Dealing with data-heavy applications? ClickHouse is a name that probably keeps popping up. And for good reason—it's particularly good at fast query processing and high data compression. ClickHouse is a beast of a database. It’s fully open source, uses columnar storage, and is built specifically for OLAP (Online Analytical Processing). Its story starts back at Yandex, the search giant, which began developing it internally around 2008–2009 for its demanding Metrica web analytics platform. They needed something seriously fast. After proving its worth internally, Yandex open sourced ClickHouse in 2016 under the Apache 2.0 license. Since then, it's built a solid reputation for lightning-fast queries (props to it unique techniques like vectorized execution), impressive data compression (columnar storage helps a lot here), and the ability to scale out across many servers to handle massive volumes of information.
How popular is it? According to DB-Engines, ClickHouse is one of the fastest-growing high-performance, column-oriented SQL database systems for OLAP out there. As you can see in the graph below, ClickHouse is sitting at 31st place and climbing, with a score of 18.73 (as of April 2024).
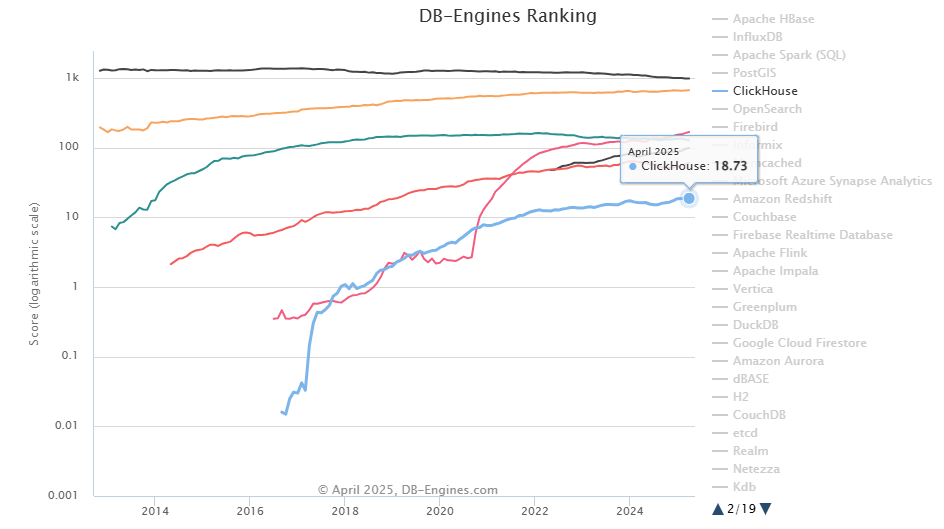
ClickHouse also pulled in a hefty $250M Series B funding round at a $2B valuation, a clear sign it’s got serious momentum. And it’s not just small startups using it. Big tech names like Yandex (where it all started), Cloudflare, Cisco, Tencent, Lyft, eBay, IBM, Uber, PayPal, and thousands more rely on ClickHouse for demanding analytics needs.
But even with all that going for it, ClickHouse isn’t perfect. It has its quirks and technical limitations. Its laser focus on OLAP speed means it often struggles with transactional (OLTP) workloads. Complex JOIN operations can sometimes be slower compared to other systems. And trying to fetch a single, specific row quickly (a point lookup)? Often painfully inefficient. That’s likely why you’re here—exploring ClickHouse alternatives, looking for a platform that might be a better technical fit, easier to manage, or simply more suited to your specific analytical needs.
In this article, we’ll break down what ClickHouse does best, its core architecture, its strengths, and those key limitations. Then, we’ll compare it to 10 notable ClickHouse alternatives across five categories: cloud data warehouses/platforms, traditional relational databases, real-time analytics or OLAP systems, time-series databases, and embedded analytics tools.
In a hurry? Jump directly to the comparisons for each ClickHouse alternative:
What Is Clickhouse Used For?
ClickHouse is built from the ground up as a distributed columnar database management system optimized for analytical workloads. It can be used to run fast analytical queries on huge datasets, especially when processing real-time logs, monitoring metrics, and analyzing time-series data. Many teams leverage ClickHouse when they need rapid aggregations on continuously incoming data, such as in monitoring systems or real-time dashboards.
How to Get Started with ClickHouse
What You Can Do With ClickHouse?
So, what do you use it for? It shines where you need to process and analyze huge amounts of data without twiddling your thumbs waiting for results. Here’s the quick rundown:
1) Real-Time Analytics — ClickHouse processes high-volume queries in near real-time. You run aggregations quickly over billions of rows, making it suitable for real-time dashboards.
2) Log and Event Data Analysis — ClickHouse handles log data and event streams well. Its design lets you sift through massive amounts of events quickly, which works well for troubleshooting and monitoring.
3) Time-Series Data Processing — While specialized time-series databases exist (some are covered as ClickHouse alternatives later), ClickHouse is very capable here due to its columnar nature and query speed.
4) High Throughput Ingestion — You can ingest hundreds of millions of rows per day. Its columnar storage minimizes the work your queries need to do, allowing you to handle vast amounts of data without lag.
….and many more!
ClickHouse Architecture Breakdown
Now, let’s break down ClickHouse’s architecture. ClickHouse splits its architecture into three distinct layers:
- Query Processing Engine
- Storage Layer (Table Engines)
- Integration Layer
Let's break down each layer.
1) ClickHouse Architecture Layer 1—Query Processing Layer
The Query processing layer is where ClickHouse handles your SQL queries. It optimizes and executes them quickly, even with massive datasets. Here’s how it works:
➥ Parsing — Your SQL query is converted into an Abstract Syntax Tree (AST).
➥ Optimization (Logical & Physical) — This is where ClickHouse gets clever.
- Logical — The AST becomes a logical plan. ClickHouse applies rules here like pushing filters down closer to the data source, calculating constant expressions, rearranging conditions for efficiency.
- Physical — The logical plan is then adapted based on how the data is actually stored.
➥ Execution Plan & Scheduling — ClickHouse creates a detailed step-by-step plan (a pipeline of operators) for executing the query. It figures out how to break this plan down to run parts in parallel across available CPU cores, and potentially across multiple servers if your data is sharded.
➥ Execution — The query is executed using a vectorized engine across multiple threads and nodes, leveraging SIMD instructions where possible.
Core Components & Techniques Making It Fast:
➥ Vectorized Execution
ClickHouse processes data in batches (vectors) of column values rather than row by row. Due to this vectorized execution, it significantly reduces overhead and improves CPU cache utilization. For computationally intensive operations, ClickHouse can use LLVM to compile optimized native machine code at runtime, which gets cached for potential reuse.
➥ Multi-Level Parallelism
Parallelism in ClickHouse operates at three levels:
- Data Elements (SIMD) — Uses Single Instruction, Multiple Data instructions to perform the same operation on multiple data points simultaneously within a single CPU cycle.
- Data Chunks (Multi-Core) — Processes different data chunks across multiple CPU cores, breaking the query plan into independent execution lanes.
- Table Shards (Multi-Node) — Orchestrates work across multiple ClickHouse servers for sharded tables, pushing work to nodes holding data shards.
➥ Advanced Query Optimizations
ClickHouse uses several optimization techniques:
- Query Optimization — Applies techniques like constant folding, filter pushdown, and common subexpression elimination.
- Data Pruning — Uses primary key and skipping indices to avoid reading unnecessary data.
- Hash Table Optimizations — Selects from over 30 specialized hash table implementations based on key types and cardinality.
- Join Execution — Supports multiple join algorithms including hash joins, sort-merge joins, and index joins.
➥ Handling Multiple Queries at Once
ClickHouse needs to juggle multiple users and queries. It manages this through:
- Concurrency Control — Dynamically adjusts how many threads a single query gets based on system load and limits you can configure.
- Memory Limits — Tracks memory usage per query, user, and server. If a query gets too greedy (e.g: for a huge aggregation), it can spill intermediate data to disk and use external algorithms instead of crashing the server.
- I/O Scheduling — Allows throttling disk access based on configured bandwidth limits or priorities.
2) ClickHouse Architecture Layer 2—Data Storage Layer
The storage layer forms the foundation of ClickHouse's performance. It revolves around table engines that determine how data is physically stored and accessed. When you CREATE TABLE, you choose an engine, and that choice dictates everything about how data lives:
- Physical storage format and location
- Indexing methods
- Replication and sharding capabilities
- Query performance characteristics
The key players here are the MergeTree* family of engines.
The MergeTree* Family Engine
If you're doing serious analytics in ClickHouse, you're almost certainly using a MergeTree variant (MergeTree, ReplacingMergeTree, AggregatingMergeTree, ReplicatedMergeTree, etc.). These engines are inspired by Log-Structured Merge Trees (LSM Trees) but have their own distinct implementation.
How is Data Stored?
When you insert data, ClickHouse writes it into immutable "parts" (directories containing column files). Each part contains a chunk of rows sorted according to the table's PRIMARY KEY.
Note: this primary key defines sort order, not uniqueness like in OLTP databases.
ClickHouse constantly runs background processes to merge smaller parts into larger, more optimized ones. Within each part directory, data for each column is stored in its own separate file.
Data within those column files is compressed (LZ4 by default, ZSTD often better). Because data in a column is typically similar, it compresses very well, saving storage space and often speeding up queries (less data to read from disk).
Column data is logically divided into "granules" (typically 8192 rows). ClickHouse doesn't index every row. Instead, for each part, it stores a small index file (primary.idx
) containing the primary key values for the first row of each granule. Since the data within the part is sorted by this key, ClickHouse can quickly scan this small index file (which often fits in memory) to determine which granules might contain the data matching your WHERE clause conditions on the primary key, skipping over potentially massive amounts of irrelevant data blocks.
Getting Data In (Ingestion)
Batching inserts is generally recommended. Each INSERT statement can create a small part, and merging too many tiny parts is inefficient. ClickHouse also supports asynchronous inserts where it buffers rows and writes parts periodically, which is better for high-frequency, small inserts.
Data Skipping
Besides the primary key index, you can define Skipping Indices on other columns or expressions. These are lightweight structures built over blocks of granules:
minmax
(Stores the minimum and maximum value for the expression within that block)set(N)
(Stores the unique values within the block (up to N values))Bloom_filter
(A probabilistic index good for checking existence).
ClickHouse can also use Projections, which are like hidden, pre-aggregated or specially sorted copies of your data. If a query matches a projection's definition, ClickHouse might read from the smaller, faster projection instead of the main table.
Transforming Data During Merges
The background merge process can do more than just combine parts:
- ReplacingMergeTree — During merges, keeps only the latest version of rows that share the same sorting key (based on an optional version column). Handles updates/deduplication.
- AggregatingMergeTree — Combines rows with the same sorting key by merging intermediate states of aggregate functions (e.g: summing sums, merging HyperLogLog states for unique counts). Often used with Materialized Views for incremental pre-aggregation.
- TTL (Time-To-Live) — Can automatically delete rows or even move entire old parts to different storage (like S3) based on time expressions during merges.
Handling Updates/Deletes
OLAP systems aren't built for frequent modifications, but ClickHouse offers various ways:
Mutations
— These are heavyweight background operations that rewrite entire data parts containing affected rows. They are asynchronous and non-atomic. Use them sparingly.Lightweight Deletes
— A faster option that marks rows as deleted using an internal bitmap. Queries automatically filter these marked rows. Physical removal happens later during merges. Faster to execute than mutations but might slightly slow down subsequent reads until merges clean things up.
Replication and Consistency:
- You typically use
Replicated*MergeTree
engines for fault tolerance. - Replication uses a multi-master approach coordinated via ClickHouse Keeper (a built-in Raft implementation, similar to ZooKeeper).
- Replicas fetch missing parts from each other and apply operations logged in Keeper to reach eventual consistency.
- ClickHouse provides snapshot isolation for reads (queries see a consistent view of data parts that existed when the query started). It's not fully ACID compliant in the traditional OLTP sense, particularly regarding durability guarantees on individual writes (it relies on the OS buffer cache by default, so a sudden power loss could lose the most recent unflushed data).
Other Table Engines:
While MergeTree is dominant, but others also do exist:
Sharding and Replication Layer (Built on Table Engines)
Sharding
— Large tables are often split across multiple nodes (shards). Each shard typically runs a local ReplicatedMergeTree table. Queries targeting the logical table use the Distributed table engine, which acts as a proxy, scattering the query to the shards and gathering results.Replication
— Using ReplicatedMergeTree within each shard guarantees data is copied across nodes for high availability.
3) ClickHouse Architecture Layer 3—Integration Layer
ClickHouse isn’t just about performance; it’s also about integration. The integration layer connects ClickHouse to external systems, enabling seamless data exchange.
So, how ClickHouse handles data integration? ClickHouse uses a pull-based approach, connecting directly to remote data sources to retrieve data. It supports over 50 integration table functions and engines, which includes:
- Relational Databases (MySQL, PostgreSQL, SQLite)
- Object Storage (AWS S3, Google Cloud Storage (GCS), Azure Blob Storage)
- Streaming Systems (Kafka)
- NoSQL/Other Systems (Hive, MongoDB, Redis)
- Data Lakes (Iceberg, Hudi, DeltaLake)
- Standard protocols like ODBC - via the ODBC table function.
On top of that, ClickHouse can read and write numerous formats like CSV, JSON, Parquet , Avro , ORC, Arrow, Protobuf .
The ClickHouse Query Lifecycle (Simplified):
To understand how these layers interact, let's follow a query through the system:
- Client sends SQL query.
- Server parses, analyzes, and optimizes the query.
- Plan identifies target parts/granules using indexes.
- Executor requests needed columns/ranges from storage.
- Data read, decompressed, processed via vectorized pipeline (parallelized).
- Results aggregated/merged (potentially shuffling data between nodes).
- Final result sent to client.
Check out the article to learn more in-depth about ClickHouse Architecture.
