflake pricing tables for comparison to get an idea of what the difference in cost could look like:
- Snowflake Data Transfer Costs in AWS
- Snowflake Data Transfer Costs in Microsoft Azure
- Snowflake Data Transfer Costs in GCP
Snowflake Pricing Plan Editions
Snowflake also offers four editions with different features and pricing:
1) Standard Plan
2) Enterprise Plan
3) Business Critical Plan
4) Virtual Private Snowflake
Check out the in-depth Snowflake Service Consumption Table
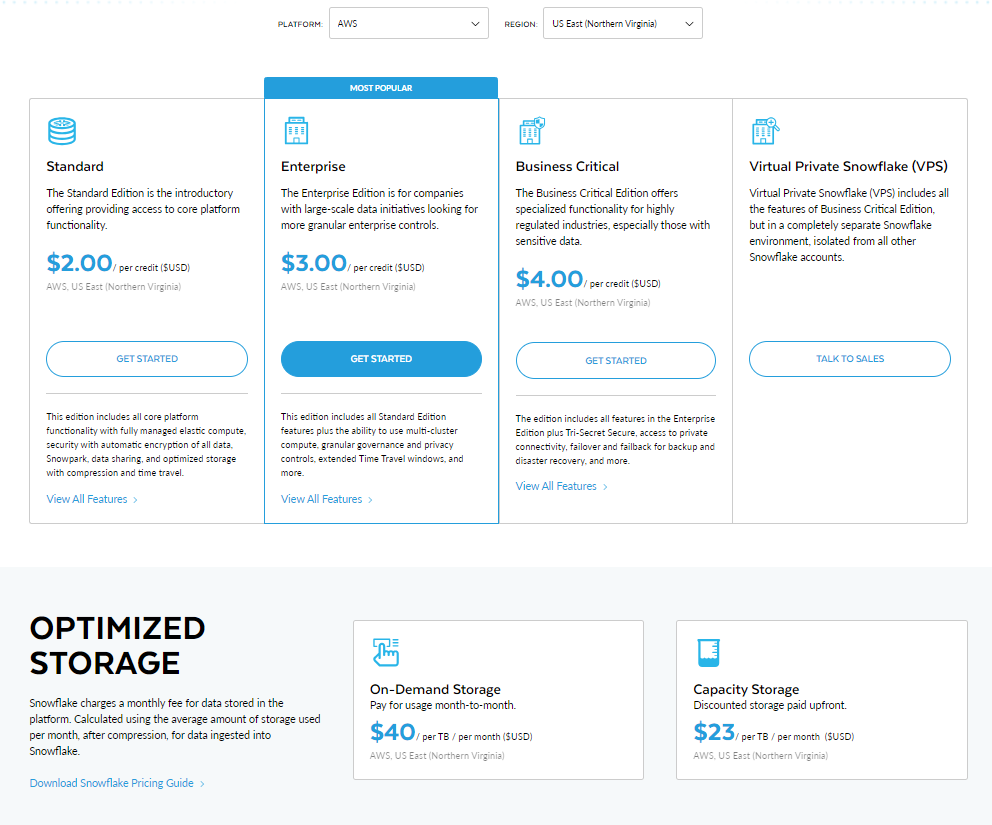
Teradata Pricing Breakdown—Teradata vs Snowflake
Teradata offers various pricing models for its products and services, depending on the user’s needs, budget, and goals. In this part, we will break down the pricing structure of Teradata Cloud(Vantage).
Teradata’s pricing is based on units, which are a measure of the resources consumed by the user’s workloads. Each unit corresponds to a certain amount of compute, storage, and data transfer capacity. The price per unit varies depending on the deployment option, the cloud service provider, the region, and the commitment term. Teradata offers both on-demand and commitment pricing, as well as consumption-based pricing for users who want more flexibility and control over their usage and spend.
