Snowflake is one of the top cloud data warehousing platforms out there. It gives you a managed service to store, process, and analyze massive datasets. What really sets it apart is its unique hybrid structure, which blends shared-disk and shared-nothing architecture. This makes scaling easy and allows it to handle high-performance data with ease. Snowflake was founded in July 2012 by Benoit Dageville, Thierry Cruanes, and Marcin Zukowski. Their mission was to tackle a big data problem: making cloud data storage and computing easy to use and scalable, so users wouldn't get bogged down by traditional warehouse systems.
Snowflake has some cutting-edge features. These include automatic scaling, secure data sharing, zero-copy cloning, time-travel capabilities, minimal maintenance overhead and strong security and governance features. Another big plus is its flexible architecture that decouples compute from storage, offering flexibility and cost efficiency. What's more, Snowflake works well with both structured and semi-structured data out of the box. And, it integrates seamlessly with AWS, Azure, and Google Cloud, which makes it a top choice.
According to DB-Engines, Snowflake is now number 7th in the rankings (as of October 2024), with a score of 140.60, showing how quickly it's risen to the top player in data warehousing. The industry has taken notice, and Snowflake is cementing its reputation as a market leader.
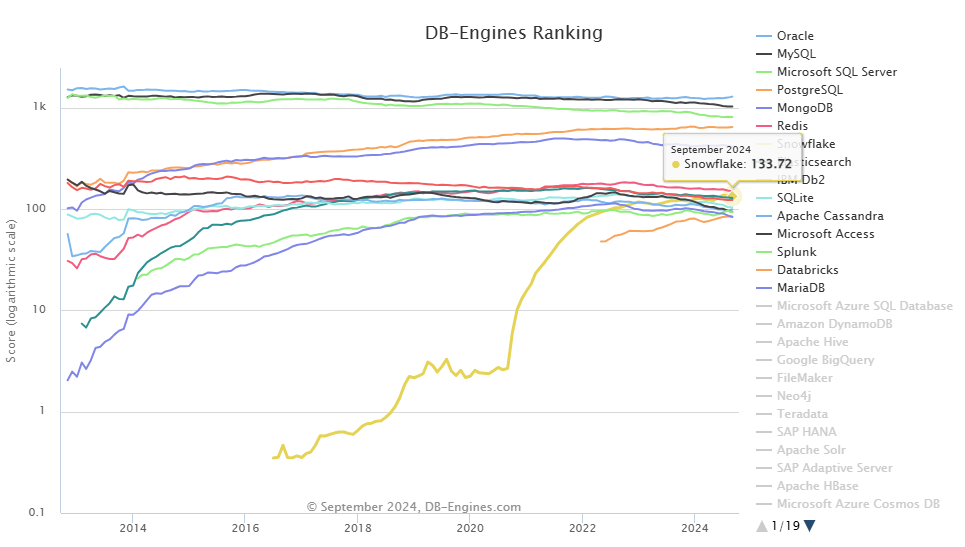
But Snowflake is up against stiff competition. There are a lot of other options out there that offer similar features and performance, all contending for the top spot. In this article, we will explore 16 top competitors—analyzing their architecture, features, performance, pricing, and use cases—to provide a comprehensive comparison of how they measure up against Snowflake.
Table of Contents
16 Real Snowflake Competitors—Which One Suits Your Data Needs?
1) Databricks
Databricks is a cloud-based data lakehouse platform that combines data engineering, data science, and analytics all in one place. It works seamlessly with big cloud providers like AWS, Azure, and Google Cloud, so organizations can easily scale their data solutions. Databricks is especially known for its lakehouse architecture, which brings together the best of both worlds—data lakes and data warehouses. This means users can handle a wide range of data, from structured to unstructured, with real ease.
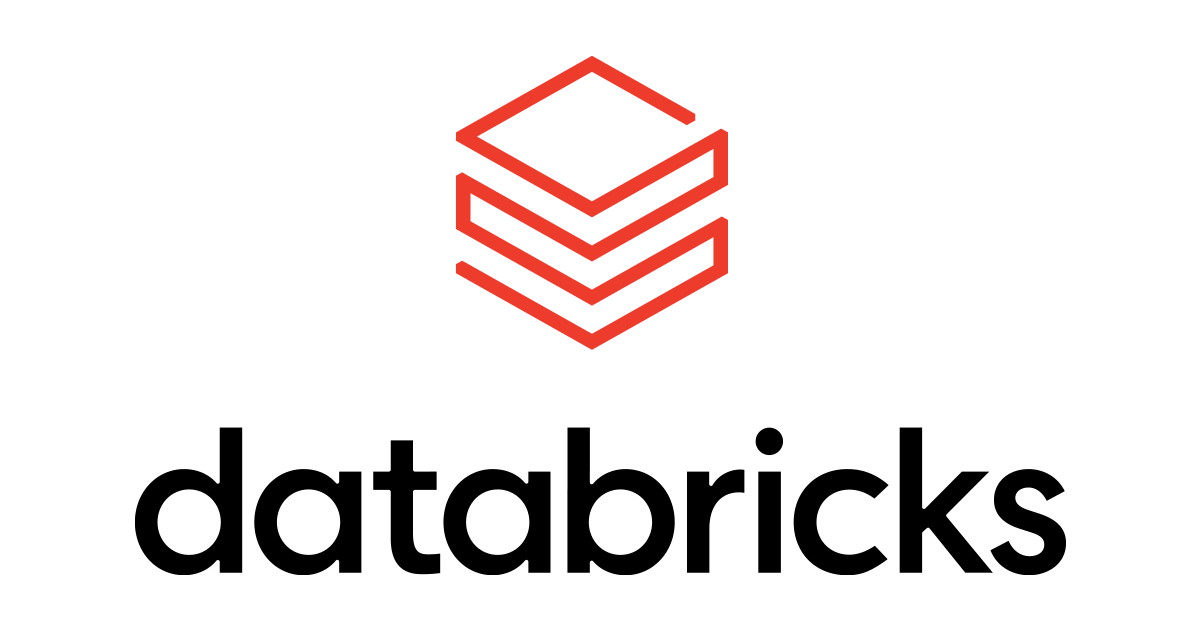
Want to take Chaos Genius for a spin?
It takes less than 5 minutes.
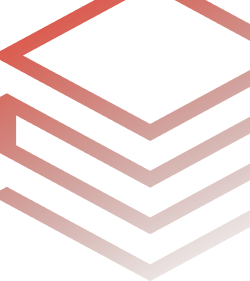
Intro To Databricks - What Is Databricks
Databricks Architecture
Databricks architecture is designed to seamlessly integrate with major cloud providers such as AWS, Azure, and Google Cloud. This versatility allows it to provide an optimized environment for data engineering, machine learning, and analytics at scale. Databricks adopts a Lakehouse Architecture, which blends the benefits of data lakes and data warehouses. The platform offers a hybrid Platform-as-a-Service (PaaS) model, consisting of two key components—the Control Plane and the Compute Plane—both of which are crucial for the platform's scalability, management, and performance optimization.
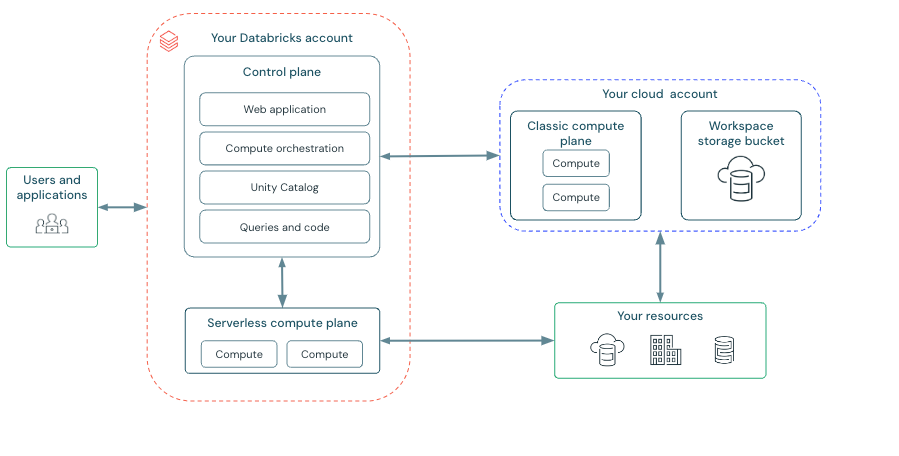
Control Plane is fully managed by Databricks and includes backend services, such as the web application, REST APIs for account management, and workspace administration. It provides an intuitive graphical interface for users to interact with notebooks, jobs, and clusters. This layer handles user authentication and metadata management, ensuring secure access and governance across the platform.
All operations within the control plane, including metadata management (cluster states, job configurations, and table schemas), are hosted and governed by Databricks.
Compute Plane is where the actual data processing takes place. The platform provides two types of compute environments, offering flexibility depending on security, control, and performance requirements:
- Serverless Compute Plane: A fully managed environment where Databricks automatically handles resource provisioning and scaling.
- Classic Compute Plane: In this setup, compute resources run within the user's cloud account (e.g., AWS or Azure), providing greater control over networking and security policies.
Databricks integrates with cloud-based storage solutions for data persistence. Each workspace has an associated storage bucket (e.g., Amazon S3 for AWS or Azure Blob Storage) that holds system data, notebooks, job details, and logs. This storage layer supports the Databricks File System (DBFS), enabling efficient data access and management.
Check out the video below for a deeper dive into Databricks architecture.
Tutorial - Databricks Platform Architecture - Snowflake Alternatives - Snowflake Competitors
Here are some key features of Databricks:
1) Databricks brings together the best of both worlds—data lakes and data warehouses—in one platform where you can store, process, and analyze your data.
2) Databricks integrates deeply with Apache Spark, enabling distributed data processing at scale using familiar languages like SQL, Python, Scala, and R. It is optimized for large-scale data processing and analytics.
3)Databricks enhances data reliability with Delta Lake, which supports ACID transactions, scalable metadata handling, and efficient data versioning for consistent data pipelines.
4) Databricks offers seamless integration with MLflow, providing comprehensive lifecycle management for machine learning models, including experiment tracking, model packaging, and deployment.
5) Databricks supports SQL-based analytics with optimized execution, enabling users to run ad-hoc queries and create interactive dashboards for real-time insights.
6) Databricks enables secure, real-time data sharing across various platforms with Delta Sharing, allowing collaboration without data replication.
7) Databricks provides centralized governance through Unity Catalog ensures fine-grained access control across all data assets, enhancing data security and regulatory compliance.
8) Databricks simplifies incremental data loading from cloud object storage with Auto Loader, a scalable tool for efficiently managing streaming data pipelines.
9) Databricks provides libraries and tools to build, train, and deploy ML models at scale.
… and so much more!
Pros and Cons of Databricks
Pros of Databricks:
- Easily handles small to large datasets effortlessly.
- Leverages Apache Spark for quick data analysis.
- Simplifies data pipeline creation and management.
- Supports real-time teamwork with shared notebooks.
- Provides comprehensive tools for the machine learning lifecycle.
- Very strong access controls and encryption features.
- Consumption-based pricing model.
- Compatible with AWS, Azure, and Google Cloud.
- Strong support for machine learning and AI workloads.
Cons of Databricks:
- More complex setup compared to Snowflake.
- Requires expertise in Spark for optimal use.
- Time-consuming initial configuration required.
- Lacks intuitive dashboard creation tools.
- Can be expensive for smaller-scale initiatives.
Databricks vs Snowflake—Which is the Better Choice?
When comparing Databricks and Snowflake, consider their key differences. Think about their architectures, use cases, and performance strengths.
Databricks, built on Apache Spark, is optimized for big data processing, machine learning (ML), and advanced analytics. It excels at handling large-scale, distributed data pipelines and real-time streaming workloads. Databricks supports processing multi-modal data (structured, semi-structured, and unstructured) and offers integrated tools like MLflow to manage the machine learning lifecycle from start to finish. Its Delta Lake layer adds ACID transactions, versioning, and data governance to your data lakes—turning them into reliable data lakes. Databricks is powerful and flexible. But, it requires some expertise to configure and optimize clusters, especially when scaling complex data workflows.
So, what about Snowflake? It's a fully managed cloud-native data warehouse designed for simplicity and scalability. A major benefit is its separation of compute and storage, which allows for independent scaling. This flexibility makes Snowflake well-suited for handling both structured and semi-structured data, perfect for BI and analytics tasks. Also, its automatic partitioning, query optimization, maintenance-free scaling, and a whole lot more features make Snowflake a perfect go-to choice. Snowflake's architecture also automatically manages tasks such as caching and compression, freeing you from manual effort.
When all is said and done, it depends on your goal. Are you focused on advanced analytics and machine learning with Databricks? Or, do you want data warehousing and simplicity with Snowflake?
🔮 TL;DR:
- Databricks: Best for real-time data processing, machine learning, and advanced analytics with highly customizable clusters.
- Snowflake: Best for SQL analytics, data warehousing, and business intelligence, with automatic performance tuning and ease of use.
For a deeper look at how Databricks vs Snowflake compare against each other, check out this article on Databricks vs Snowflake.
Want to take Chaos Genius for a spin?
It takes less than 5 minutes.
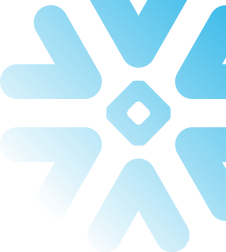
2) Google BigQuery
BigQuery is a fully managed, serverless data warehouse that is part of the Google Cloud Platform (GCP). It is designed to handle petabyte-scale datasets and offers the ability to scale storage and compute resources independently. Its serverless nature means users can focus on querying and analyzing data without managing any underlying infrastructure, offering simplicity in operations and reducing administrative overhead.
What is BigQuery?
BigQuery’s architecture is built on key components that make it scalable and efficient for large-scale analytics workloads:
1) Dremel Execution Engine
First up, BigQuery has the Dremel Execution Engine, which is the brain behind fast SQL query processing. The engine employs a multi-level execution tree that distributes queries across thousands of nodes, achieving low-latency query execution.