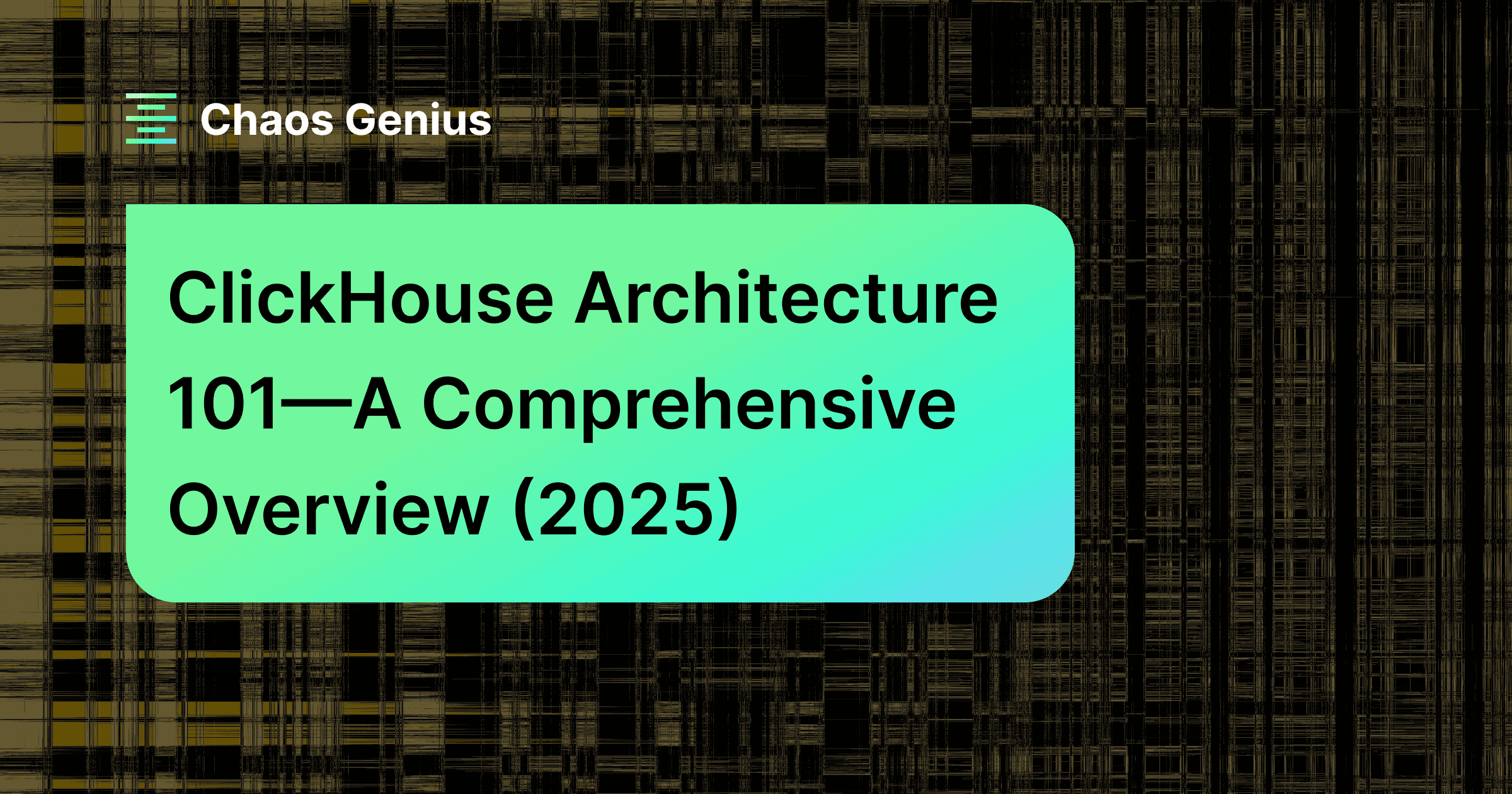
So, What Is Clickhouse Best For?
ClickHouse is best for real-time analytics on large datasets. Here are the features that make ClickHouse stand out:
➥ Columnar Storage. ClickHouse reads only the columns your query needs, cutting disk I/O and speeding up scans.
➥ MergeTree Engine Family. ClickHouse provides powerful table engines which isn't just one engine, but a family (MergeTree, ReplicatedMergeTree, AggregatingMergeTree, .....) that can handle high ingest rates and support features like deduplication and incremental aggregation.
➥ Vectorized Query Execution. ClickHouse processes data not one value at a time, but in batches (arrays or "vectors") of column values.
➥ Parallel & Distributed Processing. You can run ClickHouse on a single server or scale it out across a cluster. It splits queries across CPU cores and nodes, processing data in parallel.
➥ Data Compression. ClickHouse supports advanced compression algorithms like LZ4 and ZSTD. LZ4 for speed; ZSTD for higher compression.
➥ Real-Time Analytics. You can ingest data into ClickHouse in real-time with no locking. It’s designed for high-throughput inserts, so you can analyze fresh data as soon as it lands.
➥ Materialized Views. ClickHouse supports Materialized Views which allow you to pre-compute and store the results of aggregation queries.
➥ Pluggable External Tables and Integrations. ClickHouse can read from and write to many external systems (S3, HDFS, MySQL, PostgreSQL, Kafka, etc.,) and supports various data formats (CSV, JSON, Parquet, Avro, ORC, Arrow, Protobuf).
➥ Scalability (Horizontal). ClickHouse is designed to scale horizontally. You can add more nodes to your cluster as your data grows. Its distributed architecture means you’re not locked into a single box, and you can keep scaling without major headaches.
➥ SQL Support with Extensions. ClickHouse supports a rich SQL dialect with various extensions. You can run complex SQL queries on your data, like joins, aggregations, and window functions. Not only that, ClickHouse also allows you to create user-defined functions to extend its capabilities.
➥ Hardware Efficiency. ClickHouse is generally known for using server resources (CPU, RAM, I/O) efficiently to achieve its performance.
...and so much more!!
These features make ClickHouse a top performer for OLAP, but also define areas where a ClickHouse alternative might be needed.
What Are the Drawbacks of Clickhouse?
Okay, let's talk about the flip side. ClickHouse is fast, sure, but it's definitely not the right tool for every single job. Its strengths come with trade-offs, and ignoring them can lead to some serious headaches down the road.
➥ OLAP Focus (Not for OLTP). ClickHouse is primarily designed for OLAP (Online Analytical Processing), which means it excels at handling complex queries and large datasets. But, it’s not perfect for online transaction processing (OLTP) workloads. If your use case involves a lot of transactional operations, such as frequent updates, inserts, or deletes, ClickHouse might not be the best fit.
➥ Transactional Support? Not Here. ClickHouse doesn’t offer full ACID transactions. You can’t rely on it for workloads that need strict consistency or rollback support. If you’re used to databases like PostgreSQL or MySQL, you’ll miss this. It’s designed for OLAP, not OLTP. So, if your use case needs lots of updates, deletes, or row-level locking, you’ll hit a wall.
➥ Inefficiency for Point Lookups. Sparse indexing means ClickHouse isn’t efficient at fetching single rows by key. It’s blazing fast for scanning and aggregating millions of rows, but if you want to grab one record by ID, you’ll be waiting longer than you’d expect. It’s not built for transactional, row-based workloads.
➥ Joins? Only If You Must. Joins can be a real hassle. ClickHouse can handle them, but performance takes a hit when things get complex, especially with huge tables. You’ll often have to flatten your data—combine related tables into one—before loading it in. This adds pipeline complexity and can slow things down.
➥ Non-Standard SQL Dialect. You’ll find that ClickHouse’s SQL dialect is missing some features. No window functions (at least in earlier versions), and its join implementation is different from what you might expect if you’re coming from MySQL or PostgreSQL. Migrating existing queries can be a hassle.
➥ Cluster Management Can Be a Headache. Running ClickHouse at scale means managing clusters, replication, and sharding yourself. Cluster expansion often requires heavy, manual data rebalancing. If your team isn’t experienced with distributed systems, this can be a real challenge.
➥ Cloud Integration? Not Plug-and-Play. ClickHouse can be a bit tricky to work with when it comes to cloud-native tools and managed services. Integrating with ETL, data visualization, or reporting tools can take more work than with other databases that have broader ecosystem support.
These limitations aren't deal-breakers, but they're a trade-off since ClickHouse is built for specific use cases. Knowing these limitations will help you decide if it's the right fit or if one of the ClickHouse alternatives might better suit your needs.
10 True ClickHouse Alternatives: Which One Wins?
Okay, you see the trade-offs. Now, let's look at the ClickHouse alternatives.
ClickHouse is powerful, but the analytics landscape is broad. Let's explore alternatives, grouped by their primary function. Here we have categorize these alternatives to make comparisons clearer:
Let's dig in!
TL;DR:
If you don't want to read the entire post, here's a high-level summary of all ClickHouse alternatives based on several key factors.🔮 | Architecture | Primary Use Case | Storage Model | Deployment Model | Compute Scaling Model | Storage Scaling Model | Real-Time Ingestion Strength | SQL Dialect / Compatibility | JOIN Performance | ACID Compliance | OLTP Suitability | Open Source Core? | Cost Model(s) |
---|---|---|---|---|---|---|---|---|---|---|---|---|---|
ClickHouse | Columnar OLAP (Vectorized, MergeTree) | Real-time OLAP, Logs, Metrics, Events | Columnar (MergeTree) | Self-managed / Cloud Managed | Horizontal (Shards/Replicas) | Coupled / Independent | High | Extended SQL (Analytics Focus) | Good (Improving) | No (Atomic Operations) | No | Yes (Apache 2.0) | Infra/Ops (OSS), Usage (Cloud) |
Snowflake | Cloud DW (MPP, Shared Data) | General BI/DW, Data Sharing, Elastic Workloads | Columnar (Micro-partitions) | Cloud Managed (SaaS) | Horizontal (Elastic Warehouses) | Independent (Object Store) | Medium | High (Standard SQL) | Strong (MPP) | Yes | No | No | Usage (Compute Credits + Storage) |
Databricks | Lakehouse Platform (Spark/Photon) | Unified ETL, Streaming, BI, ML/AI | Columnar (Delta Lake/Parquet) | Cloud Managed (PaaS) | Horizontal (Spark Clusters) | Independent (Object Store) | High | High (Standard SQL + Spark APIs) | Strong (Spark SQL) | Yes (via Delta Lake) | No | Yes (Spark, Delta, etc.) | Usage (Compute DBUs + Storage) |
Google BigQuery | Cloud DW (Serverless MPP, Dremel) | General BI/DW, Serverless Analytics, ML | Columnar (Capacitor) | Cloud Managed (Serverless) | Horizontal (Auto Slots) | Independent (Object Store) | High | High (Standard SQL) | Strong (MPP) | Yes | No | No | Usage (Scan/Slots + Storage) |
Amazon Redshift | Cloud DW (MPP, RA3/Serverless Focus) | General BI/DW (AWS Focused) | Columnar | Cloud Managed (PaaS) | Horizontal (Nodes/RPUs) | Coupled / Independent | Medium | High (PostgreSQL-like) | Strong (MPP, Tuned) | Yes | No | No | Usage (Nodes/RPUs + Storage) |
MySQL | RDBMS (Row-Store, InnoDB) | OLTP, Application Backend | Row (InnoDB B+Tree) | Self-managed / Cloud Managed | Vertical, Read Replicas | Coupled (Node Storage) | Low (for Analytics) | High (Standard SQL) | Strong (Relational) | Yes (InnoDB) | Yes | Yes (GPL) | Infra/Ops (OSS), Instance (Cloud) |
PostgreSQL | ORDBMS (Row-Store, MVCC) | OLTP, Complex Apps, GIS, JSON | Row (Heap Tables, B+Tree) | Self-managed / Cloud Managed | Vertical, Read Replicas | Coupled (Node Storage) | Low (for Analytics) | High (Standard SQL, Rich Types) | Strong (Relational) | Yes | Yes | Yes (PostgreSQL License) | Infra/Ops (OSS), Instance (Cloud) |
Apache Druid | Real-time OLAP (Microservices, External Deps) | Low-Latency Dashboards, Events, Metrics | Columnar (Segments, Bitmaps) | Self-managed / Cloud Managed | Horizontal (Node Types) | Independent (Deep Store) | High | Medium (Druid SQL, Improving) | Limited (Lookups Best) | No (Immutable Segments) | No | Yes (Apache 2.0) | Infra/Ops (OSS), Usage (Cloud) |
Apache Pinot | Real-time OLAP (Microservices, External Deps) | User-Facing Analytics (Lowest Latency), Upserts | Columnar (Segments, Pluggable Indices) | Self-managed / Cloud Managed | Horizontal (Node Types) | Independent (Deep Store) | High | Medium (Pinot SQL, Improving) | Limited (Lookups Best) | No (Segment Immutability / Upserts) | No | Yes (Apache 2.0) | Infra/Ops (OSS), Usage (Cloud) |
TimescaleDB | Time-Series DB (Postgres Extension) | Time-Series + Relational Analytics | Row (+ Columnar Compression) | Self-managed / Cloud Managed | Vertical (Postgres) + Multi-node | Coupled (Node Storage) | High | High (Standard PostgreSQL SQL) | Strong (Relational) | Yes | Yes (Postgres Core) | Yes (Apache 2.0 Core) | Infra/Ops (OSS), Usage (Cloud) |
DuckDB | In-Process OLAP Library | Local/Embedded Analytics, File Querying | Columnar | Embedded Library | Single-Node (Vertical) | Single File / In-Memory | N/A | High (PostgreSQL-like) | Good (Single Node) | Yes (In-Process) | N/A | Yes (MIT License) | Library (Free) |
☁️ ClickHouse Alternatives: Category 1—ClickHouse vs Cloud Data Warehouses / Platforms
These platforms are cloud-native, often feature separation of storage and compute, and provide managed services. They excel at diverse analytical workloads but differ significantly in their underlying design, performance characteristics, and cost models compared to ClickHouse.
ClickHouse Alternative 1—Snowflake
Snowflake is a widely adopted cloud-native data platform, delivered as a fully managed SaaS (Software-as-a-Service). It aims to provide a single service for data warehousing, data lakes, data engineering, and data science workloads, running transparently on AWS, Microsoft Azure, or Google Cloud Platform infrastructure.
