We're living in a time where data is everything—it's the heartbeat of modern business and innovation. Data drives decisions, fuels AI, and powers the technologies that shape our world. Companies that manage their data well have a huge advantage over those that don’t. But as data grows and systems get more complex, making sure the reliability and quality of this precious resource gets harder and harder. Bad data means bad insights, bad decisions and costly mistakes. A single data error can cost millions in revenue, damage customer relationships or get you fined by the regulator. That’s where Data Observability comes in.
In this article, we will deep dive into the concept of Data Observability, its key pillars, components, use cases, benefits, challenges, and the tools that support its implementation.
What is Data Observability?
Data Observability means an organization's ability to fully monitor, understand, and improve the health, quality, and performance of data. This is across its entire lifecycle within their systems. It includes the use of automated monitoring, anomaly detection, root cause analysis, data lineage tracking, and data quality metrics to actively identify, diagnose, resolve, and prevent data issues.
Data Observability is crucial as businesses increasingly rely on data to drive decisions yet often struggle with issues like data downtime and hidden "data debt" due to underlying problems.
Here are 5 reasons why Data Observability matters:
- Data Accuracy and Reliability: Ensuring the precision and trustworthiness of data.
- Expedited Root Cause Identification: Promptly determining the underlying causes of data-related issues.
- Enhanced Governance and Compliance: Ensuring adherence to regulatory requirements and maintaining data security.
- Seamless Data Transmission: Guaranteeing the uninterrupted flow of data through pipelines.
- Increased Trust and Confidence: Building trust in the data for decision-making processes.
Key pillars of Data Observability
Data Observability, as outlined by Bar Moses, rests on five key pillars—Freshness, Quality, Volume, schema, and lineage—that together enable organizations to gain valuable insights into the reliability and health of their data.
- Freshness: How up-to-date is the data? Stale data leads to poor decision making.
- Quality: Is the data accurate and complete? Watch for things like missing values or outliers.
- Volume: Is the amount of data as expected? Sudden drops may indicate problems.
- Schema: Has the data structure changed unexpectedly? This often signals issues..
- Lineage: Where did the data come from, and where does it go? This traces data from start to finish.
Importance of Data Observability—Why It Matters
Data Observability is crucially important for modern data-driven organizations for several reasons.
First, it helps minimize costly data downtime by detecting inaccurate, false, incomplete, or unavailable data that can impact operations and decisions.
Second, it builds widespread trust in data across the organization by providing transparency into data health.
Third, it improves the productivity of data teams by reducing the time spent diagnosing downstream data issues through early detection and alerts.
Fourth, it ensures compliance with data regulations by quickly responding to anomalies.
On top of that, Data Observability provides a feedback loop to continuously improve data infrastructure and processes based on observed trends. It also facilitates collaboration between data producers and consumers through shared visibility.
As reliance on data grows across the enterprise, Data Observability helps efficiently scale data capabilities and prevents data issues from falling through the cracks. Ultimately, it future-proofs businesses as data becomes a core asset by providing the foundation needed to build trust and maximize value.
Benefits of Implementing Data Observability
1) Better Data
Real time detection and resolution of data issues means data stays accurate and clean. Data Observability reduces data quality problems so you don’t have to do reactive firefighting and can focus on the strategic stuff.
2) More Trust and Investment
Stakeholders will trust and invest in data initiatives when they can rely on the data. Data Observability builds trust in data by making it clean and that means more investment in data projects
3) Speed
Less time spent on finding and fixing data issues means more time for the data team to focus on proactive work and innovation.
Challenges and Best Practices
Challenges
Data Observability can be tough due to the number of data sources and pipelines. Integrating observability across different systems and getting full coverage requires planning and execution. And setting up anomaly detection models that don’t produce false positives while finding real issues can be hard.
Best Practices
To overcome these challenges, organizations should:
1) Define Clear SLAs: Define Service Level Agreements (SLAs) for data quality with specific metrics and ranges. This gives you a framework to monitor and respond to data issues
2) Implement Robust Anomaly Detection: Use advanced anomaly detection models that can adapt to different data patterns and minimize false positives. So alerts are meaningful and actionable.
3) Create a Culture of Continuous Monitoring: Encourage a proactive approach to Data Observability by monitoring data health and addressing issues before they become problems. Review and update observability practices regularly to keep up with changing data environments.
Popular Tools for Data Observability
When it comes to Data Observability, the right tools are key to monitoring, anomaly detection and optimizing your data infrastructure. Here are some of the popular tools for Data Observability:
1) Chaos Genius
Chaos Genius is a DataOps observability platform designed for managing and optimizing cloud data costs, especially for Snowflake and Databricks environments. Features of Chaos Genius are:
- Cost Allocation and Visibility: Detailed dashboards to monitor and analyze cloud data costs and identify areas to cut costs.
- Instance Rightsizing: Recommends optimal sizing and configurations for data warehouses and clusters to use resources efficiently.