2) Colossus Storage System
Next, BigQuery leverages the Colossus distributed storage system for its data storage layer. Colossus ensures durability, availability, and scalability by storing data across multiple locations, with built-in replication and encryption for reliability and security.
3) Borg
Borg, Google’s cluster management system (which later inspired Kubernetes), efficiently schedules BigQuery workloads across GCP's infrastructure, ensuring optimal resource utilization. This helps in managing distributed resources for compute-heavy queries.
4) Jupiter Network
And don’t forget the Jupiter Network—BigQuery’s high-speed network that delivers data quickly. This guarantees efficient data transfer and reduces latency in data retrieval for queries, especially when working with large-scale datasets.
As we have mentioned already, the beauty of Google BigQuery’s architecture is that it’s decoupled, meaning storage and compute resources operate separately. This separation allows users to scale storage as needed, without impacting compute resources, and vice versa—optimizing costs and enhancing performance.
For further details, take a look at this article on Google BigQuery Architecture.
Key features of Google BigQuery are:
1) BigQuery's fully managed, serverless platform eliminates the need for infrastructure management. Users can focus on analytics tasks rather than worrying about provisioning or scaling resources.
2) BigQuery automatically scales computing resources based on the complexity and size of your queries.
3) BigQuery supports real-time streaming data ingestion through APIs like Streaming Inserts. This capability allows for near-instantaneous analytics and reporting on rapidly changing datasets.
4) BigQuery offers full support for ANSI SQL, making it accessible to data analysts and engineers already familiar with SQL.
5) BigQuery ML enables data scientists and analysts to build and train machine learning models directly within BigQuery using SQL syntax. This tight integration allows for quick prototyping of models without needing separate ML infrastructure.
6) BigQuery uses a pay-per-query pricing model where users are charged based on the volume of data processed by queries.
7) BigQuery natively integrates with other Google Cloud services like Dataflow, Pub/Sub, and AI Hub.
Pros and Cons of Google BigQuery
Pros of Google BigQuery:
- No infrastructure management required.
- Fast query execution on petabyte-scale datasets.
- Seamless scaling for varying data sizes.
- Pay-as-you-go pricing model allows flexibility, paying only for the queries processed.
- Leverages Google's serverless architecture to autoscale compute resources, optimizing costs.
- Supports streaming data ingestion, enabling real-time analysis of continuously updating datasets.
- Easy integration with other Google Cloud services.
- Create and run ML models using SQL with BigQuery ML.
- Robust encryption and compliance with industry standards.
- BigQuery integrations like BigQuery Omni allow unified analytics across data stored on AWS, Azure and GCP.
Cons of Google BigQuery:
- Potentially high costs with frequent small queries or large data processing needs.
- Less flexibility compared to on-premises or self-managed cloud solutions.
- Can be complex for new users unfamiliar with SQL or cloud services.
- Risk of vendor lock-in within GCP's ecosystem.
- Fewer integrations with third-party tools.
- Pricing can become complex based on usage patterns.
- Challenges in enterprise-level data modeling.
- Limited control over infrastructure compared to traditional solutions.
Google BigQuery vs Snowflake—Which is the Better Choice?
When picking between Google BigQuery and Snowflake, consider your organization's data needs and operations. Both are top cloud data warehouses, yet differ in design, cost, and strengths. That's why they're better suited for different jobs.
Snowflake is a multi-cloud data warehousing solution that offers more flexibility regarding cloud provider choice—working seamlessly across AWS, Azure, and Google Cloud. Its decoupled storage and compute architecture allows for fine-tuned performance management, offering users more granular control over resource allocation. Snowflake’s support for sharing data across different clouds, combined with its strong data marketplace ecosystem, makes it ideal for companies with multi-cloud environments and the need for cross-cloud analytics.
On the other side, BigQuery is a solid match for organizations that are already set up on Google Cloud or prioritize ease of use. By being serverless, BigQuery cuts out operational hassle, which is a big win for teams that don't want to deal with managing infrastructure. Its real strength is handling huge analytics workloads with barely any effort, making it perfect for those focused on checking out massive datasets and machine learning workloads within GCP.
🔮 TL;DR: The choice between Google BigQuery vs Snowflake really comes down to a few factors: which cloud provider you prefer, how much flexibility you need, and how easy you want it to be to use. BigQuery is great for teams already rooted in GCP, offering a super hands-off experience. On the other hand, Snowflake gives you the flexibility to work across different clouds and fine-tune your resource usage, making it a top pick for multi-cloud approaches.
For a more detailed comparison, check out our article comparing Google BigQuery vs Snowflake.
3) Amazon Redshift
Amazon Web Services (AWS) introduced Redshift in 2013 as a fully managed, petabyte-scale cloud data warehouse service designed for high-performance analytics and complex queries on large datasets. It uses a Massively Parallel Processing (MPP) architecture and columnar storage to improve query performance, allowing organizations to analyze terabytes to petabytes of data rapidly. Redshift supports seamless integration with AWS services like S3, Glue, and EMR, along with external tools such as business intelligence (BI) platforms and more. Redshift also provides elasticity in compute and storage through its Elastic Resize and Concurrency Scaling features, making it a powerful solution for scalable data warehousing with an on-demand pricing model.
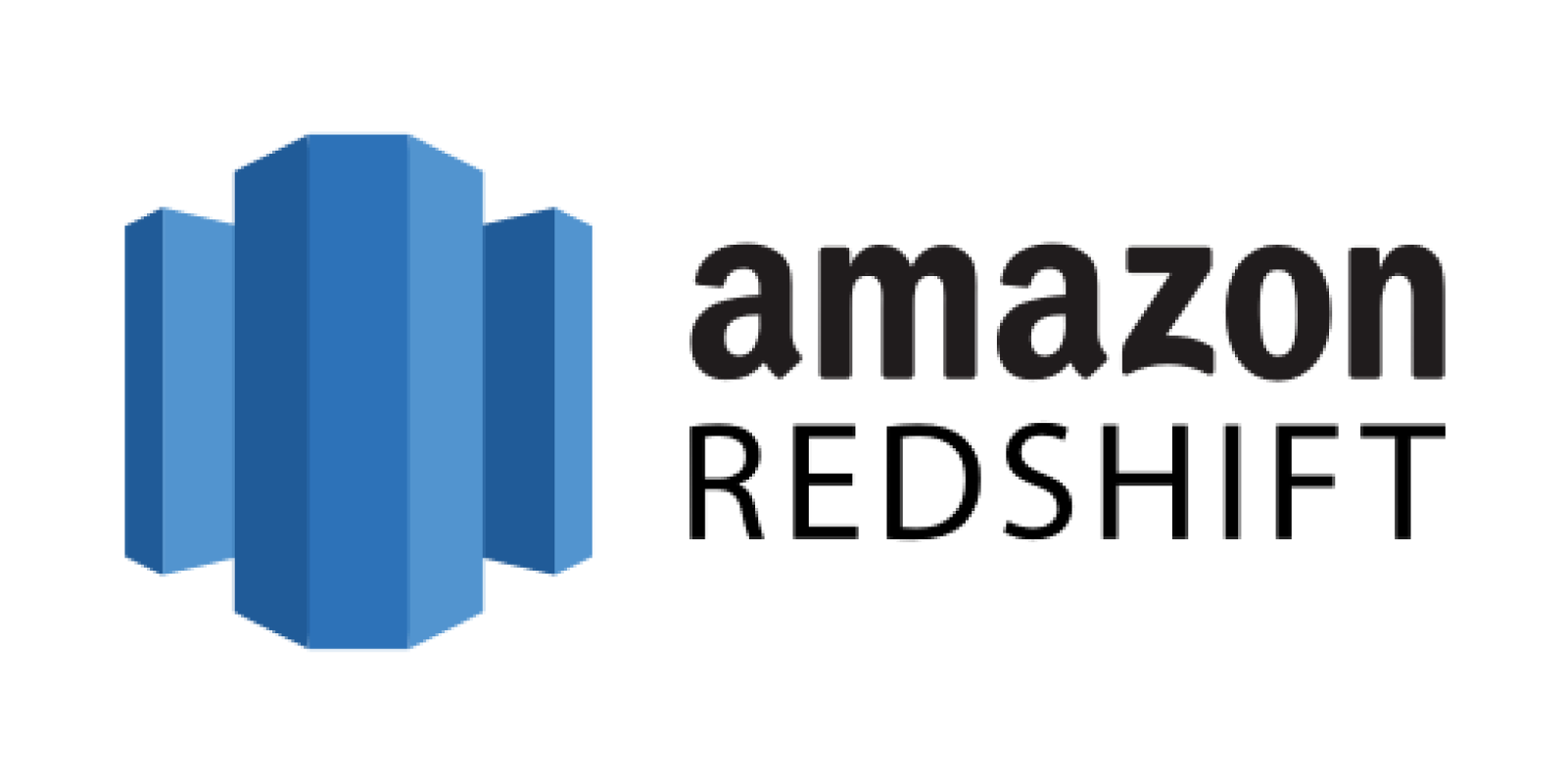
Amazon Redshift's architecture is designed for performance, scalability, and efficiency, comprising key components that ensure seamless data processing and analytics. The core components of this architecture include:
1) Leader Node
Leader Node manages the cluster, coordinating all communication between client applications and the compute nodes. It handles SQL query parsing, query optimization, and workload distribution. It performs several critical functions:
- Query Reception and Parsing: The leader node receives SQL queries, optimizes them, and creates an execution plan.
- Workload Distribution: It compiles SQL into optimized machine code (C++) and distributes the tasks to compute nodes.
- Result Caching: Frequently queried data may be cached in memory to accelerate repeated queries if underlying data hasn’t changed.
Compute nodes execute the database operations and store the data. They receive the execution plans from the leader node and perform the required operations. Amazon Redshift supports following types of node families:
- RA3 Nodes: These decouple storage and compute, allowing for dynamic storage scaling via Redshift Managed Storage (RMS) on Amazon S3.
- DS2 and DC2 Nodes: Legacy node types where Dense Compute (DC2) nodes offer high-performance SSD storage for compute-heavy workloads, while Dense Storage (DS2) nodes use HDD for cost-effective large-scale storage.
3) Redshift Managed Storage (RMS)
Introduced with RA3 nodes, RMS stores data on scalable Amazon S3 storage. It allows for automatic tiering of data, keeping frequently accessed data in high-speed SSD-based cache on compute nodes while moving colder data to S3, which enables elasticity in storage independent of compute.
4) Node Slices
Each compute node is divided into smaller units called slices, which allocate specific portions of CPU, memory, and disk resources for parallel processing. This division allows multiple slices within a compute node to work simultaneously on different segments of a query, optimizing resource utilization and improving performance.
5) Cluster
Redshift cluster consists of one or more compute nodes, with an optional leader node if there are multiple compute nodes. The cluster handles data storage and query execution. Clusters can be scaled up or down by adding/removing nodes, and Redshift supports Elastic Resize for adjusting resources based on demand.
6) Internal Network
Amazon Redshift uses a private, high-speed network between the leader node and compute nodes, allowing for fast communication between them.
7) Databases
Each Redshift cluster can host multiple databases that store user data. The leader node manages query coordination, delegating query execution tasks to compute nodes, which retrieve and process data from the relevant databases.
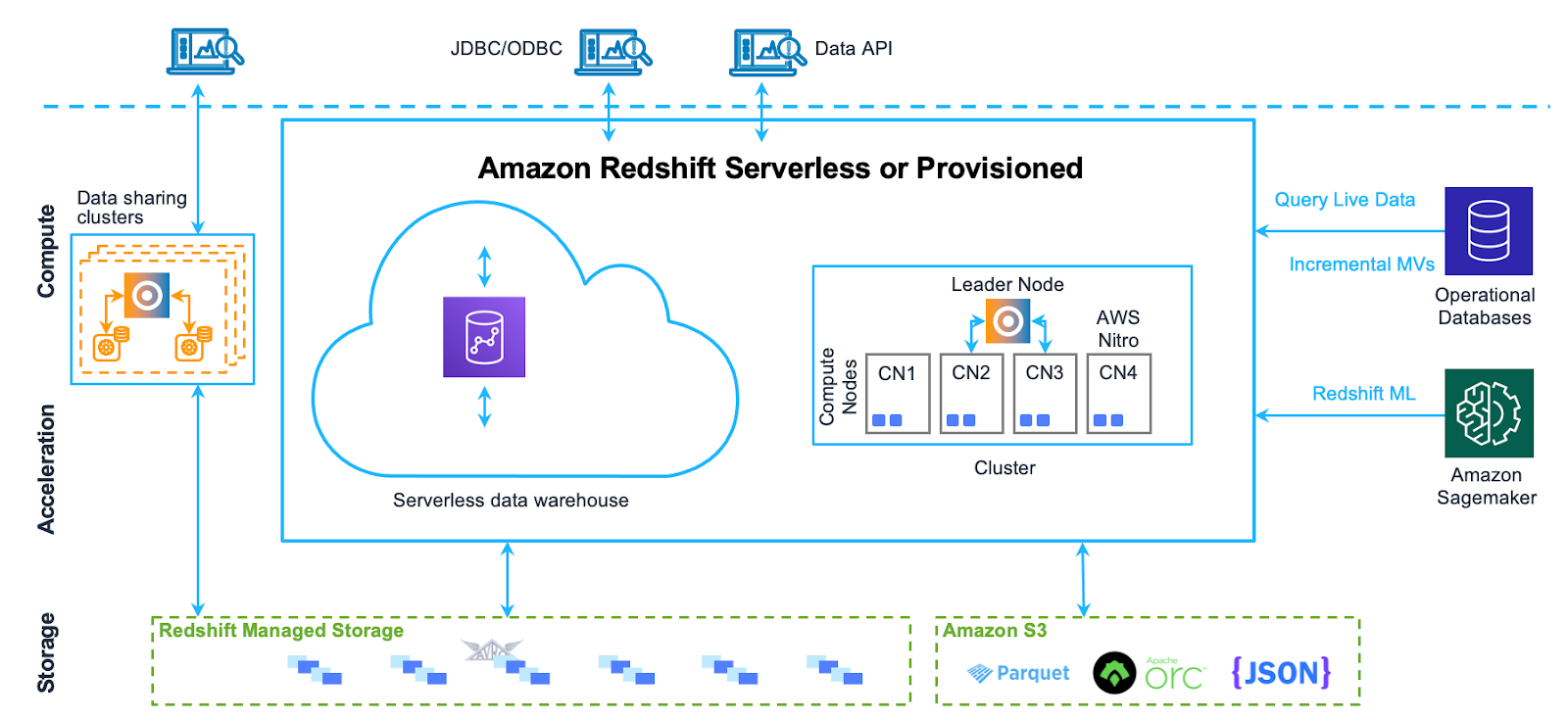
Pros and Cons of Amazon Redshift
Pros:
- High-performance queries due to columnar storage, MPP architecture, and result caching.