What is Snowflake? - ClickHouse Alternatives - ClickHouse vs Snowflake
Snowflake's Architecture
Snowflake architecture is built on three main layers that work together but can scale independently. Snowflake architecture is different from older systems where storage and compute were tied together. It runs completely on public cloud infrastructure (like AWS, Azure, or GCP).
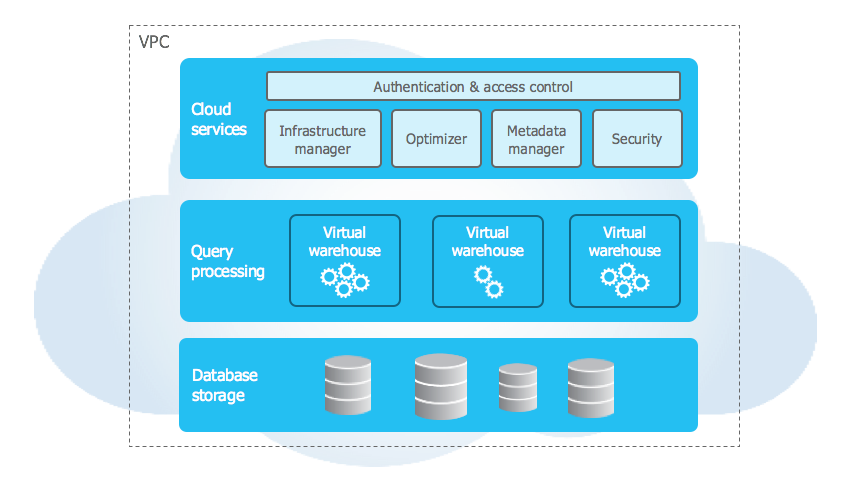
➥ Storage Layer
Snowflake’s storage layer leverages the underlying cloud provider's durable object storage (Amazon S3, Azure Blob Storage, Google Cloud Storage). When you load data, Snowflake reorganizes it into its internal optimized, compressed, columnar format. Data is stored in immutable files known as micro-partitions (typically 50-500MB uncompressed size, but stored compressed). Snowflake automatically manages all aspects of this storage: file organization, compression, encryption, and metadata management. Crucially, Snowflake collects rich metadata about the data within each micro-partition. This metadata is used extensively by the optimizer for data pruning, allowing queries to skip reading micro-partitions that cannot contain relevant data, significantly reducing scan times.
➥ Query Processing (Compute) Layer
Compute power in Snowflake is provided by Virtual Warehouses (VWs). These are independent MPP (Massively Parallel Processing) compute clusters (using underlying cloud instances like EC2 or Azure VMs) that you provision and manage via SQL commands or the UI. Each VW consists of multiple nodes with CPU, memory, and local SSD caching (for intermediate query results and temporary data). When you face a spike, you can scale up a warehouse or add multiple clusters for the same workload to handle concurrency—no manual sharding or capacity planning required.
➥ Cloud Services Layer
The cloud services layer acts as the "brain" of Snowflake, coordinating the entire platform. It's a collection of highly available, distributed services responsible for:
- Authentication and Access Control
- Infrastructure Management
- Metadata Management (catalog, micro-partition statistics)
- Query Parsing, Optimization, and Compilation
- Transaction Management
- Security Enforcement
- Client Connectors (JDBC, ODBC, ....)
This layer runs independently and scales automatically behind the scenes.
This three-layer architecture with decoupled storage and compute is fundamental to Snowflake's elasticity, concurrency handling, and managed service approach.
Snowflake's Key Features
Check out the article below for a comprehensive overview of Snowflake's extensive capabilities, including its architectural foundations, security measures, and key features.
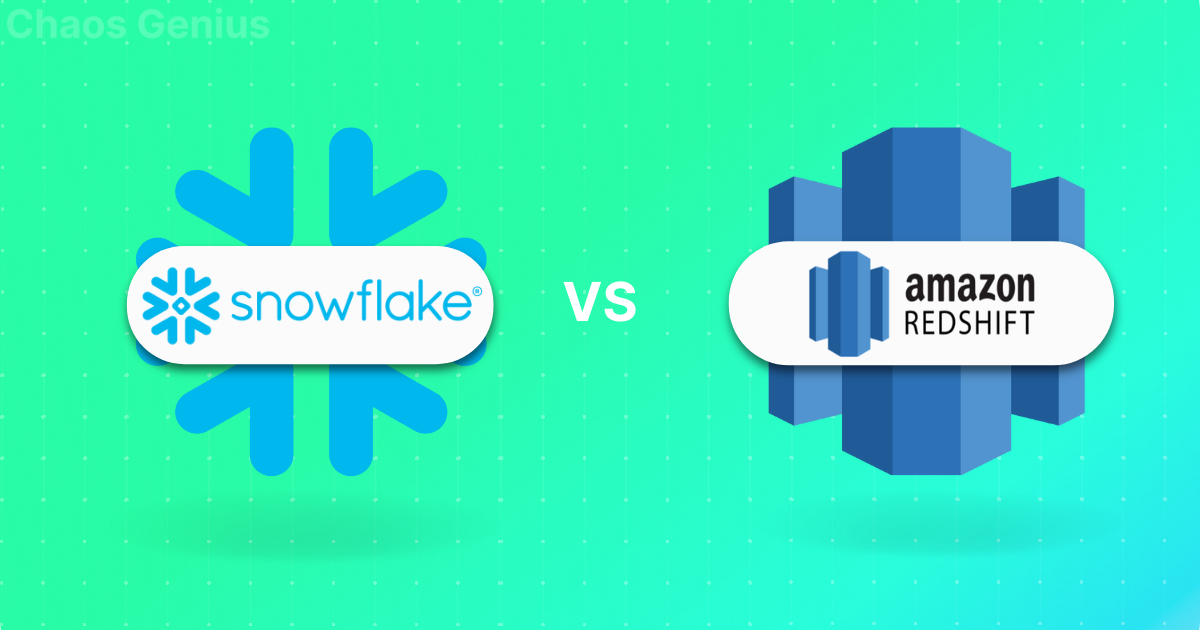
Pros and Cons of Snowflake:
Snowflake Pros:
- You pay separately for storage and compute. Scale your virtual warehouses up or down without touching data size—you only spin extra compute when you need it.
- It's a fully managed SaaS. No OS patches, no manual hardware configurations in a data center. You log in and start loading data.
- Zero‑copy cloning. Snap a copy of terabytes in seconds without doubling storage.
- Time travel and fail‑safe. Go back to data snapshots for up to 90 days.
- Automatic scaling and concurrency. Multi‑cluster warehouses spin up when you hit queuing, so your queries don’t block each other.
- Micro‑partitioning under the hood. Snowflake breaks data into small chunks and prunes non‑relevant ones automatically.
- Rich ecosystem and Snowpark. Build data pipelines in Python, Java or Scala right where your data sits.
- Strong security and compliance. End‑to‑end encryption, role‑based access and certifications like HIPAA and PCI‑DSS keep your data locked down.
- Data sharing and marketplace. Share live data with partners or use third‑party data sets.
Snowflake Cons:
- Cost surprises happen. If you forget to auto‑suspend warehouses or don’t track credit usage, bills can spike faster than you expect.
- Limited infrastructure control. You can’t tweak the OS or network stack; you have to play by Snowflake’s rules.
- Proprietary features and SQL extensions. Porting to another platform means you might need to rewrite your queries and UDFs.
- Data ingestion overhead. Continuous loads via Snowpipe avoid batch windows but add per‑file charges that can add up on lots of small files.
- Query caching can mask true performance. You might think a slow query is fast when you’re really hitting the cache, not raw compute.
- Metadata bloat. With heavy DDL or lots of micro‑partitions, your metadata store can grow, adding slight latency.
- Cross‑cloud replication limits. You can’t replicate freely between AWS, Azure and GCP in one go—you have to pick one per account.
Want to take Chaos Genius for a spin?
It takes less than 5 minutes.
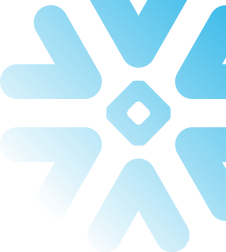
Is Clickhouse Better Than Snowflake?
Alright, let's break down the ClickHouse vs Snowflake comparison. They are both highly capable analytical databases optimized for different goals and operational models. ClickHouse often excels in raw processing speed for specific OLAP tasks, while Snowflake offers a broader, more managed, and elastic cloud data platform experience.
ClickHouse vs Snowflake—Architecture & Deployment Model
The architectural differences are fundamental to their operation, scalability, and cost characteristics.
🔮 | Snowflake | ClickHouse |
---|---|---|
Core Design | Cloud-native Data Warehouse Platform (SaaS) | Open Source Columnar Analytical Database |
Deployment | Fully managed SaaS on AWS, Azure, GCP | Flexible: Self-managed (on-prem, cloud VMs), Kubernetes, Managed Service (ClickHouse Cloud, others) |
Storage/Compute | Decoupled: Centralized object storage, separate compute (Virtual Warehouses). | Traditionally Coupled (Shared-Nothing): Nodes have own storage/compute. Decoupled Option: Can use object storage (S3, GCS, Azure Blob) via MergeTree settings (esp. in ClickHouse Cloud or newer self-managed). |
Scaling Model | Shared-Data: Compute scales independently, accessing shared storage. | Shared-Nothing (default): Scale by adding nodes (compute+storage). Shared-Disk (with object storage): Compute can scale somewhat independently of storage. |
Storage Format | Proprietary columnar format (Micro-partitions). | Open columnar format (MergeTree engine family); Can read/write Parquet, ORC, etc., directly or via table functions. |
Data Model | Relational; Strong semi-structured support (VARIANT , OBJECT , ARRAY ). |
Relational; Strong complex type support (Nested, Tuple, Map, Array, JSON object). |
Workload Isolation | High: Via separate Virtual Warehouses. | Lower (Self-hosted default): Shared resources on a cluster. Isolation often requires separate clusters or careful configuration. Higher (ClickHouse Cloud): Service-based isolation. |
Snowflake's architecture inherently enforces separation, leading to easier elasticity and managed scaling. ClickHouse offers flexibility (coupled or decoupled, self-managed or cloud) but requires more architectural decisions and potentially more effort (especially self-hosted) to achieve similar separation and isolation.
ClickHouse vs Snowflake—Performance & Query Execution
🔮 | Snowflake | ClickHouse |
---|---|---|
Query Speed (OLAP) | Fast for general DW tasks; Highly dependent on warehouse size, caching, and pruning. Seconds to minutes typical. | Extremely Fast: Optimized for aggregations, scans, time-series. Often sub-second/millisecond latency for well-tuned queries. Significantly faster for specific analytical patterns. |
Execution Engine | Traditional query execution pipeline; vectorized processing elements. | Vectorized Query Execution: Processes data in batches (vectors) for high CPU efficiency. |
Indexing & Pruning | Relies heavily on Micro-partition Metadata Pruning. Optional: Clustering Keys (sort data), Search Optimization Service (point lookups, paid). | Sparse Primary Index: Sorts data, enables efficient range scans/pruning. Data Skipping Indices: (minmax, set, bloom filter, etc.) prune granule blocks. Materialized Views. |
Query Optimization | Sophisticated cost-based optimizer; multi-layered caching (metadata, results, local disk). | Rule-based and basic cost-based elements; relies heavily on engine design (MergeTree), vectorized execution, indices, and Materialized Views. User hints/settings influential. |
Real-time Ingest | Near-real-time via Snowpipe (micro-batching, latency seconds to minutes). Batch COPY INTO for bulk loads. |
High-Throughput Writes: Designed for streaming inserts (though internal batching occurs). Can achieve very low ingestion latency (sub-second possible). |
Updates/Deletes | Background re-write of affected micro-partitions (metadata pointers updated). Asynchronous. | ALTER TABLE ... UPDATE/DELETE implemented as background mutations (rewriting parts). Optimized for append-heavy, less frequent mutations. Synchronous option exists but impacts performance. |
Compression | Automatic, efficient columnar compression. | Highly effective and tunable columnar compression (LZ4, ZSTD default, Delta, Gorilla, etc.). Often achieves higher compression ratios. |
ClickHouse is purpose-built for speed on analytical aggregations and scans, leveraging vectorized execution and efficient indexing for lower latency on specific OLAP queries. Snowflake offers strong, generally applicable performance across broader DW workloads, heavily optimized via metadata pruning and caching, with less manual tuning usually required.
ClickHouse vs Snowflake—Scalability & Concurrency
How easily can you handle more data or more users?
Both scale to petabytes, but via different mechanisms:
🔮 | Snowflake | ClickHouse |
---|---|---|
Compute Scaling | Easy & Fast: Vertical resize (change warehouse size) near-instant. Horizontal via Multi-cluster Warehouses (automatic or manual). | Self-hosted: Manual (add nodes, configure shards/replicas, rebalance data). ClickHouse Cloud: Simplified compute scaling (vertical/horizontal options available). |
Storage Scaling | Automatic & Transparent: Leverages underlying cloud object storage. | Self-hosted: Manual (add disks/nodes). Object Storage: Scales with cloud provider limits. ClickHouse Cloud: Managed, typically leveraging object storage. |
Scaling Architecture | Shared-Data: Compute nodes access central storage pool. | Shared-Nothing (default): Data partitioned/replicated across nodes. Shared-Disk (Object Storage): Compute nodes access shared object storage. |
Scaling Complexity | Low: Managed via UI/SQL; decoupling simplifies operations. | High (Self-hosted): Requires careful planning, configuration (sharding keys, replication), and operational effort (rebalancing). Medium (ClickHouse Cloud): Simplified by managed service. |
Query Concurrency | Scalable via Multi-cluster Warehouses: Each cluster handles a number of queries (default 8, adjustable). Add clusters for more concurrency. | High by Design: Nodes can handle many concurrent queries, limited by CPU/Memory/IO. Very high aggregate concurrency possible across a cluster. ClickHouse Cloud services are optimized for high concurrency. |
Snowflake provides a simpler, more elastic, and largely automated scaling experience, especially for compute and concurrency, due to its managed nature and decoupled architecture. ClickHouse (especially self-hosted) offers immense scaling potential but demands significantly more expertise for planning, configuration, and management.