Teradata’s main product is Vantage, a cloud-native platform that enables users to access, manage, and analyze all their data, regardless of the source, format, or location. Vantage can be deployed on any of the major cloud service providers, such as AWS, Azure, or Google Cloud, or on Teradata’s own managed cloud service. Users can choose from different packages that include different features and services, such as:
VantageCloud Lake is designed for users who want to leverage the power and scalability of object storage for their data lake workloads. VantageCloud Lake offers a low-cost entry point, starting from ~$4,800 per month, with a one-year commitment. Users can control when their compute resources are running, allowing for flexibility in cost management. Storage and data transfer are charged separately, based on the actual usage.
VantageCloud Lake includes:
- Premier Cloud Support
- ClearScape Analytics
- Ecosystem tools
Users can also opt for VantageCloud Lake+, starting around ~$5,700/month, which adds Priority Service and industry data models.
VantageCloud Enterprise is designed for users who want to run their enterprise-grade analytics workloads on a high-performance, secure, and reliable platform. VantageCloud Enterprise offers a higher price per unit, starting from ~$9,000 per month, with a one-year commitment. Users can scale their compute and storage resources independently, based on their needs. Storage and data transfer are included in the price per unit, up to a certain limit.
VantageCloud Enterprise includes:
- Premier Cloud Support
- ClearScape Analytics
- Ecosystem tools
- Advanced Services
Users can also opt for VantageCloud Enterprise+, starting around ~$10,500/month, which adds Priority Service, industry data models, and Direct Quick Start Support.
Teradata also offers consumption pricing, which is a pay-as-you-go model that charges users based on the units they consume, or a low monthly minimum. Consumption pricing is ideal for users who have unpredictable or variable workloads or who want to try out Teradata’s products and services without a long-term commitment. Consumption pricing is available for both VantageCloud Lake and VantageCloud Enterprise packages and includes the same features and services as commitment pricing.
Teradata’s pricing is competitive and transparent, and offers users the best price performance at scale. Users can even use Teradata’s pricing calculator to estimate their cost for Teradata in the cloud, based on their answers to five simple questions. Users can also directly contact to a Teradata expert to get a personalized quote and guidance on the best pricing option for their business needs and goals.
Teradata vs Snowflake—Pros and Cons:
Choosing the right platform is extremely crucial if you are looking to manage and analyze their data effectively. In this section, we will explore the pros and cons of Teradata vs Snowflake to help you make the right decision.
Snowflake pros and cons: Why migrate from Teradata to Snowflake?
Here are the main Snowflake pros and cons:
Snowflake pros |
Snowflake cons |
High Performance — Snowflake is renowned for its exceptional performance. Its unique architecture separates compute and storage, allowing for elastic scalability and parallel processing. |
Complexity of Usage & Learning curve — Snowflake's advanced features and capabilities may require a learning curve for users who are new to the platform. While Snowflake provides in-depth documentation and resources to help users, understanding and fully utilizing all the features may take time and effort. |
Instant Disruption-Free Scalability — Snowflake's cloud-native architecture makes it incredibly easy to scale resources up or down based on demand. With just a few clicks, you can instantly provision additional compute resources to handle peak workloads without any kind of disruption. |
Limited Support — Snowflake's support offerings are primarily focused on its cloud-based platform. If you require on-premise support or have specific customizations, you may face limitations in terms of available support options. |
Seamless Integration with Cloud Providers and Data Sources — Snowflake offers seamless integration with major cloud providers like Amazon Web Services (AWS), Google Cloud Platform (GCP), Microsoft Azure (Azure). This integration allows user/business/organizations to leverage their existing cloud infra and easily integrate with various data sources. |
Dependency on Cloud Infrastructure and Vendor Lock-In — Snowflake is a cloud-native platform, which means it relies on cloud infrastructure provided by AWS, Azure, or GCP. While this offers scalability and flexibility, it also means that organizations are tied to their chosen cloud provider. Switching cloud providers or moving back to an on-premise environment may involve significant effort and potential vendor lock-in. |
Best in class Security, Governance, and Compliance — Snowflake provides robust security features to protect your data. Security is also a top priority for Snowflake. It offers robust security capabilities like role-based access control, network policies, end-to-end encryption—and more. Also, Snowflake is compliant with industry standards and regulations like GDPR, HIPAA, and PCI DSS, which helps to make sure that your data remains secure and meets regulatory requirements. |
|
Advanced AI and ML Support — Snowflake's platform integrates well with advanced analytics, artificial intelligence (AI), and machine learning (ML) tools, enabling engineers, data scientists and analysts to leverage the power of AI and ML algorithms on Snowflake's scalable infrastructure, facilitating advanced data analytics and predictive modeling. |
|
Cost — Snowflake's pricing model is based on actual usage, allowing users to pay only for the resources consumed. This cost-effective approach eliminates the need for upfront hardware investments and provides flexibility in managing data warehousing costs. |
Teradata pros and cons: Why migrate from Snowflake to Teradata?