- Scales compute capacity easily with elastic resize.
- Concurrency Scaling supports dynamic scaling for peak workloads, improving query throughput during high-demand periods.
- Cost-effective for large-scale data warehousing.
- Materialized views and result caching improve query speed.
Cons:
- Performance tuning (e.g., vacuuming, analyzing tables) requires specialized expertise.
- Not ideal for real-time analytics as Redshift is optimized for batch processing.
- Certain tasks like manual vacuuming and compression still require manual intervention.
- Poor configuration choices can severely impact performance
- Cost management becomes complex as data scales due to storage and compute bundling (except for RA3 nodes with separate scaling).
- Limited support for semi-structured data compared to competitors like Snowflake, which natively handles JSON, Avro, and Parquet more effectively.
Amazon Redshift vs Snowflake—Which One Should You Opt For?
When comparing Amazon Redshift vs Snowflake, consider factors like data management flexibility, architeture, scalability, workload types, and integration needs.
Snowflake offers a fully managed service with a focus on ease of use and operational efficiency. It natively separates compute from storage, allowing users to independently scale each resource based on their workload, which provides more granular cost control. Snowflake also excels in handling semi-structured data formats (e.g., JSON, Avro, Parquet) without requiring manual transformations. This makes it perfect for organizations that need rapid scaling and flexible data processing, especially those leveraging data lakes.
On the flip side, Redshift, integrates deeply with the AWS ecosystem, providing cost savings through Reserved Instance pricing and tight integration with other AWS services like S3, Glue, Lambda and so much more. It is particularly suited for enterprises that require high performance for predictable, long-term workloads. While Redshift may require more hands-on management, the platform provides significant cost advantages for organizations committed to AWS and willing to invest in performance optimization.
🔮 TL;DR:
- Choose Snowflake if you need a hands-off, scalable solution that excels at handling diverse data types with minimal management overhead.
- Opt for Redshift if you prefer deep AWS integration, need cost-effective solutions for large, predictable workloads, and don’t mind additional management tasks for optimization.
Amazon Redshift vs Snowflake
For even more detailed comparison, check out our article comparing Amazon Redshift vs Snowflake.
4) Microsoft Azure Synapse Analytics
Microsoft Azure Synapse Analytics is a comprehensive cloud-based analytics service that integrates data warehousing, big data processing, and data integration into a single platform. Originally known as Azure SQL Data Warehouse, it has evolved significantly, incorporating advanced features such as serverless and dedicated SQL pools (formerly SQL DW), Apache Spark integration, and seamless connectivity with other Azure services (such as Power BI and Azure Machine Learning).
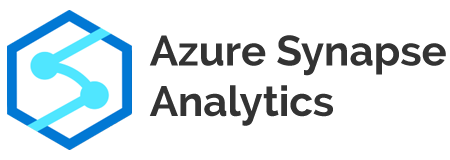
Here are some key features of Azure Synapse Analytics:
1) Unified Analytics Platform — Microsoft Azure Synapse Analytics combines enterprise data warehousing, big data analytics, and data integration into a single, cohesive service, facilitating a wide range of data-driven tasks from ETL to advanced analytics.
2) Serverless and Dedicated Resources — Microsoft Azure Synapse Analytics offers two resource models: serverless SQL pools for on-demand querying without infrastructure management, and dedicated SQL pools for provisioned resources, allowing users to choose based on workload requirements, balancing performance and cost.
3) Integration with Apache Spark — Microsoft Azure Synapse Analytics fully integrates Apache Spark, enabling scalable big data processing. Users can run complex transformations, machine learning models, and streaming analytics using languages like Python, Scala, and Spark SQL.
4) Synapse Studio — Microsoft Azure Synapse Analytics features Synapse Studio, a web-based interface that provides a unified workspace for developing, managing, and monitoring analytics solutions. It streamlines workflows across data preparation, querying, data exploration, and visualization, making it accessible to users of all expertise levels.
5) Power BI Integration — Microsoft Azure Synapse Analytics has deep integration with Power BI, enabling users to create interactive reports and dashboards from Synapse data, making it easier to derive actionable insights.
6) Advanced Security Features — Microsoft Azure Synapse Analytics offers robust security with end-to-end encryption, role-based access control, dynamic data masking, and compliance with major regulations like HIPAA, ISO and GDPR.
7) Machine Learning Capabilities — Microsoft Azure Synapse Analytics facilitates the integration of machine learning models using Azure Machine Learning and Spark MLlib, making it easy to embed predictive analytics and advanced models into data pipelines.
8) Low-Code and No-Code Capabilities — Synapse Studio includes drag-and-drop interfaces for building pipelines, creating transformations, and orchestrating data flows, reducing the need for complex coding.
9) Cost-Efficient Pricing Model — Microsoft Azure Synapse Analytics utilizes a pay-as-you-go pricing model, enabling you to pay only for the resources they use, making it cost-efficient for both small and large-scale workloads.
Microsoft Azure Synapse Analytics is highly scalable and versatile. The architecture comprises several key components, such as:
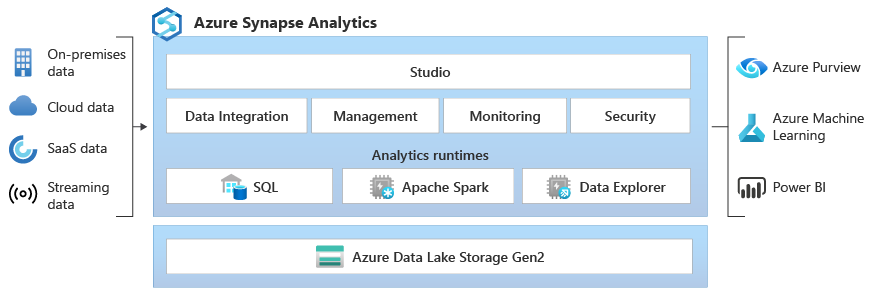
1) Control Node
Control Node manages query orchestration and optimization. It breaks large queries into distributed tasks for parallel execution across compute nodes, optimizing query performance. In both dedicated and serverless SQL pools, it coordinates the entire query lifecycle.
These provide the computational power. For dedicated SQL pools, the data is distributed across multiple nodes (up to 60), allowing parallel query execution. Serverless SQL pools dynamically allocate compute resources as needed, optimizing both performance and cost. In dedicated pools, users can scale compute resources independently of storage.
Azure Synapse a decoupled storage and compute architecture. Data is stored in Azure Data Lake Storage (ADLS) or Azure Blob Storage, allowing independent scaling of compute without affecting stored data, which ensures flexibility and cost optimization.
4) Data Movement Service (DMS)
Data Movement Service handles the transfer of data between compute nodes during distributed query execution. It ensures that data shuffling across nodes happens efficiently to maintain high query performance, especially in MPP environments.
5) SQL and Spark Integration
Synapse bridges SQL and Spark workloads seamlessly. Users can run T-SQL and Spark jobs on the same data stored in ADLS, supporting hybrid scenarios that combine big data analytics with traditional data warehousing.
6) Data Integration & Pipelines
Synapse integrates with Azure Data Factory (ADF), providing built-in capabilities for designing, scheduling, and orchestrating ETL/ELT pipelines. This allows users to ingest data from over 100+ sources, transform it, and load it without leaving the Synapse environment.
Pros and Cons of Azure Synapse Analytics
Pros of Microsoft Azure Synapse Analytics:
- Unified platform that combines data warehousing and big data analytics using both SQL and Spark engines, offering both serverless and dedicated resource options.
- Tight integration with Azure services like Power BI, Azure Data Factory, and Azure Machine Learning, making it highly versatile for AI/ML workflows.
- Uses Massively Parallel Processing (MPP) architecture, which significantly improves query speed and performance, especially for large-scale data processing
- Offers a user-friendly environment for building complex data workflows using low-code or no-code options
- AI/ML tools are integrated, making it easy to apply advanced analytics across large datasets
Cons of Microsoft Azure Synapse Analytics:
- Complex cost management — pricing models can be difficult to estimate, especially with fluctuating workloads in serverless pools.
- Steep learning curve — Synapse’s vast capabilities may overwhelm users during initial setup and usage.
- Not ideal for real-time analytics — Synapse can be less performant compared to specialized solutions like Snowflake for real-time data processing.
Snowflake vs Azure Synapse Analytics—Which One Should You Opt For?
If you’re choosing between Snowflake vs Azure Synapse Analytics, the decision depends largely on your organization's specific needs:
Snowflake is perfect for businesses looking for a highly scalable, cloud-native solution with strong multi-cloud support (AWS, Google Cloud, Azure). It offers effortless scalability, automatic performance tuning, and seamless multi-cloud operations. Its zero-copy cloning, time travel, and cross-cloud replication features provide high flexibility, and per-second billing optimizes cost-efficiency for businesses needing to scale dynamically.
Microsoft Azure Synapse Analytics is designed for businesses seeking deep integration with the Azure ecosystem, particularly for scenarios requiring a mix of data lake, data warehousing, AI/ML, and big data analytics. Its SQL and Spark integration, combined with Azure-native services, makes it a robust platform for complex, data-intensive workflows. However, it has a steeper learning curve and may require more manual optimization for dedicated SQL pools.
🔮 TL;DR: Choose Snowflake if you need a user-friendly, scalable, multi-cloud data warehouse. Opt for Azure Synapse Analytics if your organization relies on advanced analytics, requires deep integration with Azure services, and values the flexibility of combining SQL, Spark, and AI/ML workloads.
For even more detailed comparison, check out our article comparing Snowflake vs Azure Synapse Analytics
5) IBM Db2 Warehouse
IBM Db2 Warehouse is a cloud-native data warehouse solution designed for advanced analytics and big data processing. It is part of the broader IBM Db2 family, which encompasses various database management products. Db2 Warehouse provides a platform that allows organizations to store, manage, and analyze mixed analytics workloads across structured, semi-structured, and unstructured data formats.
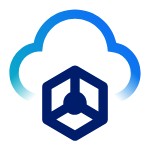
Here are some key features of IBM Db2 Warehouse:
1) IBM Db2 Warehouse leverages BLU Acceleration, which is an advanced in-memory processing engine that uses columnar storage and sophisticated data compression techniques, including Huffman encoding and other compression algorithms to reduce storage footprints and optimize query performance, which is crucial for real-time analytics.
2) IBM Db2 Warehouse supports open data formats like Apache Iceberg, Parquet, ORC, and CSV. These formats enhance data interoperability across different platforms and facilitate secure data sharing. Users can also share data in Iceberg with IBM watsonx.data lakehouse and use multiple query engines, such as Presto and Apache Spark, to augment workloads for price and performance.
3) IBM Db2 Warehouse is optimized for real-time analytics, supporting high-performance dashboards and reporting tools. This is achieved through a combination of in-memory processing, columnar data storage, and data skipping techniques. The platform maximizes CPU utilization and input/output operations, ensuring fast data retrieval and processing even for complex mixed workloads.
4) IBM Db2 Warehouse is built with a 99.9% uptime guarantee to guarantee enterprise-grade continuous availability. Its cloud-native architecture includes disaster recovery features like in-place recovery inside clusters and multi-cloud replication.
5) IBM Db2 Warehouse is built with robust security mechanisms to ensure data protection, both in transit and at rest. It provides end-to-end encryption (including Transparent Data Encryption, TDE), and features like audit trails and fine-grained access control.
6) IBM Db2 Warehouse is also compliant with industry standards such as HIPAA and GDPR, making it suitable for highly regulated industries. On top of that, it can be integrated with IBM Knowledge Catalog to guarantee centralized data governance and enforce security policies.
7) IBM Db2 Warehouse offers APIs for application integration and management, allowing developers to interact programmatically with the warehouse environment.
8) IBM Db2 Warehouse includes native machine learning capabilities, supporting a wide range of algorithms. Users can also integrate Python and R models for more advanced analytics and data science workflows. The platform supports various data types (e.g., JSON, XML, spatial, and graph data), making it a versatile environment for complex data-driven applications.
IBM DB2 Warehouse
Pros and Cons of IBM Db2 Warehouse
Pros IBM Db2 Warehouse:
- IBM Db2 Warehouse is capable of handling massive data volumes, making it ideal for enterprise-level projects.
- IBM Db2 Warehouse is optimized for fast data retrieval with in-memory processing and data skipping techniques.
- IBM Db2 Warehouse features end-to-end encryption, fine-grained access control, and compliance with standards like HIPAA and GDPR.
- IBM Db2 Warehouse supports various open formats like Apache Iceberg, and can integrate with tools like Presto and Apache Spark
- IBM Db2 Warehouse has built-in support for machine learning models, with integration for Python and R workflows.
- IBM Db2 Warehouse offers robust uptime guarantees (99.9%) and built-in disaster recovery features.
Cons IBM Db2 Warehouse:
- Can be expensive to implement and maintain, making it less suitable for small-to-medium-sized businesses with limited budgets.
- Requires specialized skills for administration and optimization, which can increase operational overhead.