ClickHouse vs Snowflake—Cost Model
🔮 | Snowflake | ClickHouse |
---|---|---|
Core Pricing | Usage-based: Compute (credits/second, warehouse size-dependent), Storage (TB/month), Serverless Features (Snowpipe, etc.). | Self-hosted: Free Open Source (pay infrastructure/operational costs). ClickHouse Cloud: Usage-based (vCPU, RAM, Storage, Data Transfer, specific features). |
Compute Cost | Billed per second (60s min) for active warehouses. Cost determined by size (XS, S,...). Can be paused (auto-suspend). | Self-hosted: Cost of hardware/VMs. ClickHouse Cloud: Billed based on compute resource usage time/size. Often lower cost per query/unit of performance for OLAP tasks. |
Storage Cost | Per TB/month on cloud object storage (includes Snowflake overhead/optimization). | Self-hosted: Cost of disks/object storage. ClickHouse Cloud: Per GB/month, often competitive due to potentially higher compression ratios. |
Extra Costs | Data transfer, Snowpipe (per file/notification), Search Optimization Service, Materialized Views (compute for refresh), Fail-safe storage. | Self-hosted: Operational overhead. ClickHouse Cloud: Data transfer (egress), backups, potentially specific features depending on provider plan. |
Cost Efficiency | Good for bursty/variable workloads due to auto-suspend. Can become expensive for sustained, high-compute tasks if not managed well. | Often More Cost-Effective for compute-intensive, sustained analytical workloads due to raw performance efficiency (less compute needed for same task). OSS offers lowest TCO if expertise exists. |
ClickHouse frequently offers better price-performance for high-throughput, low-latency analytical workloads, particularly when sustained compute is required. Snowflake's model provides flexibility and can be cost-effective for intermittent or unpredictable workloads, but requires vigilant cost management to prevent run-away compute expenses.
ClickHouse vs Snowflake—SQL Dialect, Ecosystem & Usability
🔮 | Snowflake | ClickHouse |
---|---|---|
SQL Compliance | High ANSI SQL compliance; familiar dialect for users from traditional RDBMS/DW backgrounds. | Powerful SQL dialect, but includes many unique functions and non-standard syntax extensions tailored for analytics. Learning curve can be steeper. |
Semi-structured | Excellent native support (VARIANT , OBJECT , ARRAY ) with specialized functions/operators for querying. |
Robust support (JSON , Map , Tuple , Array , Nested ) with many dedicated functions, though querying can feel less integrated than VARIANT . |
Ecosystem | Mature, extensive ecosystem; native connectors for most BI tools (Tableau, PowerBI), ETL/ELT tools, data catalogs. Snowpark for Python/Java/Scala. | Growing ecosystem; good JDBC/ODBC support, numerous third-party clients/integrations. Python clients popular. Official BI integrations improving. |
Mutability | Updates/Deletes handled via background micro-partition rewrites. Suited for batch modifications. | ALTER mutations are background operations optimized for infrequent, bulk changes. Not ideal for transactional (OLTP) updates. |
Community & Support | Enterprise support model from Snowflake Inc. Active user community forums. | Strong open source community. Commercial support available from ClickHouse Inc. and other vendors (Altinity). |
Useability | High ease-of-use due to managed service, familiar SQL, UI, and extensive documentation. | Steeper learning curve, especially for self-hosted (setup, tuning, cluster management). ClickHouse Cloud significantly improves usability. |
Snowflake generally offers a smoother learning curve, broader out-of-the-box tooling integration, and more standard SQL experience, appealing to a wider range of users. ClickHouse provides immense power and flexibility with its SQL extensions and data types but may require more specialized knowledge for optimal use and integration.
ClickHouse vs Snowflake—When Would You Pick One Over the Other?
Choose Snowflake if:
- You need a versatile, general-purpose cloud data platform for diverse analytical needs (BI, ELT, reporting, data science, data sharing).
- Ease of use, minimal operational overhead, and a fully managed SaaS experience are of utmost importance.
- Elasticity and independent scaling of compute and storage are critical requirements.
- You require robust workload isolation for different teams or applications.
- Your user base includes mixed technical/non-technical users relying heavily on standard BI tools.
- Workloads are variable or bursty, benefiting significantly from per-second billing and auto-suspension.
- Native secure data sharing capabilities are important for collaboration.
- You prefer a highly ANSI SQL-compliant environment.
- You heavily utilize semi-structured data and need flexible querying capabilities (
VARIANT
).
Choose ClickHouse if:
- Your primary driver is extreme query speed and low latency (sub-second) for OLAP, especially real-time dashboards and user-facing analytics.
- You are processing massive datasets (terabytes/petabytes) requiring rapid aggregations, filtering, and time-series analysis.
- Cost-efficiency at scale for high-performance, sustained analytical workloads is a major factor.
- You have high-throughput, append-heavy data streams (e.g: event logs, IoT data, metrics, observability).
- You value open source flexibility, deep customization potential (self-hosting), or need a managed service hyper-focused on analytical speed (ClickHouse Cloud).
- Very high query concurrency against raw data is essential.
- You have the technical expertise (or choose ClickHouse Cloud) to manage/tune its configuration for optimal performance.
So, wrapping up the ClickHouse vs Snowflake comparison: Snowflake offers a convenient, elastic, feature-rich, and broadly applicable managed cloud data platform perfect for general data warehousing, BI, and diverse user bases. ClickHouse provides superior performance and often better cost-efficiency for specific, demanding OLAP tasks, particularly in real-time scenarios, appealing strongly to use cases prioritizing speed, efficiency, and (optionally) open source control.
Continue reading...
ClickHouse Alternative 2—Databricks
Databricks is a Unified Data Analytics Platform, often referred to as a Lakehouse Platform. Founded in 2013 by the original creators of Apache Spark, Delta Lake and MlFlow, it aims to merge the benefits of data lakes (cost-effectiveness, flexibility for diverse data, scalability) with the performance, reliability, and governance features traditionally associated with data warehouses. It provides an integrated environment for data engineering, data science, machine learning (including Generative AI), and SQL analytics, available as a managed service on AWS, Azure, and GCP.
What is Databricks? - ClickHouse Alternatives - ClickHouse Competitors
Databricks Architecture—The Lakehouse Foundation
The Databricks platform architecture is logically divided into a Control Plane and a Compute Plane, built upon the customer's cloud infrastructure and storage.
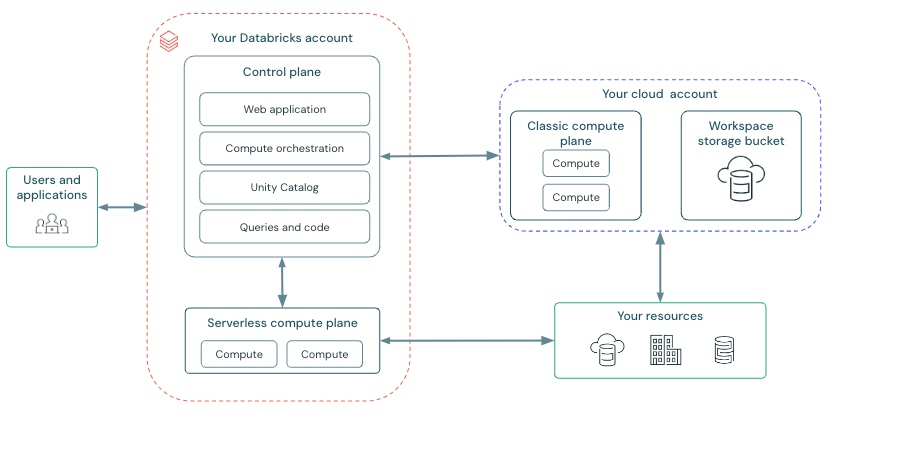
➥ Control Plane
The Control Plane is basically Databricks' command center. It runs in Databricks' own cloud account. It handles backend services like the web UI, notebook and job configurations, cluster management orchestration, REST APIs, and often metadata management, especially when using Unity Catalog. User code and business data are not processed or stored here.
➥ Compute Plane
The Compute Plane is where data processing actually occurs using Databricks compute resources (clusters). There are two deployment models:
- Classic Compute Plane — Compute resources (like VMs for Spark clusters or Databricks SQL warehouses) are provisioned and run directly within your cloud account. You have visibility into these resources in your cloud console.
- Serverless Compute Plane — Compute resources run within a secure environment in Databricks' cloud account within your region. Databricks manages the instance types, scaling, patching, and optimization, offering a more hands-off experience similar to other serverless offerings.
Regardless of the model, compute is fundamentally separated from storage.
Check out the article below to learn more in-depth about the capabilities and architecture behind Databricks.
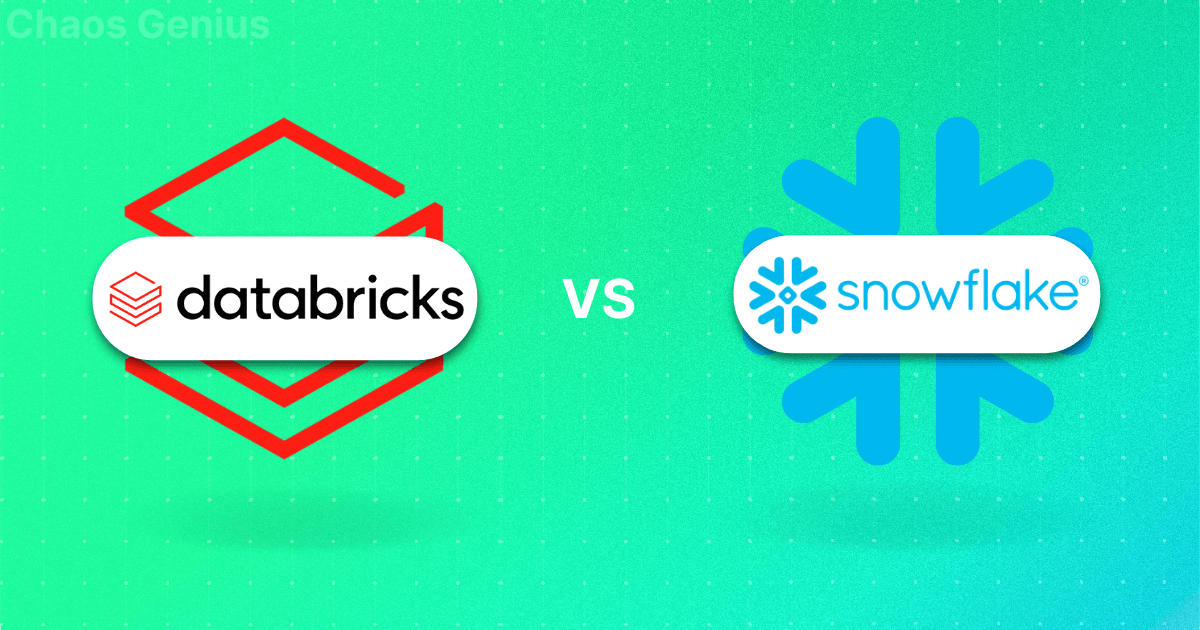
Databricks Key Features
Databricks provides a wide array of features integrated into its platform:
1) Unified Data Platform — Databricks provides a single interface for notebooks (supporting SQL, Python, Scala, R), job orchestration (Workflows), SQL querying (Databricks SQL), dashboarding, ML experimentation, and governance.
2) Optimized Apache Spark — Databricks Runtime (DBR) offers a managed, optimized version of Apache Spark with significant performance enhancements and caching mechanisms.
3) Delta Lake Integration — Databricks has native support for Delta Lake features (ACID, time travel, schema management, DML) for building reliable data lakes.
4) Databricks SQL (DSQL) — Databricks provides a dedicated SQL analytics experience with optimized SQL Warehouses (including Serverless options), query editor, dashboards, and alerts.
5) Photon Engine — Databricks comes with Photon Engine built in, which is a high-performance, C++ based vectorized execution engine accelerating SQL and DataFrame workloads.
6) MLflow for Machine Learning Lifecycle — MLflow is integrated within Databricks for tracking experiments, managing models, and automating deployments. You can track parameters, metrics, and artifacts, and even plug in with tools like OpenAI or Hugging Face for generative AI use cases.
7) Lakehouse Federation — You can query data across multiple sources, including Oracle and Hive Metastore, without moving it.
8) Collaboration Tools — Databricks provides:
- Interactive Notebooks
- Real-Time Notebook Sharing
- Version Control
9) Unity Catalog — Databricks is integrated with Unity Catalog which is centralized governance and access control for all your data assets, with REST API support for automation.
10) Delta Live Tables — Databricks comes with DLT integrated which automates and tracks your ETL pipelines with improved query tracking and maintenance features.
11) AutoML and LLM Fine-Tuning — Databricks has built-in AutoML for model selection and hyperparameter tuning, plus support for fine-tuning large language models (LLMs).
… and so much more.
Pros and Cons of Databricks:
Databricks Pros:
- Databricks handles massive data volumes and supports batch and streaming jobs.
- Databricks supports multiple languages, you can use Python, SQL, Scala, and R in the same notebook.
- Databricks supports real-time editing in notebooks which lets your team work together.
- Databricks can scale resources up or down based on workload.
- Mature ML/AI capabilities. Best-in-class integration for the end-to-end machine learning lifecycle.
- Databricks supports Delta Lake which adds ACID transactions, time travel, and schema enforcement to your data lake.
- Works with AWS, Azure, Google Cloud, and connects to popular BI (Business Intelligence) tools and data sources.
- Governance. Databricks Unity Catalog provides robust, centralized governance capabilities.
- Databricks provides extensive docs and a big community. You’ll find answers—eventually.
Databricks Cons:
- The breadth of features and underlying concepts (Spark, Delta Lake) can present a steeper learning curve than specialized tools. Configuration tuning can be very complex.
- Pricing is based on Databricks Units (DBUs) which vary by compute type, instance size, and cloud provider. Requires careful monitoring and optimization to control costs, especially for inefficient jobs or idle clusters.