Now, let’s review some of the key considerations for and against migrating from Snowflake back to Teradata:
Teradata pros |
Teradata cons |
Mature and Proven Platform — Teradata has been operating as an on-premise data warehousing leader for over 4 decades, with a proven track record of supporting some of the largest and most complex analytics environments in the world. The platform is highly stable, optimized, and refined based on decades of production usage. |
High Cost of Ownership — Teradata traditionally required very large upfront license fees for the database software and costly proprietary hardware capable of its parallel processing architecture. Total costs of maintaining an on-prem system can easily be double or triple that of cloud alternatives over 5 years. |
Advanced Security — Teradata was built from the ground up with an obsessive focus on security. Advanced/Military-grade encryption, advanced authentication, auditing, and immutable data integrity checks help ensure the highest standard of data protection for the most sensitive operations. |
Limited Scalability — While efficient in its optimized configuration, on-prem Teradata appliances have finite resource ceilings. Vertical scaling through hardware upgrades is complex and eventually hits physical limits. The elastic scaling afforded by the cloud is impossible to achieve on-premises in the same seamless, on-demand manner. |
Advanced Analytics Capabilities — Teradata provides an extensive analytics toolkit enabling users to leverage the full power of their databases for advanced scenarios like predictive modeling, multivariate testing, text/spatial analysis—and more. |
Steep Learning Curve — The depth and complexity of Teradata’s feature set means a significant ramp-up and learning time is required for users to become truly proficient. |
Strong Community Support — Teradata has a massive community of users and developers who actively contribute to knowledge sharing and problem-solving. This community support can be valuable when seeking assistance or exploring best practices. |
|
DS/AI/ML Capabilities — Teradata provides integrated support for data science, AI, and ML workflows. It offers a plethora of tools and libraries for data preparation, model development, and model deployment. |
|
Extensive Language Support — From SQL to R, Python and Java/C++, users have the flexibility to develop in their preferred languages and call any analytical routines or APIs directly against the database for streamlined end-to-end processes. |
|
On-Prem Operational Control — Teradata offers on-premise deployment options, providing organizations with greater control over their data infrastructure. This level of control can be very beneficial for companies with specific data governance, compliance, or regulatory requirements. |
Conclusion
And that’s a wrap! Teradata vs Snowflake: both offer compelling options for enterprise cloud data warehousing. Snowflake is setting the pace with its innovative architecture delivering unmatched scalability and ease of use. However, Teradata still has advantages for the most demanding use cases thanks to its proven performance, security and governance capabilities built over decades.
In this article, we covered:
- What is Teradata?
- What is Snowflake?
- Teradata vs Snowflake: 7 Critical Features You Need to Know
- Teradata vs Snowflake—Architecture Breakdown
- Teradata vs Snowflake—Performance & Scalability
- Teradata vs Snowflake—Workload Configuration & Management
- Teradata vs Snowflake—Ecosystem & Integration
- Teradata vs Snowflake—Security & Governance
- Teradata vs Snowflake—Supported Programming Languages
- Teradata vs Snowflake—Indexing
- Bonus: Teradata vs Snowflake—Pricing Model
- Snowflake Pros and Cons: Why migrate from Teradata to Snowflake?
- Teradata pros and cons: Why migrate from Snowflake to Teradata?