- IBM Db2 is great for certain workloads, it may be outperformed by other cloud-native platforms (like Snowflake) on specific query types.
Snowflake vs IBM Db2 Warehouse—Choosing the Right Platform
So, the final choice between Snowflake vs IBM Db2 Warehouse depends on your specific needs, as each excels in different ways. Both platforms offer distinct advantages.
Snowflake is perfect for organizations looking for a highly scalable, cloud-native data warehousing solution that offers flexibility in compute and storage. Its architecture lets users split these components, so they only pay for what they actually use. This means they can optimize costs easily. Snowflake handles all kinds of data, from totally structured to semi-structured formats, which is great for anyone that need to share data easily and quickly without a lot of maintenance headaches. Many users love how easy it is to get started and that it doesn't require much fine-tuning, which helps them work more efficiently and reduces operational hassle.
IBM Db2 Warehouse is a good fit for companies that need a robust analytics engine to handle large amounts of structured and unstructured data. It uses IBM's BLU Acceleration for fast analytics and advanced workload management. Companies already invested in IBM, or needing tight integration with IBM products, will find Db2 Warehouse useful. One of its key strengths is letting users run complex queries and machine learning models without having to move data out of the warehouse, which makes things run more smoothly.
🔮 TL;DR:
- Choose Snowflake if you need a flexible, easy-to-use platform that excels in scalability and handling semi-structured data with minimal maintenance.
- Go for IBM Db2 Warehouse if you need enterprise-grade analytics integrated within the IBM ecosystem with strong compliance features and AI-driven workloads.
6) Oracle Analytics Platform
Oracle Analytics Platform is a unified, all-in-one solution that helps with advanced data analytics, no matter where the data is—in the cloud, on-premises, or a mix of both. It gives business users, data engineers, and data scientists the power tools they need to easily access, process, and analyze data from all sorts of sources. Since it's tightly integrated with the rest of Oracle's services, Oracle Analytics provides insights you can act on, so you can make informed decisions based on current, complete, and accurate data.
Oracle Analytics Platform - Snowflake Competitors - Snowflake Alternatives
Here are some key main features of Oracle Analytics Platform:
1) End-to-End Analytics Workflow — Oracle Analytics provides a complete analytics pipeline—connecting to data sources, preparing and enriching data, exploring insights, visualizing results, and delivering them through intuitive storytelling interfaces. The platform’s powerful built-in connectors integrate data from a variety of sources, including Oracle Autonomous Data Warehouse (ADW), Oracle Cloud Infrastructure (OCI), and non-Oracle systems.
2) Embedded Machine Learning — Oracle Analytics Platform integrates machine learning capabilities throughout its services. This allows organizations to transition from being merely data-driven to becoming analytics-driven by leveraging predictive insights.
3) Customizable Analytics — Unlike many other analytics solutions, Oracle Analytics Platform offers a flexible balance between centralized governance and self-service analytics.
4) Hybrid and Multi-Cloud Flexibility — One of the key differentiators of Oracle Analytics is its flexible deployment model. It supports:
a) Oracle Analytics Cloud (OAC)
Oracle Analytics Cloud (OAC) is a cloud-native service built on Oracle Cloud Infrastructure (OCI), designed for organizations that are moving their data to the cloud. It provides advanced collaboration and visualization capabilities while eliminating the need for on-premises infrastructure.
b) Oracle Analytics Server (OAS)
For organizations preferring to keep their infrastructure on-premises, Oracle Analytics Server (OAS) offers a robust private cloud analytics solution. It allows users to start new deployments or migrate existing Oracle Business Intelligence Enterprise Edition (OBIEE) installations without additional cost. OAS delivers modern, AI-powered analytics while ensuring that all data remains on-premises.
Both of em’ provide capabilities like centralized governance for enterprise reporting and self-service analytics, guaranteeing flexibility for diverse business needs.
Why Use Oracle Analytics Platform?
The Oracle Analytics Platform provides:
1) Comprehensive Capabilities — It serves all roles within an organization—IT professionals, data engineers, citizen data scientists, executives, and business users—guranteeing that everyone has access to relevant analytics tools.
2) Embedded Machine Learning — Users benefit from machine learning capabilities tailored for different expertise levels, ranging from no-code solutions to customizable algorithms for specific use cases.
3) Centralized Governance with Self-Service — Oracle Analytics Platform strikes a balance between centralized reporting and self-service analytics while maintaining consistent and reliable metrics.
4) Abstracted Data Models — Oracle Analytics Platform offers a data model that abstracts physical sources and query languages from business users, simplifying the analytical process.
5) Secure Data Preparation — Built-in data preparation features are trackable and repeatable, reducing the need for insecure exports or reliance on Excel spreadsheets.
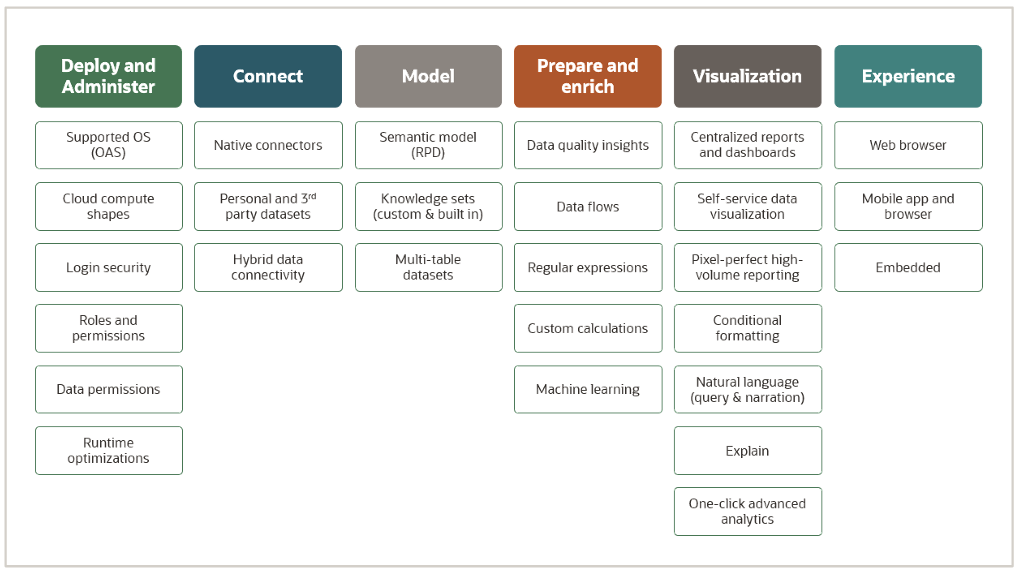
Pros and Cons of Oracle Analytics Platform
Pros of Oracle Analytics Platform:
- Strong support for ML features, including AutoML and one-click predictions, which enhance analytics capabilities.
- Offers compelling storytelling tools and supports interactive visualizations.
- Seamlessly integrates with various systems, particularly Oracle’s ecosystem, with over 40+ pre-built connectors.
- Drag-and-drop features make it relatively easy to use for most users, especially for non-technical teams.
- Flexible deployment options, allowing businesses to scale depending on workload, including hybrid deployments.
Cons of Oracle Analytics Platform:
- Generally considered expensive, especially compared to Snowflake.
- Many users find the initial setup and onboarding process complex, requiring significant time to master.
- Lacks some ready-to-use features, especially when compared to Snowflake.
- Licensing models can be confusing, especially when combined with Oracle’s database and middleware offerings.
Snowflake vs Oracle Analytics Platform—Which One Should You Opt For?
Welp! If you are choosing between Snowflake vs Oracle Analytics Platform depends on your organization’s specific data storage, governance, and analytics needs:
Snowflake is perfect for organizations who are seeking a fully cloud-native, scalable data warehousing solution with minimal administrative overhead. Its multi-cluster, shared-data architecture excels in handling massive data workloads and supports diverse analytics use cases across various cloud services. Snowflake’s strength lies in its seamless elasticity, cost-efficient scaling, and ease of integration with third-party tools for advanced analytics.
Oracle Analytics Platform is more suited for organizations that require an end-to-end analytics solution with robust support for hybrid, cloud and on-premises environments. Its tight integration with Oracle’s broader enterprise ecosystem, combined with built-in machine learning, makes it a powerful tool for organizations with complex governance and security requirements. Oracle’s advanced governance, data preparation, and data storytelling features make it an ideal choice for businesses looking for a comprehensive, governed, and flexible analytics solution.
🔮 TL;DR: If your primary goal is cloud-native scalability with minimal management overhead, Snowflake is the best option. But, if you need an advanced, integrated analytics platform with machine learning, hybrid deployment, and customizable analytics workflows, Oracle Analytics Platform may be the better choice.
7) Rockset (acquired by OpenAI)
Rockset is a real-time analytics database-as-a-service (DBaaS) optimized for low-latency SQL queries across large volumes of structured and semi-structured data, including formats (such as JSON, Parquet, and CSV). Designed to handle real-time indexing and querying, Rockset allows immediate analytics without the need for extensive data movement or pre-aggregation; especially beneficial for real-time applications such as monitoring, personalization, and live dashboards.
In 2024, Rockset was acquired by OpenAI, with the aim of enhancing the retrieval infrastructure behind large language models (LLMs).
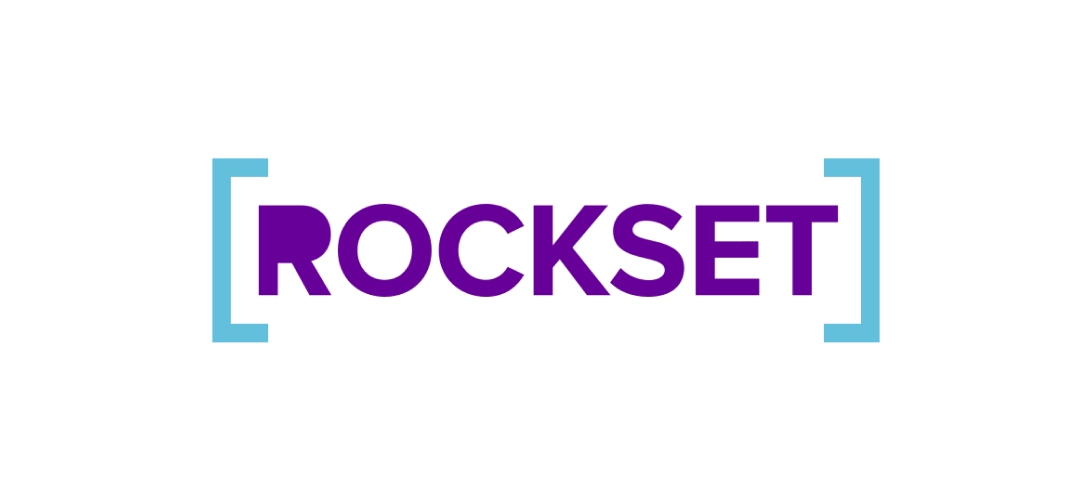
Here are some key features of Rockset:
1) Real-Time Indexing — Rockset provides real-time indexing with millisecond-level query latencies, eliminating the need for data pre-processing or predefined indexing.
2) Auto-Schema Resolution — Rockset dynamically detects and manages schemas for semi-structured data (e.g., JSON), allowing users to query new data streams immediately without manual schema management.
3) Cloud Agnostic — Rockset is compatible with any cloud provider (AWS, GCP, Azure), allowing seamless integration without vendor lock-in.
4) Enterprise-Grade Security — Rockset features end-to-end encryption for data in transit and at rest, along with role-based access control (RBAC) and compliance with SOC 2 standards.
5) Serverless Architecture — Rockset requires no infrastructure management, scaling compute and storage independently.
6) Event Stream Processing — Rockset handles real-time event data, offering fast analytics on continuous streams of data.
7) Millisecond-latency SQL Queries — Rockset delivers fast query results, optimized for real-time decision-making.
Check out this video to learn in depth about Rockset in detail:
Rockset Product Tour
Pros and Cons of Rockset:
Pros of Rockset:
- Delivers low-latency query results on semi-structured and structured data.
- Automatically handles shard distribution for easy scalability.
- Requires no infrastructure management, simplifying operational overhead.
- Supports complex SQL queries across various cloud platforms.
Cons of Rockset:
- Not suitable for transactional workloads.
- Can become costly for high-volume data-heavy use cases.
Choosing Between Rockset vs Snowflake —Which is Right for You?
When choosing between Rockset vs Snowflake, the decision depends heavily on your workload requirements:
Snowflake is perfect for batch processing and large-scale analytics on historical data. It leverages a cloud-native architecture with separate compute and storage, allowing efficient scaling for workloads like trend analysis, business intelligence, and machine learning. Snowflake’s columnar storage format ensures cost efficiency, but it can lag in real-time data queries due to its batch ingestion and scan-heavy query execution model.
Rockset, in contrast, is is optimized for real-time analytics,with low-latency queries on frequently updated data. Its Converged Index technology indexes all fields in real time, making it highly suitable for applications requiring up-to-the-minute insights, such as monitoring or personalization.
🔮 TL;DR: If you're choosing between Rockset and Snowflake, opt for Snowflake if you need batch processing and large-scale analytics on historical data. But, if real-time analytics on streaming or frequently updated data is critical, Rockset is the better choice.
8) Apache Pinot™
Apache Pinot is a completely open source, real-time distributed OLAP datastore, built to deliver scalable real-time analytics with low latency. It can ingest from batch data sources (such as Hadoop HDFS, Amazon S3, Azure ADLS, Google Cloud Storage) as well as stream data sources (such as Kafka).
It was originally developed by engineers at LinkedIn and Uber to handle mission-critical real-time analytics use cases.