- Photon is there to boosts performance... BUT optimal speed can still depend on cluster configuration, data layout (partitioning, Z-Ordering in Delta Lake), and query patterns. Not always as fast as specialized OLAP engines like ClickHouse for specific query types.
- Databricks Notebooks cap results at 10,000 rows or 2 MB. Analyzing big outputs? You’ll need to chunk or export your data.
- Large or messy notebooks become hard to manage. Searching for code snippets or outputs can get frustrating.
- Databricks is a managed service which restricts your access to underlying infrastructure. Fine-tuning hardware or networking isn’t really an option.
- Git integration is limited or clunky.
- Dependency on cloud providers. Outages or issues with your cloud provider (AWS, Azure, GCP) will impact Databricks availability and performance.
Save up to 50% on your Databricks spend in a few minutes!
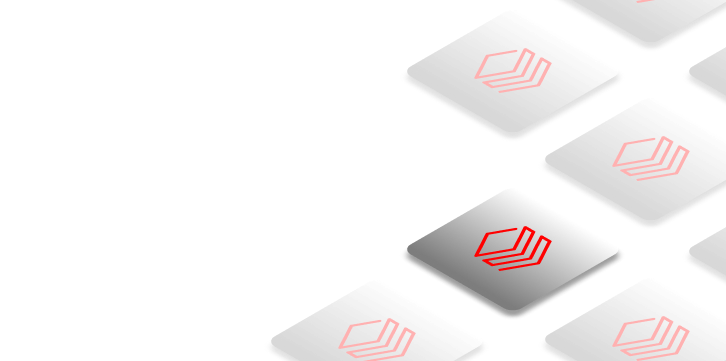
Alright, now let's break down the ClickHouse vs Databricks comparison.
ClickHouse vs Databricks: What's Best for You?
We'll dig into the technical stuff, but at a high level: ClickHouse prioritizes raw query speed for OLAP tasks, while Databricks focuses on integrating the entire data lifecycle (engineering, analytics, ML) on a flexible "Lakehouse" foundation.
ClickHouse vs Databricks—Architecture & Core Focus
🔮 | Databricks | ClickHouse |
---|---|---|
Core Engine | Apache Spark (distributed processing) enhanced by Photon (C++ vectorized execution engine). | Custom C++ vectorized execution engine optimized specifically for analytical (OLAP) queries. |
Primary Focus | Unified Platform: ETL/ELT, Streaming, SQL Analytics (BI), Data Science, ML/AI on a Lakehouse foundation. | High-performance OLAP Database: Real-time analytics, interactive dashboards, log/event analysis. |
Storage | Decoupled: Uses Delta Lake format (over Parquet) on user's cloud object storage (S3, ADLS, GCS). | Flexible: Traditionally coupled (shared-nothing nodes with local storage). Increasingly uses decoupled object storage (esp. ClickHouse Cloud or self-managed config). Stores data in proprietary columnar format (MergeTree family). |
Data Model | Relational schema via Delta Lake; Handles structured, semi-structured, and unstructured data effectively. | Primarily Columnar Relational DBMS. Strong support for structured and semi-structured (JSON, Map, Array, Nested). |
Deployment | Managed cloud service on AWS, Azure, GCP. | Flexible: Self-managed (OSS), Kubernetes, Managed Service (ClickHouse Cloud, others). |
Architecture | Lakehouse: Blends data lake flexibility (open formats, object storage) with DW reliability/performance. | Distributed Columnar Database: Optimized for fast analytical reads and aggregations. |
Databricks is architected as a comprehensive platform leveraging Spark and Delta Lake for diverse data workloads with inherent compute-storage separation. ClickHouse is a purpose-built analytical engine prioritizing query speed, offering more deployment flexibility but a narrower focus.
ClickHouse vs Databricks—Performance Profile
🔮 | Databricks | ClickHouse |
---|---|---|
Query Performance (OLAP) | Fast: Databricks SQL with Photon engine delivers competitive analytical query performance, leveraging Delta Lake optimizations (stats, Z-Ordering). | Extremely Fast: Often benchmarks faster for raw OLAP query speed (scans, aggregations, GROUP BY) due to specialized engine and storage format. Excels at low latency. |
Query Performance (ETL/ML) | Excellent: Leverages the full power of distributed Spark for complex transformations, large-scale data processing, and ML model training. | Not Designed For This: Typically used as a source/sink for external ETL/ML tools, not for executing complex pipelines or training models internally. |
Real-time Ingestion | Good: Spark Structured Streaming provides robust near-real-time ingestion into Delta Lake tables. Latency typically in seconds/minutes. | Excellent: Optimized for high-throughput, low-latency data ingestion (often sub-second possible). Handles millions of inserts per second. |
Joins | Generally Strong: Spark's distributed join strategies handle large joins well. Databricks SQL optimizes joins further. | Improving: Performance varies. Best with denormalized schemas or small dimension table lookups. Large distributed joins can be less efficient than Spark/MPP DWs. |
Concurrency | Good: Scales via clusters. Databricks SQL Warehouses are designed for high-concurrency BI workloads. Autoscaling helps manage load. | Very High: Designed for high query concurrency per node, often suitable for user-facing applications hitting the database directly. |
Optimization Techniques | Delta Lake statistics, partition pruning, Z-Ordering/Liquid Clustering, Photon vectorized execution, Spark Adaptive Query Execution (AQE). | Vectorized execution, sparse primary index, data skipping indices, MergeTree storage engine optimizations, efficient compression, Materialized Views (pre-aggregation). |
Update/Delete Performance | Efficient: Delta Lake's MERGE , UPDATE , DELETE operations leverage transaction log and data skipping efficiently. |
Less Efficient: ALTER TABLE ... UPDATE/DELETE performs background mutations (rewriting data parts). Optimized for append-heavy workloads; frequent updates are costly. |
ClickHouse typically offers lower latency and higher throughput for pure OLAP queries. Databricks provides strong, broad performance across SQL, ETL, and ML, with robust support for data mutability via Delta Lake.
ClickHouse vs Databricks—Scalability & Management
🔮 | Databricks | ClickHouse |
---|---|---|
Compute Scaling | Easy & Automated: Autoscaling Spark clusters and SQL Warehouses (Classic/Serverless). Serverless offers near-instant scaling. | Self-hosted: Manual horizontal scaling (add nodes/shards, rebalance). ClickHouse Cloud: Managed scaling options available (vertical/horizontal). |
Storage Scaling | Independent & Automatic: Scales seamlessly with cloud object storage capacity. | Self-hosted: Scales with node storage capacity. Object Storage: Scales with cloud provider limits. ClickHouse Cloud: Managed, uses object storage. |
Architecture | Decoupled Storage & Compute: Inherent in the Lakehouse design using object storage. | Flexible: Can be Coupled (OSS default) or Decoupled (ClickHouse Cloud / Object Storage config). |
Management | Fully Managed Platform: Databricks handles infrastructure, patching, Spark/Photon optimization. Unity Catalog for governance. | Self-hosted: Significant operational overhead (setup, tuning, upgrades, scaling). ClickHouse Cloud: Managed service reduces operational burden. |
Databricks offers a more automated, elastic, and managed scaling experience due to its cloud-native, decoupled architecture. ClickHouse provides high scalability potential but requires more manual effort and expertise when self-hosted.
ClickHouse vs Databricks—Feature Ecosystem & Transactions
🔮 | Databricks | ClickHouse |
---|---|---|
SQL Support | Supports ANSI SQL via Databricks SQL endpoint. Also native Spark SQL dialect. Multi-language Notebooks (Python, Scala, R, SQL). | Extended SQL dialect with powerful analytical functions; some non-standard syntax/behavior. Primarily SQL interface. |
ML/AI Integration | Core Strength: Deeply integrated with MLflow, libraries (scikit-learn, TensorFlow, PyTorch), AutoML features, LLM support. Model serving capabilities. | Limited Built-in: Primarily serves as a fast data source/sink for external ML frameworks and tools. Some basic ML functions exist. |
Transactions | ACID Transactions: Provided by Delta Lake for data reliability and consistency on object storage. | Limited: No traditional ACID transactions. Mutations are atomic but eventual consistency applies to replicas. Designed for analytical immutability patterns. |
Data Formats | Primary: Delta Lake (on Parquet). Excellent support for reading/writing Parquet, ORC, CSV, JSON, Avro, etc. Handles unstructured data well. | Primary: MergeTree family. Can read/write Parquet, ORC, CSV, JSON via functions/engines but optimized for internal format. Limited unstructured data support. |
Governance | Strong: Centralized via Unity Catalog (access control, lineage, audit, discovery, sharing). | Basic role-based access control (RBAC). Governance relies more on external tools or manual processes. |
Ecosystem | Broad partner ecosystem, extensive connectors via Spark, strong BI tool integration (esp. via Databricks SQL). | Growing ecosystem, especially strong in observability/monitoring (Grafana, Prometheus). Standard JDBC/ODBC drivers. |
Databricks provides a much broader feature set, particularly excelling in multi-language support, deeply integrated ML/AI capabilities, ACID transactions via Delta Lake, and unified governance. ClickHouse focuses intently on maximizing analytical query performance with a powerful, specialized SQL dialect.
ClickHouse vs Databricks—When Would You Pick One Over the Other?
Okay, decision time. Here's a quick rundown:
Choose Databricks if:
- You require a unified platform spanning data engineering (ETL/ELT), SQL analytics, streaming, data science, and machine learning.
- Workloads involve significant data transformations, complex pipeline dependencies, or ML model training integrated with your data storage.
- Robust, integrated ML/AI capabilities (MLflow, libraries, deployment) are a core requirement.
- You are building a Lakehouse architecture leveraging open formats like Delta Lake on cloud object storage.
- A fully managed cloud service abstracting infrastructure and providing automated features (scaling, optimization) is preferred.
- Multi-language support (Python, Scala, R alongside SQL) is essential for your teams.
- ACID transactions on your analytical data are necessary for reliability and consistency.
- Centralized data governance (access control, lineage, auditing) across diverse assets is critical.
Choose ClickHouse if:
- Your absolute top priority is extreme low-latency (sub-second) OLAP query performance, especially for real-time dashboards, analytics APIs, or user-facing features.
- You are analyzing massive volumes of append-heavy data like time-series, events, logs, or metrics where fast aggregations are key.
- Cost-efficiency for high-throughput analytical compute is a major decision factor.
- Very high data ingestion rates (millions of rows/sec) with low latency are required.
- You need a specialized analytical database and plan to handle complex ETL or ML tasks using separate, dedicated systems.
- High query concurrency against the analytical store is a fundamental need.
- You prioritize performance per hardware unit and have the expertise for self-hosting (or choose ClickHouse Cloud for a managed option).
So, wrapping up the ClickHouse vs Databricks comparison: Both are powerful, but they solve different core problems. Databricks provides breadth and integration across the data lifecycle on an open lakehouse foundation. ClickHouse provides unparalleled depth and speed for specific, demanding OLAP query scenarios, excelling where raw analytical performance is the most critical factor. They are both powerful ClickHouse alternatives in different contexts, serving distinct primary needs.
Continue reading...
ClickHouse Alternative 3—Google BigQuery
Google BigQuery is Google Cloud's fully managed, serverless, petabyte-scale data warehouse. It distinguishes itself through its serverless architecture, abstracting infrastructure management and allowing users to focus on querying data using standard SQL. It's designed for large-scale analytics and integrates deeply within the Google Cloud Platform (GCP).
Google BigQuery in a minute - ClickHouse Alternatives - ClickHouse Competitors
Google BigQuery's Architecture: Serverless Power
Google BigQuery achieves its scale and serverless nature by leveraging several core Google infrastructure technologies, fundamentally separating storage and compute:
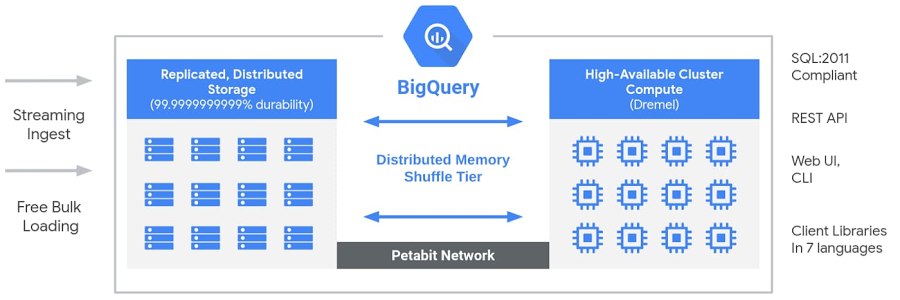
➥ Storage Layer (Colossus + Capacitor)
- Data resides physically within Colossus, Google's global-scale distributed filesystem.
- BigQuery stores table data using Capacitor, its proprietary columnar storage format, optimized for reading semi-structured data efficiently. Capacitor organizes data by column, applies advanced compression, encrypts data at rest, and physically layouts data to maximize parallelism for Dremel.
- Storage is fully managed by Google, scaling transparently and independently of compute. Users interact with logical tables; physical sharding and replication are handled automatically.
➥ Compute Layer (Dremel Engine)
- Query execution is powered by Dremel, Google's large-scale distributed SQL query engine, architected as a Massively Parallel Processing (MPP) system.
- Dremel utilizes a multi-level serving tree: Incoming SQL queries hit a root server, which parses the query, reads metadata, and dispatches query stages to intermediate "mixer" nodes and finally to thousands of leaf nodes or "slots".
- Slots are the fundamental units of computation, representing an abstracted bundle of CPU, RAM, and network resources dynamically allocated for a query. Thousands of slots can operate in parallel, each scanning a portion of the data directly from the Capacitor storage layer.
➥ Execution Coordination & Data Shuffling (Borg & Jupiter)
- Borg, Google's cluster management system, orchestrates the allocation of hardware resources (slots) required by Dremel for each query stage.
- Jupiter, Google's internal petabit-scale network fabric, facilitates extremely fast data shuffling between slots during query execution phases involving large-scale JOINs, aggregations, or window functions.
This architecture allows BigQuery to dynamically provision potentially massive amounts of compute resources tailored to each query's complexity, executing it rapidly without requiring users to perform manual capacity planning or cluster management (especially in the on-demand model).