- Query and Database Optimization: Insights and recommendations to tune queries and databases for performance and cost savings.
- Anomaly Detection: Identifies unusual patterns and deviations in data usage, so teams can quickly fix issues.
- Alerts and Reporting: Real-time alerts via email and Slack on any anomalous activity so you can respond to data issues fast.
Try it yourself for free!!
For Snowflake Observability:
Want to take Chaos Genius for a spin?
It takes less than 5 minutes.
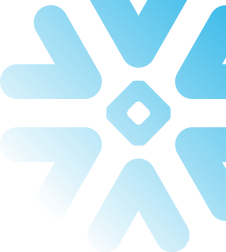
For Databricks Observability:
Want to take Chaos Genius for a spin?
It takes less than 5 minutes.
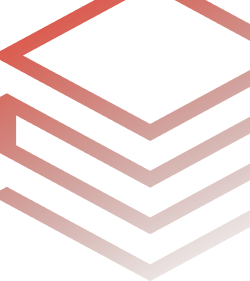
2) Monte Carlo Data
Monte Carlo Data is another Data Observability platform, with comprehensive tools to ensure data reliability and health across the data lifecycle. Features of Monte Carlo Data are:
- Automated Data Lineage: Tracks data flow across systems and pipelines, shows data transformations and dependencies.
- Anomaly Detection: Uses machine learning to detect and alert on data freshness, volume, distribution and schema anomalies.
- End-to-End Monitoring: Monitors data pipelines to detect and resolve issues before they become problems, reduces data downtime.
- Integration with Existing Tools: Integrates with data warehouses, ETL tools and business intelligence platforms so you can add Data Observability to your existing workflows.
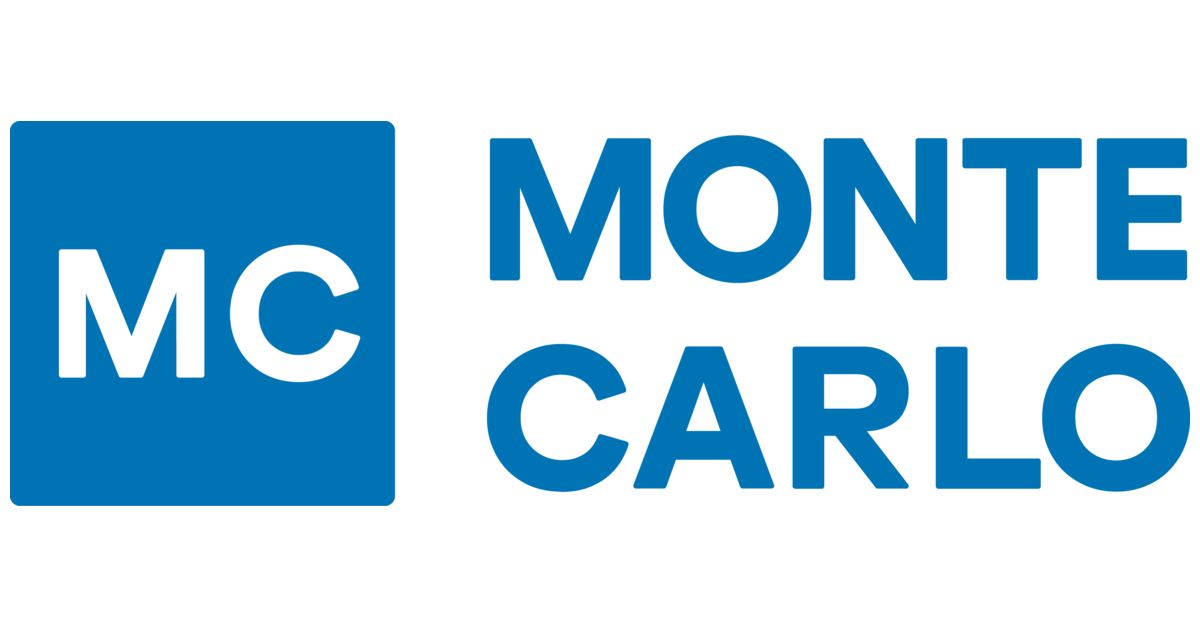
3) Acceldata
Acceldata is an enterprise grade Data Observability platform that provides robust tools to monitor data quality, pipeline performance and infrastructure. Key features of Acceldata are:
- Shift-Left Data Observability: Observability into the data pipeline and infrastructure from landing zone to consumption, reduces cost of bad data.
- Petabyte Scale Data Processing: Horizontal scalability and in-memory processing for high performance.
- AI-Assisted Anomaly Detection: AI algorithms to detect anomalies and provide fast insights to resolve data issues.
- Multi-Environment Support: Cloud, on-premises and hybrid data environments, single pane of glass for monitoring multiple data stacks.
- Enterprise Class Security: Data security with SOC2 Type 2 certification, RBAC and audit logs for large enterprise deployments.