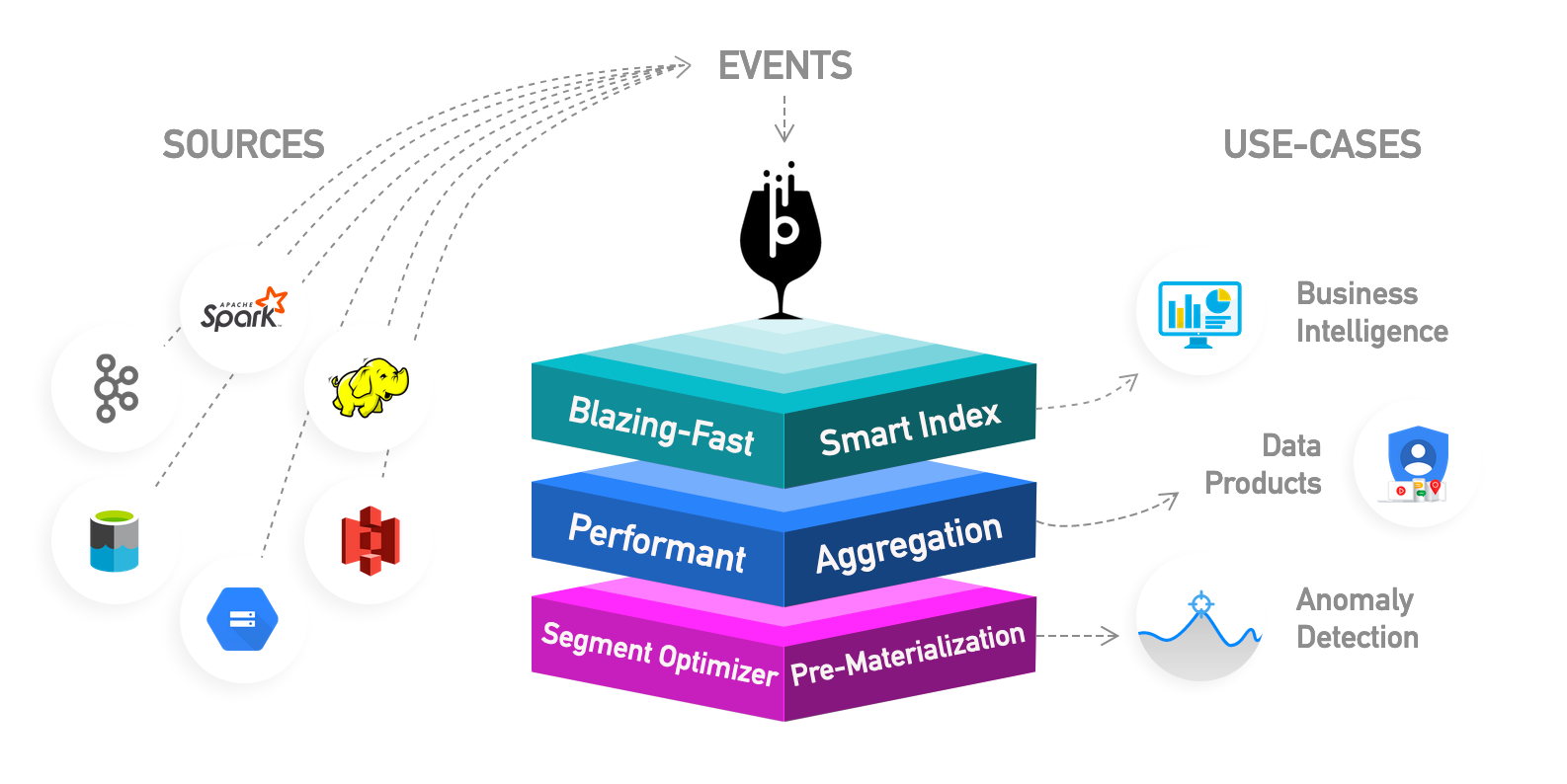
Pinot's architecture is built to handle large volumes of data with low latency and high concurrency. Below is a detailed overview of its architecture and components.
1) Core Apache Pinot Components:
a) Controller — Controller is the central orchestrator of the Pinot cluster. It manages the assignment of tables and segments to servers, oversees cluster state, and handles metadata operations. The controller operates using Apache Helix, which facilitates resource management in distributed systems.
b) Broker — Brokers act as intermediaries that receive queries from clients and route them to the appropriate Pinot servers. They consolidate the results from multiple servers before returning a unified response to the client.
c) Server — Servers are responsible for hosting segments (the basic units of data storage) and executing queries against these segments. Each server can independently scale horizontally by adding more nodes as needed.
d) Minion — Minion is the optional component executes background tasks such as data ingestion from batch processes and optimizing segments. Minions help offload processing tasks from the main servers, enhancing overall system efficiency.
2) Data Storage:
a) Segments — Data in Apache Pinot is divided into segments, which are immutable collections of rows that can be processed independently. Each segment contains a metadata file and an index file, allowing for efficient querying.
b) Columnar Storage — Apache Pinot stores data in a columnar format, which optimizes read performance for analytical queries by allowing efficient access to specific columns rather than entire rows.
c) Indexing — Apache Pinot supports various indexing techniques (e.g., inverted indexes, star-tree indexes) that enhance query performance by allowing faster data retrieval based on specific query patterns.
3) Data Ingestion:
a) Real-time and Batch Ingestion — Apache Pinot supports both real-time ingestion from streaming sources like Apache Kafka and batch ingestion from data lakes or file systems like HDFS or Amazon S3. This dual capability makes sure that data is available for analysis almost immediately after it is produced.
b) Deep Store — Segments are initially stored in a deep store (like cloud storage) before being loaded into servers for querying. This architecture allows for high availability and fault tolerance since segments can be replicated across multiple servers.
4) Cluster Management:
a) Apache Helix Integration — Apache Pinot uses Apache Helix for managing its cluster state, including partitioning and replication of segments across different servers. Helix coordinates the health and status of each component within the cluster.
b) Apache Zookeeper Integration — Apache Zookeeper is used to maintain metadata about the cluster state, ensuring that all components have a consistent view of the system's health and configuration.
What is Apache Pinot? (and User-Facing Analytics) | A StarTree Lightboard by Tim Berglund
Here are some key features of Apache pinot.
1) Low Latency & High Throughput — Apache Pinot is designed to handle ultra-low-latency queries (as low as 10ms) with high concurrency, making it suitable for user-facing applications requiring real-time insights.
2) Scalability — Apache Pinots architecture allows for horizontal scaling by adding more servers or brokers without downtime or loss of performance.
3) Fault Tolerance — Because there is no single point of failure, if one node fails, others can continue to execute requests without interruption thanks to segment replication.
4) Immutable Data Handling — Apache Pinot enables upserts from streaming data sources, allowing for flexible data handling while retaining performance.
Pros and Cons of Apache Pinot™
Pros of Apache Pinot:
- Optimized for real-time OLAP queries, Pinot can deliver low-latency results for time-series and event-based data streams.
- Supports horizontal scaling, capable of handling massive data volumes and distributed across thousands of nodes.
- Efficiently processes millions of queries simultaneously, making it ideal for user-facing applications.
- Built to serve queries with minimal latency, even for large datasets.
- Offers a variety of indexing techniques such as inverted, star-tree, and sorted indexes, providing tunable query performance.
- Automatically replicates and distributes data for fault-tolerance, ensuring high availability and system reliability.
- Uses columnar storage, optimizing the performance for OLAP workloads with minimized storage overhead.
Cons of Apache Pinot:
- Managing and configuring Pinot clusters, especially in production environments, requires expertise and considerable setup effort.
- It can consume significant system resources (CPU, memory, and storage), especially in high-throughput scenarios.
- While Pinot supports SQL-like querying, its query language is limited compared to more traditional relational databases.
- Pinot's strength lies in real-time analytics; it may not be as efficient for batch processing or historical data analysis.
Apache Pinot vs Snowflake—Which One Should You Opt For?
So, if you are deciding between Apache Pinot vs Snowflake, it’s essential to understand that they serve different purposes and have distinct architectures optimized for specific use cases.
Snowflake is a cloud-based data warehousing platform designed for batch processing and large-scale analytics on structured data. It excels in:
- General-purpose data analytics across structured and semi-structured data.
- Batch processing of large historical datasets.
- Elastic scaling in a cloud-native environment, automatically managing resources.
- Simplified management—with automatic scaling, partitioning, and optimization—ideal for business intelligence, reporting, and machine learning use cases where complex queries run on large historical datasets.
Apache Pinot™, on the other hand, is optimized for real-time OLAP queries on event streams and time-series data. It stands out in:
- Real-time analytics with ultra-low latency (as low as 10ms) and high concurrency.
- User-facing applications requiring instant query results (e.g., dashboards, monitoring tools).
- Handling event-driven data such as time-series and streaming analytics from sources like Kafka.
- Distributed architecture enabling fault tolerance, high availability, and horizontal scalability for real-time data ingestion and query execution.
🔮 TL;DR:
- Choose Snowflake if you need a robust, managed data warehouse solution for complex analytics over historical datasets, especially in the context of batch processing.
- Choose Apache Pinot if you need real-time, low-latency analytics on streaming data or time-series data, especially for high-concurrency, user-facing applications.
9) Teradata
Teradata is a highly scalable enterprise data warehouse (EDW) solution that leverages a Massively Parallel Processing (MPP) architecture for high-performance data analytics. Teradata enables users/organizations to store, process, and analyze large datasets from diverse sources in structured, semi-structured, and unstructured formats. Teradata’s core strength lies in its ability to handle complex queries and mixed workloads at scale, making it a reliable choice for data-heavy industries.
Teradata was first founded in 1979 (~45 years ago) by Jack E. Shemer, Philip M. Neches, Walter E. Muir, Jerold R. Modes, William P. Worth, Carroll Reed, and David Hartke. Teradata pioneered the concept of MPP, which distributes data and query workloads across multiple nodes—each with its own processing resources (CPU, memory, disk)—to achieve high throughput, scalability, and fault tolerance. Teradata also introduced the concept of shared-nothing architecture, which minimizes data contention between nodes, ensuring maximum parallelism and system efficiency.
Teradata has transitioned from being an on-premises EDW solution to offering flexible deployment options, including cloud, hybrid, and on-premises configurations. Some of Teradata’s modern offerings are:
Teradata also provides tools like Teradata Studio & Studio Express, Teradata Data Fabric, Teradata ClearScape Analytics, and Teradata Data Lab for data integration, manipulation, and management.
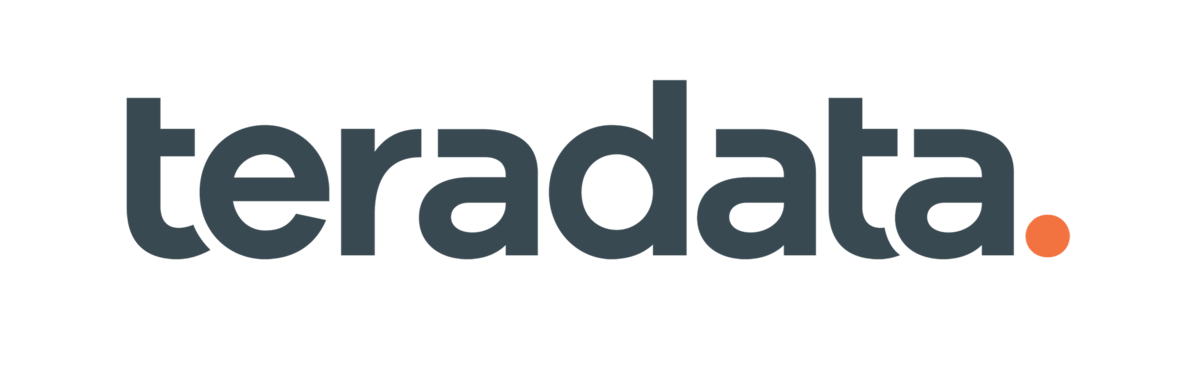
Some of the key features of Teradata are:
1) Massively Parallel Processing (MPP) architecture — Teradata’s MPP architecture ensures high availability and load balancing by distributing both data and queries across nodes. Teradata uses a unique hashing algorithm to distribute data evenly across AMPs (Access Module Processors), reducing hotspots and improving performance.
2) Workload management — Teradata’s workload management features enable the platform to optimize system resources by monitoring and controlling query workloads.
3) Data federation capabilities — Teradata supports querying across disparate data sources without the need for data movement. Teradata also supports foreign tables, which allow users to access and query data stored outside of Teradata, such as in Amazon S3, Azure Blob Storage, and Google Cloud storage, as if they were regular tables in the Teradata DBs.
4) Shared Nothing Architecture — Each component in Teradata works independently.
5) Connectivity — Teradata provides extensive connectivity options to mainframes, network-attached systems, and cloud platforms
6) Advanced analytics functions — Teradata provides advanced analytics functions that enable users to perform complex and sophisticated analyses of the data.
7) Various programming language support — Teradata also supports a wide array of programming languages, such as Python, C++, C, Java, Ruby, R, Perl, Cobol, and PL/I, to interact with the database and perform advanced analytics.
8) Linear Scalability — Teradata systems are highly scalable. They can scale up to 2048 Nodes.
9) Fault Tolerance — Teradata has many functions and mechanisms to handle fault tolerance. It has the protection mechanism against both hardware and software failure. Features like hot standby nodes and dual BYNET networks help maintain system availability during failures.
10) Flexible pricing options — Teradata offers a variety of pricing options, including pay-as-you-go and reserved instances, supporting deployment across on-premises, hybrid, and cloud environments.
Teradata Tutorial in 3 Hours | Teradata Tutorial for beginners | Teradata complete training |
Teradata’s architecture is based on a Massively Parallel Processing (MPP) system that integrates storage and compute nodes in a single system, meaning that Teradata distributes data and queries across multiple nodes, each with its own processor, memory, and disk, to achieve high performance, throughput, and reliability.
Teradata can handle massive data volumes, support complex workloads, and provide enterprise-grade performance at scale.
Let's do a deep dive into the various components that make up Teradata's robust MPP architecture.
The architecture of Teradata consists of five main components: Teradata Nodes, Parsing Engine, BYNET, AMP, Vdisks and PDE. Here is the high-level architecture of the Teradata Node.