Core Strength of Google BigQuery
Google BigQuery offers a range of capabilities built upon its serverless architecture:
1) Serverless Operation — No infrastructure (servers, clusters) for users to provision, manage, or tune. Google handles all underlying operations.
2) Automatic Scaling & High Availability — Compute resources (slots) scale automatically based on query complexity and data size (on-demand) or provisioned capacity. Storage scales seamlessly. High availability and durability are built-in.
3) Separation of Storage and Compute — Google BigQuery allows independent scaling and pricing for storage and compute resources.
4) Standard SQL Support — Google BigQuery is compliant with the ANSI:2011 SQL standard, providing a familiar interface for analysts.
5) Google BigQuery ML (BQML) — Enables users to train, evaluate, and predict with machine learning models (regression, classification, clustering, time series forecasting, matrix factorization, DNNs via TensorFlow integration) directly within BigQuery using SQL syntax, minimizing data movement.
6) Google BigQuery Storage APIs:
- Storage Write API — High-throughput gRPC-based API for efficient streaming ingestion (preferred over older
tabledata.insertAll
) and batch loading, offering exactly-once semantics within streams. - Storage Read API — High-throughput gRPC-based API allowing direct, parallelized data access from BigQuery storage into applications or frameworks like Spark, TensorFlow, or Pandas, often faster and cheaper than exporting data.
7) Google BigQuery BI (Business Intelligence) Engine — An in-memory analysis service that caches frequently accessed data used by BI (Business Intelligence) tools (Looker, Looker Studio, Tableau, etc.), accelerating dashboard performance and providing sub-second query latency for interactive exploration.
8) Geospatial Analytics (GIS) — Native support for geography data types (points, lines, polygons based on WGS84 spheroid) and standard GIS functions.
9) Federated Queries — Ability to query data residing in external sources (Google Cloud Storage files, Cloud SQL, Spanner, Cloud Bigtable) directly without loading it into Google BigQuery’s managed storage.
10) Flexible Pricing Models — Google BigQuery offers various pricing models:
- On-Demand — Pay per terabyte (TB) of data processed by queries. Includes a monthly free processing tier. Best for variable or unpredictable workloads.
- Capacity Pricing (Slots) — Purchase dedicated processing capacity (slots) via Flex Slots (minimum 60-second commitment) or monthly/annual commitments. Available in Standard, Enterprise, and Enterprise Plus editions, offering varying features, performance levels, and pricing for predictable costs on sustained workloads. Storage is billed separately based on data volume (active vs long-term).
11) Security & Governance — Google BigQuery easily integrates with GCP IAM for authentication/authorization; supports column-level and row-level security, data masking, VPC Service Controls, customer-managed encryption keys (CMEK), detailed audit logging via Cloud Logging, and integration with Data Loss Prevention (DLP) API.
12) Data Management & Optimization — Google BigQuery supports table partitioning (by time-unit, integer range, or ingestion time) and table clustering (by specified columns) to optimize query performance and cost by pruning data scanned based on query predicates.
…and so much more!
Pros and Cons of Google BigQuery:
Google BigQuery Pros:
- Google BigQuery runs on a serverless model with no infrastructure to manage.
- Google BigQuery scales instantly to petabyte‑size data warehouses without cluster planning.
- Google BigQuery executes SQL queries over terabytes of data in seconds using Dremel‑based columnar storage.
- Google BigQuery supports the standard SQL dialect (plus legacy SQL) so analysts don’t face a steep learning curve.
- Google BigQuery includes BigQuery ML for training and using machine learning models with simple SQL commands.
- Google BigQuery offers federated queries to run statements on external databases like Cloud SQL and Spanner.
- Google BigQuery integrates natively with Google Cloud services such as Storage, Pub/Sub, and Dataflow.
- Google BigQuery provides flexible pricing with on‑demand and flat‑rate options to match different workload patterns.
- Google BigQuery encrypts data at rest and in transit and enables fine‑grained access via IAM policies.
- Google BigQuery auto‑backs up data and maintains high availability through built‑in replication.
Google BigQuery Cons:
- Google BigQuery charges per TB scanned in on‑demand mode, which can lead to unpredictable query costs.
- Choosing the right edition, number of slots, and commitment type requires careful analysis to be cost-effective.
- Users have less direct control over query execution compared to systems like ClickHouse. Optimization relies mainly on SQL patterns, partitioning, and clustering.
- Google BigQuery can exhibit higher initial latency (seconds) for simple or small queries compared to low-latency optimized engines, making it less ideal for real-time interactive applications needing millisecond responses.
- DML limitations. Subject to quotas on Data Manipulation Language (UPDATE, DELETE, INSERT) frequency and volume; not designed for high-frequency transactional workloads (OLTP).
- Exporting large datasets out of GCP can incur significant network egress charges.
- Scheduled queries can only run at 15‑minute minimum intervals, which restricts near‑real‑time workflows.
- Query performance can vary due to slot contention or cold‑start delays.
- BigQuery is exclusively available on Google Cloud Platform.
Let's get into the ClickHouse vs BigQuery showdown. Both are major players that can handle massive data loads, but they take different approaches and excel in different spots.
ClickHouse vs BigQuery: What's Best for You?
Choosing between them really hinges on what you need to do, how fast you need it, and what your setup looks like.
Clickhouse vs BigQuery—Architecture & Management
🔮 | Google BigQuery | ClickHouse |
---|---|---|
Core Design | Fully managed, serverless cloud data warehouse. | Open source columnar analytical database (DBMS). |
Deployment Model | Exclusively on Google Cloud Platform (GCP) as a managed service. | Flexible: Self-hosted (on-prem, any cloud), Kubernetes, Managed Service (ClickHouse Cloud on GCP/AWS/Azure, others). |
Storage/Compute | Fully Decoupled: Storage (Colossus/Capacitor) and Compute (Dremel/Slots) scale and operate independently. | Flexible: Coupled (OSS default, shared-nothing) or Decoupled (ClickHouse Cloud / Object Storage config). |
Management | Zero-Ops: Fully managed by Google. Users manage data and queries, not infrastructure. | High (Self-hosted): Requires significant setup, tuning, scaling, upgrades. Low (ClickHouse Cloud): Managed service option. |
Resource Model | Abstracted: Compute measured in Slots (dynamic resource units). Users don't manage VMs/nodes directly. | Direct: Resources map directly to hardware (CPU, RAM, Disk - OSS) or specific service tiers (ClickHouse Cloud). |
Storage Format | Proprietary columnar format (Capacitor) on Colossus filesystem. | Proprietary columnar format (MergeTree engine family) on local disk or cloud object storage. Known for high compression. |
BigQuery offers extreme operational simplicity via its serverless, GCP-native model. ClickHouse provides deployment flexibility (multi-cloud, on-prem, managed) and more direct control over resources and configuration, especially when self-hosted.
Clickhouse vs BigQuery—Performance Profile
🔮 | Google BigQuery | ClickHouse |
---|---|---|
Query Speed (OLAP) | Good to Fast: Excels at large-scale scans/joins/aggregations due to massive parallelism. Latency typically seconds to tens of seconds. Slower for small/simple queries. | Extremely Fast: Optimized for low latency (often sub-second) on analytical queries, especially scans, aggregations, time-series analysis. High throughput. |
Real-time Ingestion | Good: High throughput via Storage Write API (gRPC streaming). End-to-end latency typically seconds. | Excellent: Very high throughput batch inserts. Kafka engine & integrations support near-real-time. Lower ingestion latency often achievable. |
Tuning & Optimization | Limited User Control: Relies on Google's auto-optimization. Users influence via schema (partitioning/clustering) and SQL patterns (declarative optimization). | Extensive Tuning: Fine-grained control via primary/skipping indexes, Materialized Views, engine settings, hardware config (imperative tuning). Requires expertise. |
Performance Consistency | Variable (On-Demand): Can fluctuate due to shared resources. More Predictable (Capacity): Dedicated slots offer steadier performance. |
Generally Predictable: Latency is more consistent when cluster is adequately resourced and tuned for the workload. |
Complex Queries (Joins) | Handles very large, complex joins well due to distributed shuffle network (Jupiter) and massive parallelism. | Performance varies; large distributed joins can be less efficient than BigQuery/Spark. Best with denormalization or smaller dimension joins. |
ClickHouse typically delivers significantly lower latency for targeted OLAP queries and offers more tuning levers. BigQuery provides effortless scaling for extremely large or complex ad-hoc queries and handles massive joins robustly, albeit often with higher baseline latency.
Clickhouse vs BigQuery—Scalability & Concurrency
🔮 | Google BigQuery | ClickHouse |
---|---|---|
Scalability Approach | Serverless & Automatic: Managed entirely by Google, scales transparently based on demand or capacity. | Horizontal: Scales by adding nodes/shards. Requires manual configuration/rebalancing (OSS) or managed service features (ClickHouse Cloud). |
Compute Scaling | Automatic: Scales via dynamic slot allocation (on-demand) or provisioned slot capacity (Editions). | Manual/Managed: Add compute nodes/VMs (OSS) or adjust managed service tiers (ClickHouse Cloud). |
Storage Scaling | Automatic & Independent: Managed by Google on Colossus. | Manual/Managed: Add disk/nodes (OSS coupled) or relies on object storage scaling (decoupled). |
Concurrency | High: Default quotas allow many concurrent queries (e.g: 100+ interactive). Managed via slot availability. | Very High: Designed for high concurrency; limits depend on node resources/cluster size/configuration. Often supports thousands per service. |
BigQuery offers unparalleled ease of scaling. ClickHouse provides high scalability potential but requires more planning and active management if self-hosted.
Clickhouse vs BigQuery—Data Handling, SQL, & Ecosystem
🔮 | Google BigQuery | ClickHouse |
---|---|---|
SQL Dialect | Standard SQL (ANSI:2011 compliant). Familiar for most analysts. | Extended SQL dialect with powerful analytical functions, array/map manipulation, and some non-standard syntax optimized for performance. |
Updates/Deletes | Standard SQL UPDATE /DELETE /MERGE DML statements. Subject to quotas and not optimized for high-frequency (OLTP) use. |
Limited via asynchronous ALTER TABLE... UPDATE/DELETE mutations. Optimized for append-heavy workloads; mutations are background rewrite operations. |
Materialized Views | Supported, with automatic refresh and smart tuning options. | Supported, powerful mechanism often used for incremental aggregation and query acceleration. Requires manual definition. |
Ecosystem | Deep GCP Integration: Native connectors to Dataflow, Pub/Sub, Dataproc, AI Platform, Cloud Functions, Looker/Looker Studio. Federated Queries. | Strong Open Source & Observability: Integrates well with Kafka, Prometheus, Grafana, Vector DBs. Reads many formats/sources (S3, GCS, HDFS, JDBC/ODBC). Growing connectors. |
BigQuery provides standard SQL and seamless integration within the GCP ecosystem. ClickHouse offers a more specialized, powerful SQL dialect for analytics and strong integrations within open source and observability stacks. BigQuery has better support for standard DML, while ClickHouse focuses on append-heavy patterns.
Clickhouse vs BigQuery—Cost Framework
🔮 | Google BigQuery | ClickHouse |
---|---|---|
Model | Dual: On-demand (per TB scanned) or Capacity (per slot-hour/committed, via Editions). Storage billed separately (active/long-term rates). | Flexible: OSS is free (pay infrastructure/operational costs). Managed ClickHouse Cloud typically usage-based (compute, storage, data scan/transfer). |
Cost Driver | Data scanned (On-Demand) or Slot reservation/commitment (Capacity). | Hardware/Instance resources (OSS) or Managed service tier/usage metrics (ClickHouse Cloud). |
Predictability | On-demand can be highly variable. Capacity offers predictable compute costs. | Self-hosted depends on infrastructure stability. Managed Cloud plans often offer better predictability based on chosen tier/commitments. |
Efficiency | Pay-per-scan incentivizes strict query optimization (partitioning/clustering crucial). Can be cost-effective for sporadic use. | High performance & compression often lead to lower resource needs (compute/storage) for the same analytical task, potentially yielding better price-performance. |
ClickHouse's efficiency often makes it more cost-effective for sustained, high-volume analytical workloads. BigQuery's serverless on-demand model can be cheaper for infrequent or exploratory usage but requires careful optimization or capacity planning to control costs under heavy load.
When Would You Choose BigQuery vs ClickHouse?
Choose Google BigQuery if:
- You are deeply integrated into the Google Cloud Platform (GCP) ecosystem.
- Minimal operational overhead and a fully managed, serverless experience are top priorities.
- Your primary use cases involve large-scale reporting, ad-hoc data exploration, BI dashboards, where multi-second latency is generally acceptable.
- You need effortless, automatic scaling for unpredictable workloads or massive datasets without manual intervention.
- Your team prefers standard SQL and prioritizes ease of use over performance tuning control.
- Seamless integration with specific GCP services like BQML, AI Platform, Dataflow, or Looker is crucial.
- Built-in Geospatial (GIS) capabilities are required.
Choose ClickHouse if:
- Extreme low-latency query performance (sub-second) is essential for your application (e.g: real-time dashboards, analytics APIs, user-facing features).
- You are processing high volumes of time-series, event, log, or telemetry data requiring rapid aggregation and filtering.
- High data ingestion throughput and high query concurrency are critical operational requirements.
- You need fine-grained control over performance tuning, system configuration, and hardware/resource allocation (especially if self-hosting).
- Storage efficiency (due to high compression) and potentially better cost-performance for compute-intensive analytical workloads are major drivers.
- You require advanced analytical SQL functions or specialized data types (Arrays, Maps, LowCardinality) for optimization.
- Deployment flexibility (multi-cloud, on-prem, managed options) is important, or you prefer an open source core.
So, wrapping up the ClickHouse vs BigQuery comparison: Google BigQuery really shines with its operational simplicity, automatic scalability, and tight integration with GCP, making it ideal for large-scale analytics that require minimal upkeep. ClickHouse, on the other hand, is perfect for high-performance OLAP workloads that demand low latency and high throughput, offering more control and flexibility in how it's deployed.