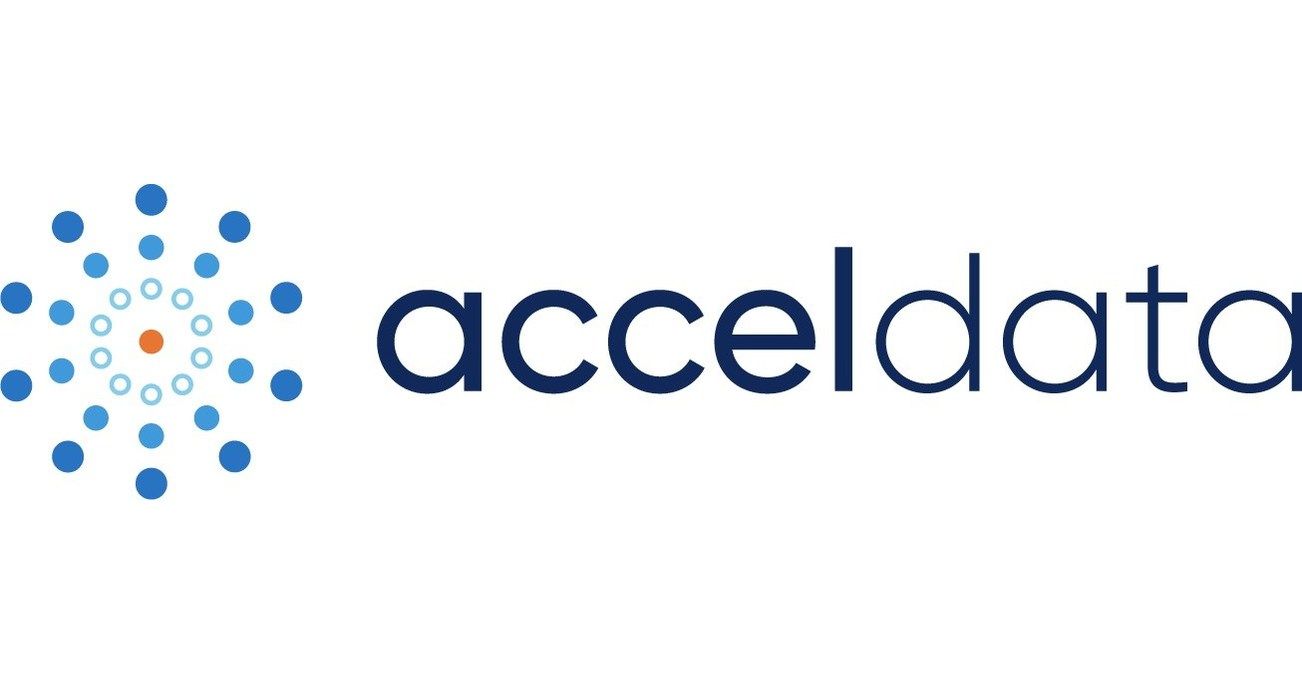
Check out this article to learn about the difference between Monte Carlo and Acceldata
Conclusion
Data Observability is key to data quality and reliability in data management. It gives you visibility into your data systems so you can catch and fix data issues in real-time, have accurate analytics, improve machine learning models and operate more efficiently. Despite the challenges of Data Observability, following best practices and using the right tools will get you to data health and reliability. As data becomes more and more important in decision-making and operations, Data Observability will be key to data driven success.
Data Observability is not just a technical requirement, but a strategic asset that keeps data a valuable asset for your organization. If you invest in Data Observability, you can build a solid foundation for your data projects.
FAQs
What is Data Observability?
Data Observability provides real-time visibility into data health across systems using telemetry like logs, metrics, and traces.
Why is Data Observability important?
Data Observability enables early detection and quick resolution of data issues before they cause downstream impacts.
What are the key capabilities of Data Observability?
Key capabilities include automated data monitoring, anomaly detection, root cause analysis, data lineage mapping, and data quality insights.
What are some benefits of Data Observability?
Benefits include preventing data downtime, improved decision making, faster incident response, and increased trust in data.
What tools enable Data Observability?
There are numerous tools available, but the main ones are Data Observability platforms such as Chaos Genius, Monte Carlo, and Acceldata. These platforms offer comprehensive Data Observability, including automated data health monitoring, which enables organizations to gain valuable insights and maximize the potential of their data.
What are the pillars of Data Observability?
The main pillars of Data Observability are Freshness, Quality, Volume, Schema and Lineage
How does Data Observability differ from monitoring?
Observability collects all data from all systems, while monitoring predetermines metrics to collect from individual systems.
What data sources does observability use?
Key data sources are application logs, database logs, metadata, performance metrics, and lineage.
How can you implement Data Observability?
Steps include inventorying use cases, aligning teams, implementing monitoring, optimizing response, creating custom alerts, and enabling prevention.
What are some challenges with Data Observability?
Challenges include data volumes, fragmented tools, organizational silos, and alert fatigue.