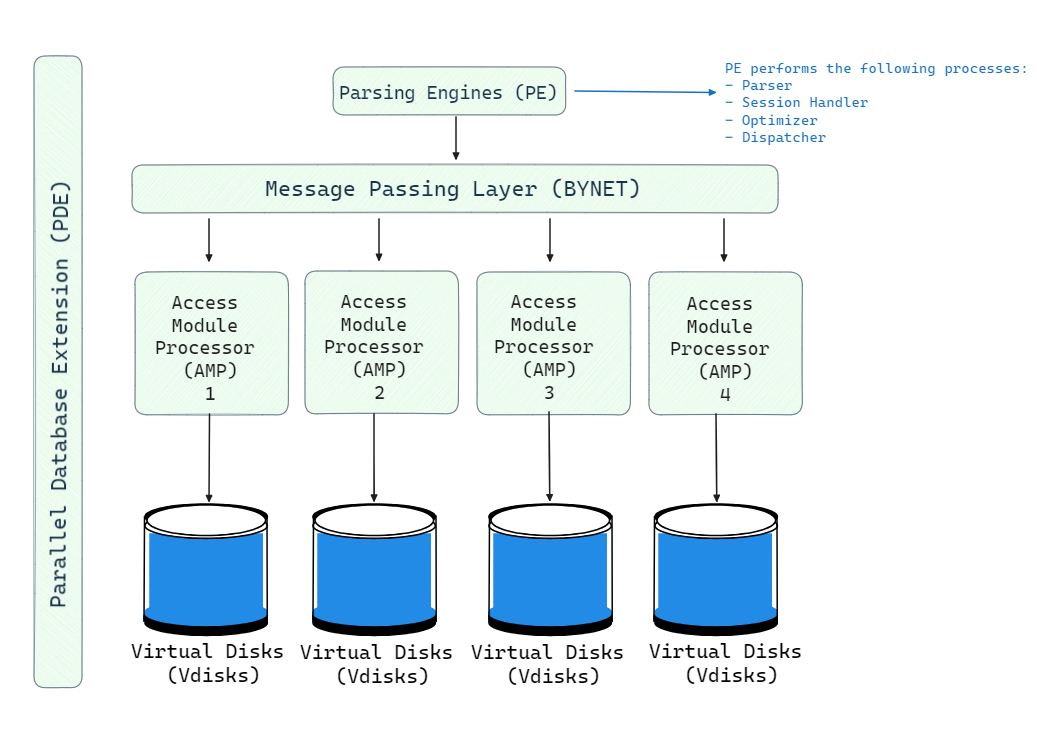
Here's a technical breakdown of the key components in Teradata's architecture:
1) Teradata Nodes — Each node operates independently with its own CPU, memory, and storage, forming the building blocks of Teradata's parallel architecture.
2) Parsing Engine (PE) — Receives SQL queries, checks privileges, and generates an optimized query plan. It then forwards the plan to BYNET for distribution to the AMPs.
3) BYNET (Message Passing Layer) — Acts as the communication layer between PEs and AMPs, ensuring efficient message passing and parallel query execution. Teradata employs dual BYNETs for fault tolerance.
4) Access Module Processors (AMPs) — AMPs store and process data in parallel, managing a portion of the database’s physical storage. They handle query execution by performing tasks like sorting, aggregation, and locking.
5) Vdisks (Virtual Disks) — AMPs manage Vdisks, which store the physical data. These virtual disks map to the underlying physical storage, and AMPs ensure efficient data retrieval by accessing their allocated portion of the database.
6) Parallel Database Extension (PDE) — PDE layer allows Teradata to interact with different operating systems, abstracting system-level details and enabling multi-OS support, including Linux and Windows.
Pros and Cons of Teradata
Pros of Teradata:
- Teradata offers strong performance for processing large datasets due to its parallel processing architecture.
- Teradata can scale to handle terabytes and petabytes of data.
- Seamless integration with popular ETL tools(like Informatica), as well as multiple different cloud platforms.
- Teradata Vantage supports multi-cloud and hybrid cloud setups, integrating well with public cloud providers like AWS, Azure, and Google Cloud.
Cons of Teradata:
- Teradata’s licensing and infra costs can be prohibitive, especially for smaller businesses.
- Initial setup and maintenance of Teradata systems can be complex
- Teradata’s offerings is less flexible compared to competitors like Snowflake.
- Due to its advanced features, Teradata can have a steep learning curve, especially for users not familiar with the platform
Teradata vs Snowflake—Which One Should You Opt For?
Teradata excels in handling complex, mixed workloads in enterprise environments, especially those that require on-premises or hybrid cloud setups. Its MPP architecture is highly optimized for large-scale queries and high concurrency.
Snowflake, in contrast, is a fully cloud-native platform designed for flexibility and simplicity. With independent scaling of storage and compute, Snowflake is ideal for organizations with dynamic workloads that need elasticity, especially in cloud-only environments. Snowflake’s near-zero maintenance and automatic scaling are key advantages for businesses that prioritize ease of use and scalability.
🔮 TL;DR: If you prioritize cloud-native architecture, elasticity, and minimal operational overhead, Snowflake is likely the better choice. However, for large enterprises requiring hybrid or on-premises deployments with extensive analytics capabilities and robust workload management, Teradata remains a very strong contender.
10) Firebolt
Firebolt is a modern cloud data warehouse designed to address the challenges faced by traditional analytics platforms, particularly in handling data-intensive applications that require high concurrency and low latency. It integrates various functionalities into a single platform, aiming to simplify the analytics stack while optimizing performance and cost.
Firebolt Product Showdown | Product Demo
Here are some key features of Firebolt:
1) High Concurrency and Low Latency — Firebolt is engineered to support numerous concurrent users and queries, providing sub-second response times crucial for customer-facing analytics and emerging data applications.
2) Flexible Infrastructure — Firebolt's architecture allows for tailored configurations based on workload demands, enabling vertical scaling (changing node types) and horizontal scaling (adding nodes or clusters) to meet varying performance needs.
3) Managed Service Simplicity — Firebolt simplifies resource provisioning with just-in-time scaling, layered security, and workload observability.
4) SQL Compatibility — Firebolt supports SQL queries and provides native handling of semi-structured data, streamlining the data processing lifecycle and accelerating development efforts.
5) Efficient Data Ingestion — Firebolt supports fast parallel ingestion processes that efficiently prepare large volumes of data for analysis. Firebolt maintains ACID compliance during updates and deletes, ensuring data integrity.
6) Optimized Storage Format — Data is stored in a proprietary columnar format known as Firebolt File Format (F3), which enhances query performance through reduced disk I/O and supports efficient compression for cost savings.
7) Sparse Indexes — These indexes significantly reduce the amount of data processed during queries by allowing for fine-grained data pruning, which lowers resource consumption and improves performance.
8) Aggregating Indexes — Designed for rapid access to fresh data, aggregating indexes pre-compute aggregations, allowing for quick query responses without compromising data freshness.
9) Integration with Data Lakes — Firebolt facilitates integration with external data lakes through direct read capabilities from common file formats like Parquet and CSV.
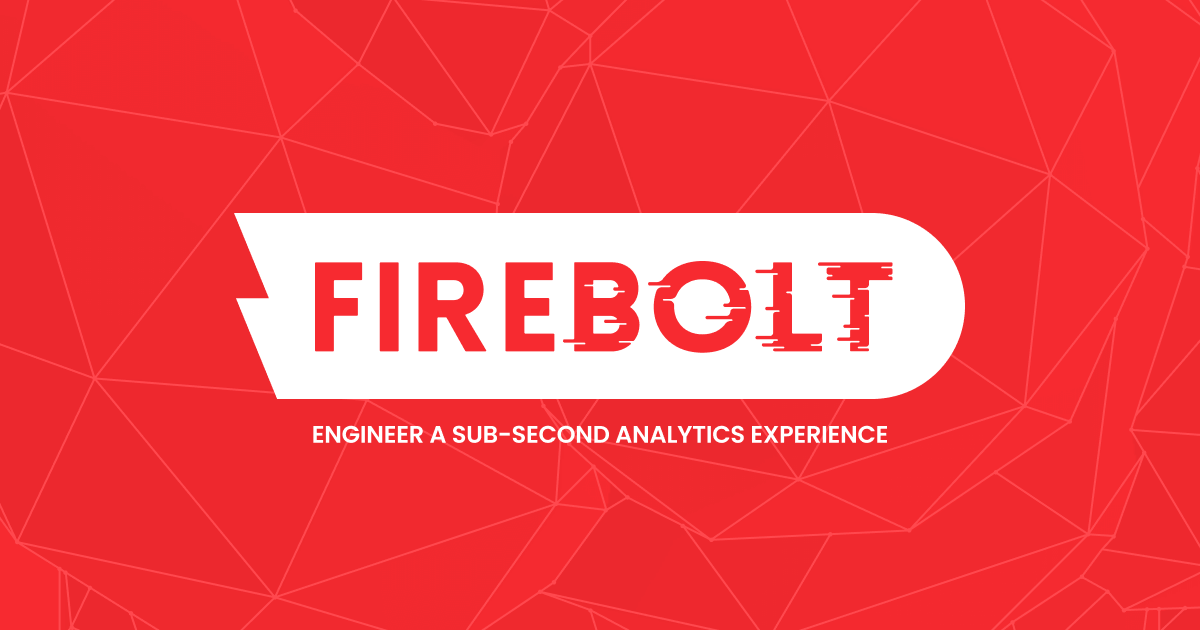
Pros and Cons of Firebolt
Pros of Firebolt:
- Designed for high concurrency and low latency, enabling sub-second query response times even with numerous concurrent users.
- Supports both vertical and horizontal scaling, allowing dynamic adjustments to compute resources based on workload demands.
- Enables direct access to external data lakes, facilitating federated queries across diverse data sources without requiring extensive ETL processes.
- Simplifies infrastructure management with automatic resource provisioning and monitoring capabilities.
- Provides advanced indexing options such as sparse and aggregating indexes that enhance query performance by minimizing unnecessary data scanning.
Cons of Firebolt:
- Some unique features may require time for teams to adapt effectively.
- Performance can be impacted by network latency, especially during cold reads from cloud storage.
- The integration ecosystem may be smaller compared to more established platforms like Snowflake.
- Actual costs can vary based on usage patterns, leading to potential budget fluctuations.
- As a newer platform, there may be concerns regarding stability and long-term support.
Firebolt vs Snowflake—Which One Should You Opt For?
Feature | Firebolt | Snowflake |
Architecture | Natively decoupled storage and compute architecture on AWS only. | Decoupled storage and compute architecture on AWS, Azure, and GCP. |
Separation of storage and compute | Yes | Yes |
Supported cloud infrastructure | AWS only | AWS, Azure, Google Cloud |
Tenancy Options | Isolated tenancy available; dedicated resources for compute and storage. | Multi-tenant metadata layer; isolated tenancy for compute & storage available via “VPS” tier. |
Control vs Abstraction of Compute | Configurable cluster size (1-128 nodes) with configurable compute types. | Configurable cluster size (1-128 nodes, 256 and 512 in preview); no control over compute types. |
Elasticity for Data Volumes | Granular cluster resize with node types and number of nodes. | Cluster resize without downtime; autoscaling up to 10 warehouses. |
Elasticity for Concurrency | A single engine can handle hundreds of concurrent queries; manual scaling for more engines. | 8 concurrent queries per warehouse by default; supports autoscaling for higher concurrency. |
Indexing | Primary indexes, aggregating indexes, join indexes. | “Search optimization service” indexes fields at an additional cost; materialized views available. |
Compute Tuning | Isolated control over the number of nodes with tuning options for CPU/RAM/SSD. | Fixed T-shirt size list abstracts underlying hardware properties; no tuning options available. |
Storage Format | Columnar, sorted & compressed & sparsely indexed storage (code named “F3”). | Columnar micro-partitioned & compressed storage. |
Table-Level Partitioning | User-defined table-level partitions are optional; data is automatically sorted and indexed. | Data is automatically divided into micro-partitions with pruning at the micro-partition level. |
Result Cache | Yes | Yes |
Warm Cache (SSD) | Yes, at indexed data-range level granularity. | Yes, at micro-partition level granularity. |
Support for Semi-structured Data | Yes, including Lambda expressions. | Yes |
Performance (Low-latency Dashboards) | Sub-second load times at TB scale. | Dozens of seconds load times at 100s of GB scale. |
Enterprise BI Features | Newer product with a narrower Enterprise DW feature set. | Mature and broad Enterprise DW feature set. |
11) Dremio
Dremio is an open source Data-as-a-Service (DaaS) platform designed to facilitate data access, analysis, and visualization from various sources in real time. It functions as a data lake engine and a self-service tool for data exploration, making it particularly suitable for organizations dealing with large and diverse datasets.
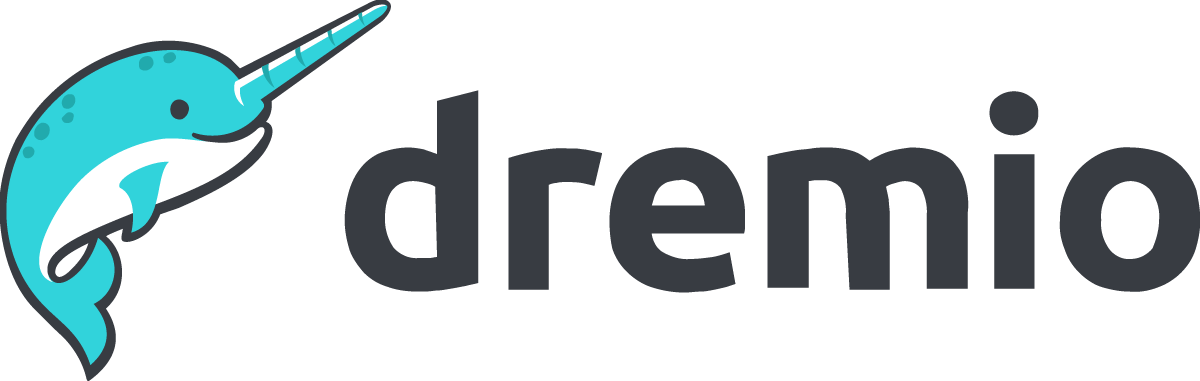
Here are some key Features of Dremio:
1) Dremio enables users to connect to various structured, semi-structured, and unstructured data sources without the need for physically moving or copying data.