Continue reading...
ClickHouse Alternative 4—Amazon Redshift
Amazon Redshift is AWS's fully managed, petabyte-scale cloud data warehouse service. As one of the prominent ClickHouse alternatives within the AWS ecosystem, it's designed for large-scale data analysis and business intelligence (BI) workloads. Redshift offers compatibility with standard SQL (with PostgreSQL-like syntax), integrates with numerous BI tools, and connects tightly with other AWS services.
Intro to Amazon Redshift - ClickHouse Alternatives - ClickHouse Competitors
Amazon Redshift Architecture
Amazon Redshift traditionally employs a shared-nothing, Massively Parallel Processing (MPP) architecture built around clusters. Redshift is meant to work in cluster formation. A typical Redshift Cluster has two or more Compute Nodes which are coordinated through a Leader Node. All client applications communicate with the Cluster only through the Leader Node. However, recent developments (RA3 nodes, Serverless) have introduced significant architectural shifts, particularly decoupling storage from compute.
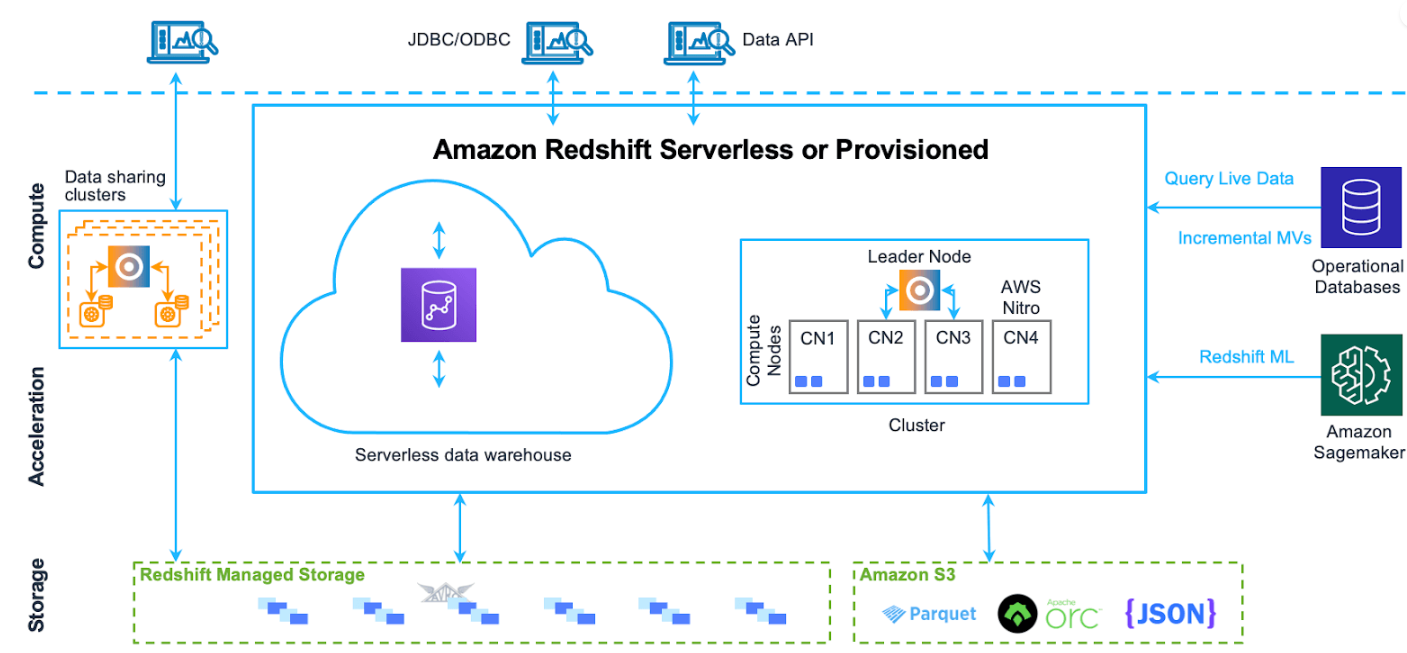
The Amazon Redshift architecture can be broken down into the following components:
➥ Leader Node
The leader node acts as the entry point for client connections and query coordination. It receives SQL queries, parses them, develops an optimized query execution plan (leveraging table statistics and metadata), and coordinates the parallel execution of plan fragments across the compute nodes. It aggregates intermediate results from compute nodes before returning the final result to the client.
➥ Compute Nodes
The compute nodes execute the query plan fragments assigned by the leader node and store a portion of the user table data locally. Each compute node is partitioned into slices, where each slice gets a portion of the node's memory and disk space and processes a part of the workload in parallel. Data distribution across slices is determined by the table's distribution style.
Amazon Redshift offers different node types impacting the storage/compute relationship:
- Node Types (Evolution):
- Dense Compute (DC2 - older) — Optimized for performance with local SSD storage. Compute and storage are tightly coupled.
- Dense Storage (DS2 - older) — Optimized for large data volumes using HDDs. Compute and storage are coupled.
- RA3 Nodes (Current Generation) — This is a significant architectural shift. RA3 nodes feature managed storage (RMS). They use large, high-performance local SSDs as a cache, while the primary, durable copy of the data resides transparently in Amazon S3, managed by Redshift. This separation allows compute (number/size of RA3 nodes) and managed storage (backed by S3) to be scaled and billed somewhat independently. RMS automatically manages data placement between S3 and the local SSD cache based on access patterns.
- Amazon Redshift Serverless — An option that abstracts away nodes entirely. You specify compute capacity in Redshift Processing Units (RPUs), and AWS automatically provisions and scales the underlying resources. It uses RMS for storage.
➥ Redshift Managed Storage (RMS)
As mentioned, this decouples storage from compute nodes by using S3 as the primary durable store, with compute nodes caching active data locally on SSDs.
➥ Node Slices
Each compute node is partitioned into multiple slices. Each slice receives a portion of the node's memory and disk space and processes a segment of the workload assigned to the node. Data distribution across slices (and nodes) is determined by the table's distribution style (KEY, ALL, EVEN).
➥ Internal Network
Amazon Redshift uses a private, high-speed network between the leader node and compute nodes, allowing for fast communication between them.
Amazon Redshift Key Features
Amazon Redshift offers a range of features tailored for cloud data warehousing:
1) Columnar Storage & Compression — Amazon Redshift stores data columnarly for efficient I/O during analytical queries. Supports various compression encodings (ZSTD, LZO, AZ64), often applied automatically.
2) Massive Parallel Processing (MPP) — Amazon Redshift distributes query execution across multiple compute nodes/slices for high throughput on large datasets.
3) Redshift Security — Amazon Redshift provides security features like encryption at rest (KMS/HSM) and in transit (SSL), VPC integration, IAM-based access control, row-level and column-level security.
4) Automated Scaling — Amazon Redshift can automatically scale storage and compute resources based on your workload requirements.
5) Concurrency Scaling — Amazon Redshift automatically adds temporary cluster capacity (billed per second when active) during high concurrent query bursts to maintain throughput, accessing data via RMS/Spectrum.
6) Amazon Redshift Spectrum — With Amazon Redshift Spectrum you can directly query data in Amazon S3 without having to load it into Redshift.
7) Advanced Machine Learning — Amazon Redshift uses machine learning capabilities to optimize and improve query performance.
8) Easy Deployment — Amazon Redshift is fast and simple to deploy as it is a fully managed service from AWS.
9) AWS Ecosystem Integration — Amazon Redshift is tightly integrated with services like S3, Glue (ETL/Catalog), Kinesis (streaming), IAM (security), Lake Formation, SageMaker (ML), etc.
10) Performance Optimizations — Amazon Redshift uses Sort Keys (defines data sort order within blocks to aid range filtering), Distribution Keys/Styles (controls how data is spread across nodes/slices to optimize JOINs and aggregations), workload management (WLM) queues, short query acceleration (SQA), and ML-driven optimizations (like automatic table optimization).
12) Flexible Billing — Amazon Redshift offers a pay-as-you-go pricing model based on provisioned resources like nodes, concurrency scaling, and scanned data.
…and so much more!
Pros and Cons of Amazon Redshift:
Amazon Redshift Pros:
- Strong performance for analytics. MPP architecture and columnar storage deliver high throughput for complex queries and large datasets.
- Scales to petabytes. RA3/Serverless options offer good elasticity for compute and storage.
- Mature service and ecosystem. Well-established service with a large user base, good documentation, and deep integration within the AWS ecosystem.
- Cost-Effective potential. Often considered price-competitive, especially compared to traditional on-premises warehouses or when using reserved instances for provisioned clusters.
- Familiar SQL interface. PostgreSQL compatibility lowers the barrier for analysts and simplifies tool integration.
- Managed service. AWS handles patching, backups, provisioning, and monitoring, reducing operational burden.
- Amazon Redshift provides Redshift spectrum which is useful for extending queries to data lakes without ETL.
Amazon Redshift Cons:
- Performance of Amazon Redshift heavily depends on choosing appropriate Distribution Keys and Sort Keys. Requires understanding data access patterns.
- Base concurrency of Redshift is limited (historically ~50 concurrent queries per cluster/endpoint across queues). Concurrency Scaling helps but adds cost and has limits (up to 10 additional clusters). Can be a bottleneck for highly interactive applications compared to systems like ClickHouse or Snowflake (with multi-cluster warehouses).
- Resizing provisioned clusters (adding/removing nodes) can take minutes to hours, involving data redistribution, making it less elastic than truly serverless options or Snowflake warehouses.
- Amazon Redshift is primarily designed for and integrated with the AWS ecosystem.
- Query compilation latency. Initial execution of a query involves compilation, which can add noticeable latency (seconds) the first time it runs after a cluster restart or code change. (Result caching mitigates this for subsequent identical queries).
- Data loading at scale sometimes hits bottlenecks without parallel frameworks.
- Costs climb quickly with large clusters or many concurrent queries.
- While compute nodes in Redshift are distributed, the Leader Node is logically single (though managed for HA by AWS). Historically, Leader Node issues could impact cluster availability.
Alright, let's break down the ClickHouse vs Redshift comparison. Both are powerful columnar analytical databases, but cater to different strengths and operational preferences.
ClickHouse vs Redshift: What's Best for You?
Choosing between ClickHouse vs Redshift really depends on what you need to do, your tech setup, and how much you want to manage yourself. We'll dig into the technical bits to help you figure that out.
ClickHouse vs Redshift—Architecture & Deployment
🔮 | Amazon Redshift | ClickHouse |
---|---|---|
Core Design | Cloud Data Warehouse, MPP architecture. | Open Source Columnar DBMS, Vectorized Execution engine. |
Deployment Model | Fully managed AWS service: Provisioned Clusters (RA3 nodes primary) or Serverless (RPU-based). | Flexible: Self-hosted (OSS on-prem/cloud), Kubernetes, Managed Service (ClickHouse Cloud on AWS/GCP/Azure, others). |
Storage/Compute | Separated (RA3/Serverless): Via Redshift Managed Storage (RMS) using S3 + local SSD cache. <br> (Coupled in older DC2/DS2 nodes). | Flexible: Coupled (OSS default, shared-nothing) or Decoupled (ClickHouse Cloud / Object Storage config). |
Data Storage | Columnar format on RMS (S3 backed) or local node storage (legacy). Data distributed via Distribution Keys/Styles. | Columnar format (MergeTree engine family) on local disk or cloud object storage. Data typically sharded across nodes. High compression. |
Query Execution | MPP: Parallel execution across nodes/slices coordinated by Leader Node/Endpoint. Involves query compilation step. | Vectorized Execution: Processes data in batches (vectors) for CPU efficiency. Typically no query compilation overhead. |
Source Code | Closed-source (AWS proprietary). | Open Source (Apache 2.0 License). |
Amazon Redshift offers a managed AWS experience with evolving storage/compute separation (RA3/Serverless). ClickHouse provides deployment flexibility (OSS/Managed, multi-cloud/on-prem) and focuses on vectorized execution speed.
ClickHouse vs Redshift—Performance Profile
🔮 | Amazon Redshift | ClickHouse |
---|---|---|
Query Speed (OLAP) | Fast: Strong performance for BI/DW queries, especially when well-tuned with Sort/Dist Keys. Query compilation adds initial latency (seconds). | Extremely Fast: Often leads benchmarks for raw OLAP query speed (scans, aggregations). Lower latency due to vectorized engine & no compilation step. |
Concurrency | Moderate Base: Limited native concurrency per cluster/endpoint (managed via WLM). Extended via paid Concurrency Scaling (adds temporary clusters). | Very High: Designed for high query concurrency (hundreds/thousands per service/node possible), suitable for user-facing applications. |
Optimization | Relies heavily on Schema Design: Sort Keys, Distribution Keys are crucial. Automated features (Auto Table Optimization, Auto MVs) assist. Workload Management (WLM) for priority. | Relies on Engine & Indices: Primary Key (sorting), skipping indexes, Materialized Views, projections, engine settings. Often requires more manual tuning for peak performance. |
Data Compression | Good columnar compression (ZSTD, AZ64). | Excellent, highly tunable columnar compression (LZ4, ZSTD, Delta, Gorilla, etc.), often achieving higher ratios than Redshift. |
Real-time Suitability | Less Ideal: Query compilation latency and base concurrency limits make it better suited for BI/reporting than millisecond real-time dashboards. | Optimized: Designed for real-time analytics, low-latency user-facing dashboards, and APIs due to speed and high native concurrency. |
Joins | Handles standard SQL JOINs well, performance heavily dependent on Distribution Keys matching join keys. | Supports JOINs; performance varies. Often better with denormalization, dictionary lookups, or smaller dimension joins compared to large distributed fact-fact joins. |
ClickHouse generally provides lower query latency and superior native concurrency, excelling in real-time scenarios. Amazon Redshift offers robust performance for traditional DW workloads, especially within AWS, with performance highly dependent on schema design (keys).