—and so much more!
If you are considering modernizing your analytics stack in the cloud, you cannot go wrong with either option. However, you must carefully evaluate your existing infrastructure, workloads, costs, and use cases.
The data warehouse market will likely continue to see stiff competition between Snowflake having its dominance VS Teradata reinventing itself for the cloud world. There are also BIG challengers like Databricks, BigQuery, and Redshift pressing on both sides. But, both Snowflake and Teradata are here to stay as leading enterprise-grade solutions for the foreseeable future.
FAQs
What is Teradata?
Teradata is an enterprise data warehousing solution that provides high-performance analytics and data management on a massively parallel processing (MPP) system. It has been a leader in data warehousing for over 45 years (4 decades).
What is Snowflake?
Snowflake is a cloud-native data warehouse platform that runs on public cloud infrastructure. It uses a unique architecture that separates storage and compute to provide flexibility, scalability, and performance.
How do Teradata vs Snowflake architectures differ?
Teradata uses an MPP architecture that integrates storage and compute in a shared-nothing system. Snowflake has a hybrid architecture separating storage and compute layers.
Which solution scales better - Teradata vs Snowflake?
Snowflake can scale compute and storage independently leveraging the elasticity of the cloud. Teradata relies on scaling up nodes but has more finite limits.
How do Teradata and Snowflake handle diverse workloads?
Snowflake isolates workloads into separate virtual warehouses. Teradata uses centralized workload management to optimize mixed workloads globally.
Does Teradata offer cloud deployment options?
Yes, Teradata provides managed services called Vantage for deploying its solutions on public clouds like AWS, Azure, and GCP. It also enables hybrid cloud architectures.
Which solution has better security capabilities - teradata vs Snowflake?
Both Snowflake and Teradata provide robust security and encryption capabilities. However, Teradata offers more advanced features due to its enterprise background.
What languages can be used with Snowflake and Teradata?
Snowflake supports SQL, JavaScript, Python, Scala, and Java. Teradata supports SQL, C/C++, Java, Python, Go, R, and traditional languages like COBOL.
How does query performance compare between the two platforms?
Both of ‘em can deliver excellent query performance for different reasons. Snowflake leverages scale-out power while Teradata relies on optimizations and workload management.
What compliance standards do Snowflake and Teradata support?
Snowflake supports SOC, HIPAA, and PCI DSS compliance. Teradata supports various standards including HIPAA, PCI DSS, FedRAMP, and more.
How does indexing work in Teradata vs Snowflake?
Snowflake uses micro-partitioning and clustering instead of indexes. Teradata offers advanced indexing capabilities like primary, secondary, join, and hash indexes.
What pricing models do Snowflake and Teradata offer?
Snowflake uses pay-per-usage billing. Teradata offers capacity-based and consumption pricing models with discounted tiers.
Does Snowflake require upfront costs or commitments?
No. Snowflake uses a purely usage-based model with no upfront fees or capacity commitments required.
Can Teradata be deployed on-premises?
Yes, Teradata continues to support on-premises appliances and software deployments in customer data centers.
Which solution has a larger ecosystem of partners and integrations - Teradata vs Snowflake?
Teradata has a more expansive ecosystem due to its long history in enterprise environments. But Snowflake is rapidly growing its partner network.
Is Snowflake completely serverless?
No, Snowflake still utilizes compute clusters. But its architecture automates provisioning and management of those resources.
Can Teradata query object storage like S3?
Yes, Teradata can query data in S3, Azure Blob and other cloud object stores.
Does Snowflake allow unlimited storage and compute?
Snowflake storage and compute can scale to very large amounts but still have some limits based on configuration.
When is Teradata a better choice than Snowflake - Teradata vs Snowflake?
For existing complex EDWs with large legacy investments, specialized needs like high security, and advanced analytics use cases.
When is Snowflake a better choice than Teradata - Teradata vs Snowflake?
For new cloud initiatives, variable/unpredictable workloads needing elasticity, and organizations without legacy infrastructure constraints.