2) Dremio utilizes Data Reflections and Apache Arrow for optimized query performance.
3) Dremio supports community-driven, open data formats like Apache Parquet, Apache Iceberg, and Apache Arrow. This ensures there’s no proprietary data lock-in, allowing organizations to retain control over their data with open, scalable solutions.
4) Dremio offers a user-friendly data catalog for efficient data discovery and self-service.
5) Dremio integrates with existing authentication systems such as LDAP, OAuth, and Kerberos, and provides features like fine-grained access controls, data encryption (at-rest and in-transit), and audit logs, ensuring regulatory compliance and data security.
6) Dremio supports flexible deployment on-premises or in the cloud, with options for elastic scaling using Kubernetes.
7) Dremio allows for optimized resource allocation across various workloads and users, enhancing performance and efficiency.
8) Dremio has native connectors for various data sources, including AWS S3, Azure Data Lake, and relational databases.
Pros and Cons of Dremio
Pros of Dremio:
- Dremio’s modern UI simplifies the data exploration process, making it accessible to non-technical users and reducing dependence on IT teams for basic queries.
- Dremio can perform high-speed, in-place queries on data stored in data lakes, reducing latency compared to traditional data warehousing solutions.
- Dremio can dramatically cut infra and ops costs by eliminating the requirement for ETL operations and querying data in place.
Cons of Dremio:
- Dremio may not support as many legacy data sources.
- Despite its user-friendly interface, some users find Dremio challenging to master due to the depth of its features.
- Dremio has limitations in handling certain data types, which might require workarounds or additional tools.
- Dremio can be resource-intensive when working with huge datasets, necessitating significant compute power to maintain performance.
Dremio vs Snowflake—A Head-to-Head Comparison
So, if you're trying to decide between Dremio vs Snowflake, it's essential to understand their core functionalities and how they align with your organization's data strategy.
Snowflake is a fully managed, cloud-native data warehouse known for its simplicity, scalability, and minimal administrative overhead. It automates many management tasks such as auto-scaling, data replication, and security, making it ideal for companies needing a highly performant solution for structured and semi-structured data. Snowflake’s architecture allows for seamless data sharing and advanced analytics without requiring users to manage infrastructure.
Dremio excels in environments where organizations need to query multiple data sources without ETL. Its Data Reflections feature improves query performance by pre-computing commonly accessed datasets and caching results. Dremio’s open-source nature and support for various deployment models (cloud or on-prem) provide flexibility and control. It’s a strong choice for organizations needing real-time analytics, cost-efficiency, and the ability to integrate with diverse data ecosystems.
🔮 TL;DR:
- Choose Snowflake if you need a managed, cloud-based data warehouse with seamless scalability, ease of use, and powerful analytics for structured data.
- Choose Dremio if your focus is on real-time, self-service analytics across diverse data sources, without ETL, with an emphasis on cost-efficiency and flexible deployment options.
12) Cloudera Data Platform
Cloudera is a prominent provider of enterprise data management and analytics solutions, offering a comprehensive platform known as the Cloudera Data Platform (CDP). CDP integrates a wide range of data management capabilities, including data engineering, data warehousing, machine learning, and operational data management, all with robust security, governance, and regulatory compliance in mind.
Cloudera's platform was born from the merger of Cloudera and Hortonworks, two pioneers in the Hadoop ecosystem, and it is designed for enterprises aiming to leverage large-scale data for actionable insights across various industries. CDP supports hybrid and multi-cloud environments, which gives organizations the flexibility to run workloads on-premises, across multiple public clouds (e.g., AWS, Azure, Google Cloud), or in a hybrid setup.
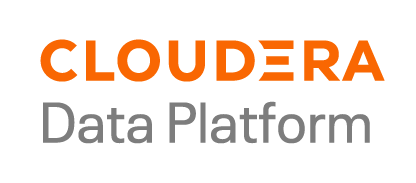
Here are some key features of Cloudera:
- Cloudera provides a unified platform that integrates data engineering, data warehousing, machine learning, and analytics, streamlining workflows and reducing data silos.
- CDP has built-in security features such as encryption at rest and in transit, role-based access control (RBAC), and comprehensive auditing and lineage tracking through Apache Atlas.
- Cloudera is designed for horizontal scalability, making it possible to expand infrastructure resources based on demand without downtime.
- Cloudera Data Platform (CDP) can be deployed on various cloud environments (AWS, Azure, Google Cloud) as well as on-premises, providing flexibility in data management strategies.
- Cloudera integrates tools for building, deploying, and scaling machine learning models using Cloudera Machine Learning (CML).
- Cloudera platform supports various Apache projects, including Apache Hadoop, Apache Spark, and Apache Kafka, allowing users to leverage existing tools and frameworks.
Watch this video for an in-depth exploration of the Cloudera platform.
Discover Cloudera Data Platform
Pros and Cons of Cloudera
Pros of Cloudera:
- Cloudera provides a full-stack solution for data engineering, data lakes, ML, and analytics.
- Strong focus on security and governance, making it suitable for regulated industries.
- Multi-cloud deployment options providing flexibility and scalability.
- Robust community support due to its integration with the Apache ecosystem.
Cons of Cloudera:
- Complexity in setup and management may require specialized knowledge and resources.
- Compared to cloud-native platforms like Snowflake, Cloudera’s operational complexity can drive higher costs in both infrastructure and staffing, particularly for smaller businesses.
- Finding skilled professionals with deep knowledge of Cloudera and its related technologies, such as HDFS, Impala, and Spark, can be challenging.
Snowflake vs Cloudera Data Platform—Which One Should You Opt For?
When comparing Snowflake vs Cloudera, it’s important to understand that they are designed for different workloads and use cases.
Snowflake is a cloud-native data platform that prioritizes ease of use, scalability, and performance. Its serverless architecture abstracts infrastructure management, allowing organizations to scale compute and storage independently. This makes Snowflake an ideal choice for organizations that need elastic, high-performance data warehousing without the need for deep infrastructure management expertise. Its columnar storage and query optimization capabilities are optimized for analytical workloads.
Cloudera, in contrast, provides a more flexible and customizable platform with a focus on managing both real-time and batch data pipelines. Its Hadoop-based architecture supports large-scale data lakes, distributed storage (HDFS), and diverse workloads, from ETL to machine learning and streaming analytics. This makes Cloudera better suited for organizations with complex data environments and those needing to manage structured, semi-structured, and unstructured data in an integrated platform.
🔮 TL;DR:
- Snowflake is ideal for organizations that prioritize simplicity, scalability, and performance in a cloud-only setup, with a strong focus on data warehousing and analytics.
- Cloudera is better suited for organizations needing extensive support for data lakes, real-time streaming, batch processing, and on-premises/hybrid cloud flexibility. While it is highly customizable, it also requires more technical expertise for management.
13) StarRocks
StarRocks iis a high-performance analytical database designed for real-time, large-scale analytics. It uses a Massively Parallel Processing (MPP) that allows for fast, sub-second query performance on complex data sets. The system is optimized for both Online Analytical Processing (OLAP) and real-time data analysis, enabling businesses to gain insights quickly and efficiently without having to pre-aggregate or denormalize data.
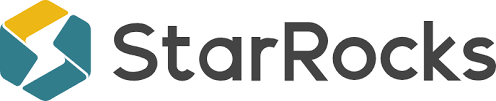
Here are some key features of StarRocks:
1) Massively Parallel Processing (MPP) — StarRocks distributes queries across multiple nodes to enhance performance and reduce execution time.
2) Columnar Storage — StarRocks stores data in a column format, optimizing for compression and retrieval speeds, which is especially beneficial for analytical workloads.
3) Real-Time Analytics — StarRocks allows immediate querying of data as it is ingested, supporting both batch and streaming data loads.
4) Synchronous Materialized Views — StarRocks supports real-time, automatically updated materialized views that accelerate query performance by reducing the need to reprocess data every time a query is run.
5) High-Concurrency Handling — StarRocks is capable of managing numerous concurrent queries efficiently due to its robust architecture.
6) Data Lake Integration — StarRocks can query data directly from various data lakes (e.g., Apache Hive, Delta Lake) without needing data migration.
7) Flexible Data Modeling — StarRocks supports multiple schema designs allowing for versatile data organization.
Pros and Cons of StarRocks
Pros:
- Offers sub-second query response times even with large datasets due to its vectorized execution engine and efficient storage mechanisms.
- Easily scales horizontally by adding more backend nodes to accommodate growing data volumes without performance degradation.
- Provides immediate access to newly ingested data, making it ideal for applications requiring up-to-date information.
- Generally offers better price-performance ratios compared to some competitors like Snowflake, especially for large-scale deployments.
Cons:
- For users unfamiliar with OLAP systems or MPP databases, setting up and optimizing StarRocks for specific workloads might involve a learning curve.
- Certain advanced features and customizations may require additional community support or consulting for optimal implementation.
- StarRocks is powerful, but the resource requirements to achieve optimal performance—especially in real-time analytics—can be significant.
StarRocks vs Snowflake—Which One Should You Opt For?
Feature | StarRocks | Snowflake |
---|---|---|
Architecture | Optimized for real-time analytics | Cloud data warehouse |
Deployment | On-premises, Cloud, Hybrid | Multi-cloud support (AWS, Azure, GCP) |
License | Open Sourced (under Apache 2.0) | Closed Source |
Data Model | Supports multi-table materialized views | Single table for materialized views |
Materialized Views | Cost-effective for large-scale BI | Costly for heavy usage |
Cost Efficiency | Sub-second query response time | Variable query response depending on configuration |
Query Latency | Suitable for large datasets | Great for structured data |
Analytics | Better for real-time analytics | Strong support for structured and semi-structured data |
14) Imply (Apache Druid)
Imply is a real-time analytics platform built on top of Apache Druid, designed for high-performance, low-latency analytics on large datasets. It enables fast, scalable, and efficient querying of both real-time and historical data. Imply simplifies the deployment and management of Druid, offering both fully-managed and self-managed options, making it accessible for a variety of analytics applications.
Apache Druid in 5 Minutes
Here are some key features of Imply:
1) Real-Time Analytics — Imply enables the analysis of both streaming and historical data with high concurrency at any scale, supporting sub-second query response times even on trillions of rows.
2) Fully Managed Infrastructure — Imply provides a fully managed service called Imply Polaris that abstracts away the operational complexities of managing Apache Druid clusters.
3) High Concurrency — Imply, leveraging Druid's columnar storage and distributed architecture, can handle thousands of concurrent queries per second.
4) Simplified Data Operations — Provides advanced management software that simplifies cluster operations, making it easier to deploy and scale Druid without requiring deep expertise in Druid itself.
5) Flexible Deployment Options — Users can choose between a fully managed cloud service (Imply Polaris) or self-managed deployments, which can be deployed in on-premises environments, on private clouds, or in public clouds (e.g., AWS, GCP, Azure).
6) Cost Efficiency — Imply reduces the total cost of ownership (TCO) by optimizing infrastructure resources. Features like intelligent auto-scaling, tiered storage (hot/cold data separation), and efficient resource utilization help reduce hardware and operational costs. Imply claims a potential reduction of TCO by up to 50% compared to self-managed Druid deployments.
7) Integration with Streaming Data Sources — Apache Druid, at the core of Imply, natively integrates with streaming data sources such as Apache Kafka, Amazon Kinesis, and others.
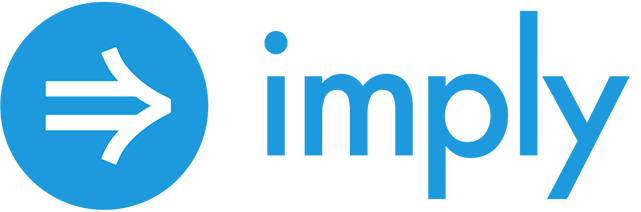
Pros and Cons of Imply
Pros of Imply:
- Delivers fast query responses with sub-second latency, essential for real-time analytics.
- Can easily scale to handle large volumes of data and high query loads without significant performance degradation.
- Imply abstracts much of the complexity of Apache Druid, making real-time analytics accessible even to teams without deep expertise in Druid.
- Efficient resource utilization and tiered storage reduce infrastructure costs compared to traditional DBs.
Cons of Imply:
- Some advanced features may still require a deeper understanding of Druid's architecture.
- While Imply offers flexibility, reliance on their managed services could lead to vendor lock-in.
- Although it supports SQL-like queries, some complex operations (like JOINs) may not perform as well compared to traditional SQL databases.
Snowflake vs Imply—Which One Should You Opt For?
The choice between Snowflake vs Imply depends on your specific data use case:
Snowflake is a cloud data platform optimized for data warehousing and batch analytics. It separates compute and storage, allowing for flexibility in scaling and cost management. However, its architecture is more suited for batch-oriented workflows where data is ingested periodically. While it can support streaming data ingestion via connectors, it typically incurs delays due to batch processing, making it less ideal for real-time analytics.
Imply, on the other hand, excels in real-time analytics. Built on Apache Druid, it’s optimized for high concurrency and low-latency queries. Imply natively supports real-time ingestion from streaming sources like Kafka and Kinesis, allowing for immediate queryability of incoming data. This is crucial for applications that require instant insights, such as monitoring dashboards, fraud detection systems, or time-series analysis.
🔮 TL;DR:
- Choose Imply if you need real-time analytics with immediate query capabilities and high concurrency.
- Choose Snowflake if your primary focus is batch processing, with a flexible pricing model for data warehousing and less frequent queries.
15) Clickhouse
ClickHouse is a high-performance, open source, column-oriented database management system (DBMS) designed for Online Analytical Processing (OLAP). Initially developed by Yandex, ClickHouse is widely used for processing and analyzing large volumes of data with SQL-like queries.
ClickHouse is particularly well-suited for scenarios requiring real-time analytics on big datasets, such as web analytics, advertising technology, and financial data analysis.

Here are some key Features of Clickhouse:
- ClickHouse stores data in a column-oriented format, which is highly efficient for analytical queries that typically access only a subset of columns in a table.
- ClickHouse processes data as it arrives without requiring extensive Extract, Transform, Load (ETL) processes. It supports near-instant query responses, making it ideal for applications needing real-time insights.
- ClickHouse is designed to scale horizontally, allowing users to add more servers to handle increasing data volumes and query loads.
- ClickHouse also supports replication and sharding for improved fault tolerance and load balancing.
- ClickHouse offers a familiar SQL-like syntax, making it easy for developers and analysts to adopt and integrate into existing workflows. However, it lacks support for full ACID transactions.
- ClickHouse utilizes various compression algorithms to reduce storage requirements and improve performance.
What Is ClickHouse?
Pros and Cons of Clickhouse
Pros of Clickhouse:
- ClickHouse is optimized for fast, large-scale analytical queries.
- ClickHouse's innovative compression techniques reduce storage requirements, allowing users to store petabytes of data without incurring prohibitive fees.
- ClickHouse’s distributed architecture supports seamless horizontal scaling, which is essential for handling growing datasets and workloads.
- ClickHouse provides real-time analytics capabilities, which is especially valuable in time-sensitive applications like monitoring, fraud detection, or financial trading systems.
- ClickHouse's SQL-like language enables easy adoption and integration with existing tools such as Apache Kafka, Grafana, and others.
Cons of Clickhouse:
- ClickHouse lacks full ACID transaction support, making it less suitable for use cases that require complex transactions or frequent updates
- Relatively new technology compared to Snowflake, with a smaller ecosystem and community.
- ClickHouse may require more CPU, memory, and I/O resources to perform optimally in certain high-performance scenarios.
Clickhouse vs Snowflake—Which One Should You Opt For?
Both ClickHouse and Snowflake are powerful tools for data processing, but they serve different purposes and excel in distinct areas. Here's a quick comparision:
Feature | ClickHouse | Snowflake |
---|---|---|
Primary Use Case | Optimized for real-time analytics and low-latency OLAP queries. | Designed for data warehousing, batch processing, and broader analytics workloads. |
Architecture | Tightly coupled storage and compute. Can be deployed on-premises or cloud. | Decoupled storage and compute. Fully cloud-based (AWS, Azure, GCP). |
Scaling | Horizontal scaling through manual provisioning of additional nodes. | Automatic vertical and horizontal scaling for both compute and storage. |
Storage Format | Columnar storage optimized for analytical queries and compression. | Hybrid (row-based for transactional, columnar for analytical queries). |
Data Ingestion | Supports flexible ingestion from various sources including CSV, JSON, and streaming. | Ingestion primarily through third-party tools or cloud services (e.g., S3, Fivetran). |
SQL Compatibility | Partial SQL support, lacking some complex functionality (e.g., full ANSI SQL). | Full ANSI SQL compliance, with support for semi-structured data (JSON, XML). |
Latency and Performance | Exceptionally fast query execution for real-time workloads. Requires manual tuning. | Great for large analytical workloads, with solid performance for long-running queries. |
Security Features | Supports encryption and basic user/role-based access control. | Advanced security with encryption, role-based access control (RBAC), and data masking. |
Management & Deployment | Requires manual tuning and management, suitable for experienced engineers. | Fully managed, with minimal manual management required. |
Cost Structure | Open-source, low infrastructure cost but requires manual scaling and optimization. | Subscription-based, pay-as-you-go pricing with scaling flexibility. |
Use Cases | Best for real-time analytics, log analysis, and high query performance needs. | Ideal for data warehousing, BI, and complex analytical workloads. |
Cloud Providers | Can run on any cloud or on-premise. | Fully cloud-based on AWS, Azure, and Google Cloud. |
🔮 TL;DR:
- Choose ClickHouse if you need real-time, high-performance analytics at a lower cost and can handle the operational complexity of managing a distributed system. It's best for fast, streaming data queries with low-latency requirements.
- Choose Snowflake if you require a cloud-native, highly scalable data warehouse for diverse analytical workloads, including structured and semi-structured data, with minimal operational overhead.
16) SingleStore (formerly MemSQL)
SingleStore, originally known as MemSQL, was founded in January 2011 by Eric Frenkiel, Nikita Shamgunov, and Adam Prout. It is an extremely powerful distributed database platform designed for handling real-time analytics and transactional workloads. It combines the speed of in-memory data processing with the scalability of distributed systems, making it well-suited for modern applications that require fast query processing and large-scale data handling.
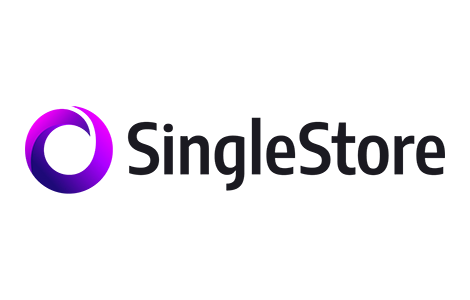
Here are some key Features of SingleStore:
1) In-Memory Rowstore and Disk-Backed Columnstore — This dual storage architecture allows you to manage both operational and analytical workloads efficiently.
2) Unified data processing — It combines transactional (OLTP) and analytical (OLAP) workloads in one platform, eliminating the need for separate databases. This simplifies infrastructure and improves efficiency.
3) SQL and MySQL Wire Protocol Compatibility — You can seamlessly integrate SingleStore into existing environments, leveraging familiar SQL syntax.
4) Code Generation for Enhanced Query Performance — This feature boosts the speed of SQL queries, ensuring quick data retrieval and processing.
Why SingleStore?
Pros and Cons of SingleStore
Pros of SingleStore:
- High-performance querying and data ingestion capabilities, handling millions of queries per second.
- Easily scales horizontally across distributed nodes to handle large datasets.
- Strong support for real-time data processing and analytics.
- Manages both transactional and analytical queries in one platform.
- Integrates with multiple external systems like Kafka, S3, and Spark
Cons of SingleStore:
- Can be expensive for larger deployments, especially as data storage needs grow.
- Although it uses standard SQL, the system's architecture can be complex for those unfamiliar with distributed databases.
- Requires careful management of the “bottomless” storage to avoid potential issues with large, unqueried datasets being stored indefinitely.
SingleStore vs Snowflake—Which One Should You Opt For?
Feature | SingleStore | Snowflake |
---|---|---|
Primary Use Case | Operational analytics, real-time analytics, machine learning, AI | Data warehousing, batch analytics, data lake applications |
Architecture | Distributed relational database with memory-optimized performance and separation of compute and storage | Cloud-native data warehouse with a clear separation of compute and storage |
Data Ingestion | Ingests structured and semi-structured data (JSON as a column type); real-time data via pipelines and streams | Batch ingestion (bulk loading) from various sources; supports JSON and XML as a VARIANT data type |
Query Performance | Fast, low-latency queries (50-1000ms when data resides in memory) | Optimized for large-scale batch analytics; seconds to minutes query time for petabyte-scale data |
Compute & Storage | Separation of compute and storage with cloud object storage; supports both transactional and analytical workloads | Separation of compute and storage; multiple virtual warehouses allow for isolation of workloads |
Data Formats | Supports rowstore and columnstore (universal storage) for transactional and analytical workloads | Columnar format stored in cloud object storage for analytical workloads |
Indexing | Supports manual indexing (e.g., skiplist, hash, full-text, and geospatial indexing) | No native indexing; relies on columnar format for performance optimization |
Scalability | Horizontal scaling via distributed architecture; optimized for real-time applications | High scalability with virtual warehouses that can scale up or down based on workload demands |
Security & Compliance | Encryption at rest and in transit; role-based access control; GDPR, SOC 2, HIPAA certifications | Encryption, dynamic data masking, role-based access control; supports ISO 27001, SOC 2, GDPR, HIPAA |
Streaming Capabilities | Real-time data processing via memory; lower data latency | Batch-based ingestion with Snowpipe; typically minutes delay for streaming |
Developer Tools | APIs, MySQL compatibility, JDBC drivers, integration with Python and MariaDB | Supports SQL APIs, UDFs (JavaScript, Python), and integrations with modern BI tools |
Pricing | Tailored pricing based on compute and storage usage; considered more cost-effective for real-time analytics | Subscription-based, pay-for-usage model; can be more expensive for continuous workloads, but good for batch processing |
🔮 TL;DR:
- Choose Snowflake if you need batch analytics, large-scale data warehousing, and cloud-native infrastructure with excellent scalability for handling massive data volumes.
- Opt for SingleStore if your focus is on real-time analytics, low-latency queries, and handling both transactional and analytical workloads with a distributed, in-memory architecture.
Want to take Chaos Genius for a spin?
It takes less than 5 minutes.
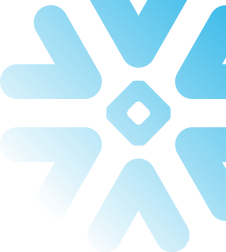
Further Reading
- Databricks vs Snowflake: 5 Key Features Compared
- Amazon Redshift vs Snowflake Comparison - 10 Key Differences
- Google BigQuery vs Snowflake Comparison: 7 Critical Factors
- Teradata vs Snowflake: 7 Features You Need to Know
- 22 Best DataOps Tools for Data Management and Observability
- What is Amazon Redshift?
- Amazon Redshift Concurrency Scaling
- Microsoft Azure Synapse Analytics
- Oracle Analytics Platform
- IBM Db2 Warehouse
- Discover Cloudera Data Platform
- Firebolt - The World's Fastest Cloud Data Warehouse
- What is Dremio?
- Overview of ClickHouse Architecture
- Introduction to SingleStore
Conclusion
And that's a wrap! Snowflake stands out in the cloud data warehousing space, but it's not the only option. Other competitors have their own strengths, and they might be a better fit for your specific needs. Knowing your options—and how they compare—can help you choose a platform that aligns with your overall strategy.
In this article, we have covered:
- Overview of Snowflake
- 10 Real Snowflake Competitors—Which One Suits Your Data Needs?
- Databricks vs Snowflake
- Google BigQuery vs Snowflake
- Amazon Redshift vs Snowflake
- Snowflake vs Azure Synapse Analytics
- Snowflake vs IBM Db2 Warehouse on Cloud
- Snowflake vs Oracle Analytics Platform
- Rockset vs Snowflake
- Apache Pinot vs Snowflake
- Teradata vs Snowflake
- Firebolt vs Snowflake
- Dremio vs Snowflake
- Snowflake vs Cloudera Data Platform
- StarRocks vs Snowflake
- Snowflake vs Imply
- Clickhouse vs Snowflake
- SingleStore vs Snowflake
… and so much more!
FAQs
What are the key competitors to Snowflake?
Competitors include Databricks, Google BigQuery, Amazon Redshift, Microsoft Azure Synapse, Teradata, and IBM Db2, among others.
How does Databricks compare to Snowflake?
Databricks offers a unified platform for data lakes and data warehouses, focusing heavily on machine learning and AI, whereas Snowflake excels in pure cloud data warehousing.
What are the strengths of Google BigQuery compared to Snowflake
BigQuery offers seamless integration with Google Cloud services, real-time analytics, and serverless architecture for efficient scaling.
How does Amazon Redshift stack up against Snowflake?
Redshift provides deep integration with AWS services and offers flexible pricing models, but Snowflake is often praised for its ease of use and performance with semi-structured data.
Which Snowflake competitor is known for its real-time analytics capabilities?
Apache Druid and Imply excel in real-time analytics, offering low-latency query performance for user-facing analytics applications.
What is Microsoft Azure Synapse’s advantage over Snowflake?
Azure Synapse integrates deeply with the entire Azure ecosystem, combining big data and data warehousing capabilities in one platform.
How does IBM Db2 compare to Snowflake?
IBM Db2 provides robust enterprise solutions with strong security, but it is generally more expensive and less flexible than Snowflake.
How does Dremio differ from Snowflake?
Dremio focuses on accelerating queries directly on data lakes, eliminating the need for data copies and providing a cost-efficient alternative to Snowflake for lakehouse architecture.
Is ClickHouse a viable alternative to Snowflake for high-speed analytics?
Yes, ClickHouse offers real-time analytical reporting with exceptional performance, especially for large-scale data processing.
Which competitor offers open source solutions?
Platforms like StarRocks and Apache Druid are open source, allowing for greater flexibility and cost efficiency compared to Snowflake.
What is the main trade-off between using Snowflake and Apache Druid?
Snowflake provides more flexibility for data warehousing, while Druid is optimized for real-time analytics with low-latency queries.
Who is Snowflake's biggest competitor?
Snowflake's primary competitor is Databricks. Both platforms offer cloud-based data warehousing and analytics solutions, but Databricks emphasizes machine learning and big data processing capabilities.
Are Snowflake and AWS competitors?
Snowflake operates on AWS but does not directly compete with it. Instead, it complements AWS by providing data warehousing capabilities that can be deployed on AWS infrastructure.
What is the Microsoft Snowflake equivalent?
Microsoft equivalent of Snowflake is Azure Synapse Analytics, which combines data warehousing and big data analytics services
Is Snowflake in demand?
Yes, Snowflake continues to be in very high demand, driven by the growing need for cloud-based data analytics and storage solutions among businesses.
Who are Snowflake's biggest clients?
Major clients of Snowflake include Netflix, Adobe, DoorDash, and Square, among others, which leverage its data warehousing and analytics capabilities
What is Google's equivalent to Snowflake?
Google BigQuery is considered the Google equivalent to Snowflake, providing a serverless data warehouse for analytics.
What makes Snowflake so unique?
Snowflake's unique features include its architecture that separates storage and compute, allowing for flexible scaling, as well as its ability to handle structured and semi-structured data seamlessly.