ClickHouse vs Redshift—Scalability & Elasticity
🔮 | Amazon Redshift | ClickHouse |
---|---|---|
Compute Scaling | Provisioned: Elastic Resize (manual/scheduled, minutes-hours). Concurrency Scaling (auto temp clusters). <br> Serverless: Auto-scales based on RPU config. | Self-hosted: Manual horizontal scaling (add nodes/shards, rebalance). <br> ClickHouse Cloud: Managed scaling options (vertical/horizontal). |
Storage Scaling | Independent (RA3/Serverless): Scales via RMS backed by S3. <br> (Coupled with nodes in legacy DC2/DS2). | Self-hosted: Scales with node storage (coupled). <br> Decoupled: Scales independently via object storage (ClickHouse Cloud / config). |
Workload Isolation | Limited: WLM provides query queues but resources on a provisioned cluster are shared. Full isolation requires separate clusters/endpoints. | Possible: Achieved via careful cluster design (self-hosted) or inherently better through service separation in managed platforms like ClickHouse Cloud. |
Elasticity | Good (Serverless/RA3): Serverless auto-scales compute; RA3 decouples storage. <br> Moderate (Provisioned): Elastic Resize is slow; Concurrency Scaling provides burst elasticity. | Variable: Self-hosted requires manual effort (less elastic). Managed Cloud services offer significantly better elasticity. |
Amazon Redshift Serverless and RA3+RMS offer good elasticity within AWS. Provisioned Redshift cluster resizing is less elastic. ClickHouse scalability is high but requires more manual effort (OSS) or relies on managed service features (Cloud).
ClickHouse vs Redshift—Data Handling, Features, & Ecosystem
🔮 | Amazon Redshift | ClickHouse |
---|---|---|
SQL Support | Standard SQL, closely compatible with PostgreSQL dialect. | Extended SQL dialect with powerful analytical functions, array/map operations; some non-standard syntax. |
Data Types | Standard SQL types. SUPER type for ingesting/querying semi-structured data (JSON). |
Rich types: Array , Map , Tuple , Nested , LowCardinality , Enum , UUID , IPv4 /IPv6 , native JSON object, AggregateFunction . Geospatial types available. |
Updates/Deletes | Standard SQL UPDATE /DELETE supported. Can be resource-intensive (involve block rewrites) on large tables. |
Limited via asynchronous ALTER TABLE... UPDATE/DELETE mutations (background rewrites). Best suited for append-heavy workloads. |
Primary/Unique Keys | Defined syntactically but not enforced; purely informational for the query planner and modeling tools. | Primary Key is fundamental for MergeTree: defines sort order for sparse index, enabling efficient range scans. Not for uniqueness enforcement. |
Indexing | No traditional indexes. Relies on Sort Keys (for range pruning via zone maps) and Distribution Keys (for data placement). | Sparse Primary Index (based on Primary Key sort order). Secondary Data Skipping Indexes (minmax, set, bloom filter, ngram, tokenbf) to prune data blocks (granules). |
Materialized Views | Supported, including options for incremental refresh and automated recommendations/creation based on query patterns. | Supported, powerful mechanism often used for incremental aggregation, pre-joining data, or creating query-specific projections. |
Ecosystem | Deep AWS Integration: S3, Glue, Kinesis, Lambda, SageMaker, Lake Formation, IAM, CloudWatch. Wide support from BI/ETL vendors. | Strong Open Source & Observability: Kafka, Prometheus, Grafana, Vector DBs. Growing BI/ETL support via JDBC/ODBC. Cloud-agnostic integrations (S3, GCS, HDFS). |
Management | Fully Managed by AWS. Serverless option further simplifies operations. Requires understanding of keys/WLM for optimization. | High Effort (OSS): Requires expertise in setup, tuning, scaling, upgrades. <br> Low Effort (Cloud): Managed services significantly reduce burden. |
Amazon Redshift offers a familiar PostgreSQL-like SQL experience and unparalleled integration within the AWS ecosystem. ClickHouse provides more specialized data types and analytical functions, a unique indexing approach, and greater flexibility via its open source nature, but with a steeper learning curve for its SQL dialect and tuning.
ClickHouse vs Redshift—Pricing Framework
🔮 | Amazon Redshift | ClickHouse |
---|---|---|
Model | Flexible: On-Demand (per node-hour or RPU-hour), Reserved Instances (1/3 year commitments for provisioned clusters, significant savings). Separate billing for RMS Storage, Spectrum scans, Concurrency Scaling. | Flexible: OSS is free (pay infrastructure/operational costs). Managed ClickHouse Cloud typically usage-based (compute, storage, data scan/transfer, features). |
Cost Driver | Provisioned node hours/RPUs, RI commitments, data scanned (Spectrum), RMS storage volume, Concurrency Scaling usage. | Hardware/Instance resources (OSS) or Managed service tier/usage metrics (ClickHouse Cloud). |
Predictability | Reserved Instances offer high predictability for provisioned compute. Serverless/On-Demand can be variable but controllable via settings/usage. | Self-hosted depends on infra stability. Managed Cloud plans often offer predictable tiers or usage-based billing that can be monitored. |
Efficiency | RA3/Serverless improve cost-efficiency over older nodes. RI discounts significant. Performance-per-dollar depends heavily on tuning (keys) and workload fit. | High performance & compression efficiency often lead to lower resource needs (compute/storage) for analytical tasks, frequently resulting in better raw price-performance. |
Amazon Redshift offers various pricing models, with Reserved Instances providing cost certainty for predictable workloads in AWS. ClickHouse's efficiency often translates to better price-performance for compute-heavy analytical tasks, with OSS offering the lowest potential TCO if expertise is available.
When Would You Choose Redshift vs ClickHouse?
Choose Amazon Redshift if:
- You are heavily invested and operate primarily within the AWS ecosystem.
- You need a robust, general-purpose cloud data warehouse for traditional BI, reporting, and complex SQL analytics where multi-second latency is acceptable.
- You prefer a fully managed service from AWS, potentially leveraging Serverless for simplified operations or Reserved Instances for cost predictability.
- Your team is comfortable with PostgreSQL-like SQL and AWS management consoles/APIs.
- Seamless integration with AWS Glue Data Catalog, Lake Formation, Kinesis, S3, and SageMaker is critical.
- Workloads can benefit from Redshift Spectrum for querying S3 data lakes or require features like automated Materialized Views.
- Moderate base concurrency is sufficient, or you can leverage Concurrency Scaling within your budget for bursts.
Choose ClickHouse if:
- Your primary requirement is extreme query speed and low latency (sub-second) for OLAP, essential for real-time dashboards, analytics APIs, or user-facing features.
- High native query concurrency (handling hundreds or thousands of simultaneous queries efficiently) is fundamental.
- You are processing massive volumes of event streams, logs, time-series, or telemetry data requiring fast aggregations and filtering.
- Cost-performance and storage efficiency for analytical workloads are major decision drivers.
- You need deployment flexibility (on-premises, multi-cloud, Kubernetes) or strongly value open source software.
- Your analytical workloads can significantly benefit from ClickHouse's specialized data types (Arrays, Maps, LowCardinality, AggregateFunction) or data skipping indexes.
- You have the operational capacity to manage it (for OSS) or prefer a managed service laser-focused on analytical speed (ClickHouse Cloud).
TL;DR: Amazon Redshift is a well-established data warehouse solution on AWS. It provides many features and strong integration, making it great for various traditional BI and analytics tasks. ClickHouse is a fast analytical engine. It excels in situations that need speed, concurrency, and efficiency, like real-time applications. It often offers better price-performance but requires specialized knowledge.
Continue reading...
🗄️ ClickHouse Alternatives: Category 2—ClickHouse vs Traditional Relational Databases (OLTP-Focused)
This category covers the operational workhorses of the internet: Relational Database Management Systems (RDBMS) like MySQL and PostgreSQL. These databases are typically row-oriented and optimized for Online Transaction Processing (OLTP). Their primary function is efficiently handling high volumes of small, concurrent read and write operations while guaranteeing strong data consistency through ACID transactions (Atomicity, Consistency, Isolation, Durability). This design philosophy is fundamentally different from ClickHouse's columnar, Online Analytical Processing (OLAP) focus, which prioritizes complex queries scanning large volumes of data across fewer columns.
Let's see why these systems differ so significantly.
Note: It's important to talk about these because users sometimes attempt to run heavy analytics directly on their transactional databases, inevitably encounter performance limitations, and then start seeking OLAP solutions like ClickHouse.
Quick Refresher: OLTP vs OLAP
🔮 | OLTP (Online Transaction Processing) | OLAP (Online Analytical Processing) |
---|---|---|
Main Purpose | Manage operational data, support business processes. | Analyze historical data, support decision-making, reporting, BI. |
Workload | High volume of short, simple transactions (INSERT, UPDATE, DELETE, SELECT by ID). | Complex queries, aggregations, scans over large datasets. Read-heavy. |
Data Structure | Typically normalized (3NF), minimizes redundancy. | Often denormalized (star/snowflake schema), optimized for query performance. |
Storage | Primarily Row-oriented (efficient access to entire records). | Primarily Column-oriented (efficient access to specific columns, high compression). |
Concurrency | High concurrency for short transactions. | High concurrency for analytical queries. |
Data Scope | Current, operational data. | Historical, aggregated data. |
Examples | E-commerce order processing, banking transactions, user registration. | Sales trend analysis, customer segmentation, financial forecasting. |
Key DB Examples | MySQL, PostgreSQL, SQL Server (OLTP mode), Oracle (OLTP mode). | ClickHouse, Snowflake, BigQuery, Redshift, Apache Druid. |
Now that you know the difference between OLTP vs OLAP. Let’s move on to next ClickHouse alternative which is MySQL.
ClickHouse Alternative 5—MySQL
MySQL is one of the world's most widely deployed open source RDBMS. Originating in the mid-1990s and now managed by Oracle, it powers countless websites, applications, and services. Its strengths lie in reliability, ease of use, strong performance for transactional (OLTP) workloads (particularly with its default InnoDB storage engine), a vast ecosystem, and a large, active community.
What is MySQL? - ClickHouse Alternatives - ClickHouse Competitors
MySQL Architecture—Optimized for Transactions (InnoDB Focus)
MySQL employs a layered client-server architecture with a pluggable storage engine model, allowing different engines to handle data storage and retrieval based on needs.
Lets dig into the detailed breakdown of MySQL architecture:
MySQL follows a three-layered system of client/server, SQL, and storage-engine layers. The client/server layer handles connections, authentication, and resource allocation. The SQL layer parses, preprocesses, and optimizes SQL, turning statements into execution plans. The storage-engine layer performs data I/O operations and manages indexes via plug‑in modules.
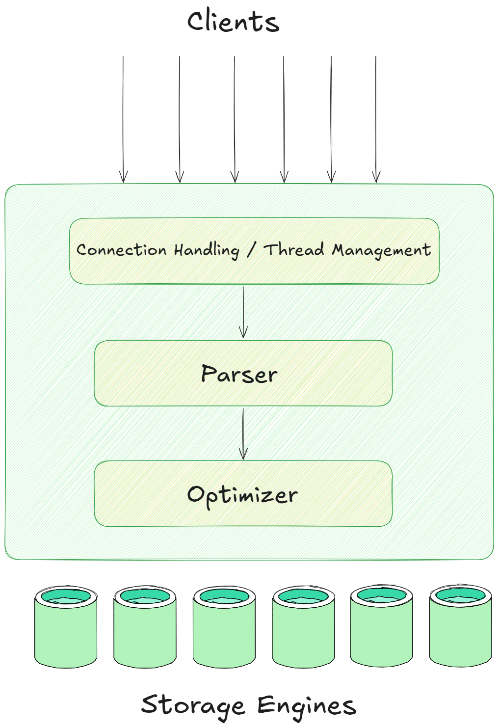
➥ Connection Handling / Thread Management (Client/Server Layer)
- The
mysqld
server process listens for client connections. - Historically used a one-thread-per-connection model, which could limit scalability under very high connection counts due to thread creation/destruction overhead.
- Modern versions (including Community Edition) offer a thread pool plugin, which manages a pool of worker threads to handle incoming connections more efficiently, significantly improving performance and scalability for high-concurrency applications.
➥ Query Processing (SQL Layer)
SQL layer handles the lifecycle of SQL statements:
- Parsing — Incoming SQL queries are parsed into an internal representation (parse tree).
- Analysis & Optimization — The query is validated. The cost-based optimizer analyzes potential execution plans, considering available indexes (B+Trees), table statistics, and join strategies. It chooses the plan estimated to be fastest for typical OLTP access patterns (like selective lookups via primary or secondary keys, small range scans).
- Execution — The execution engine interprets the optimized plan and interacts with the chosen storage engine via a common API to fetch or modify data.
➥ Storage Engine Layer
The storage-engine layer defines how data is physically stored, indexed, and accessed. MySQL's pluggable architecture allows different engines, but InnoDB is the default and most commonly used for transactional workloads.
InnoDB Engine
- Storage Format — Fundamentally row-oriented. Data is stored in pages (typically 16KB), with all columns for a given row usually residing together. Data is organized within tablespaces.
- Indexing — Uses B+Tree indexes. The primary key is typically stored as a clustered index (physically ordering the row data). Secondary indexes contain the primary key value to look up the full row. B+Trees excel at point lookups (WHERE id = ?) and range scans (WHERE date BETWEEN ? AND ?).
- Concurrency — Implements MVCC (Multi-Version Concurrency Control) using undo logs, allowing reads to proceed without blocking writes and vice-versa, providing snapshot isolation.
- ACID Compliance — Gurantees Atomicity, Consistency, Isolation, and Durability through mechanisms like redo logs (Write-Ahead Logging principle for durability) and undo logs (for atomicity/rollback). Uses row-level locking for fine-grained concurrency control.
- Caching — Relies heavily on the InnoDB Buffer Pool, an in-memory cache for frequently accessed data and index pages, minimizing disk I/O for transactional operations. Features like the Adaptive Hash Index can further speed up lookups in memory.
- Durability Aids — Uses mechanisms like a doublewrite buffer to prevent data corruption from partially written pages during crashes.
MyISAM Engine (Legacy)
MyISAM Engine is a non-transactional engine using table-level locking. Faster for certain read-heavy workloads but unsuitable for concurrent writes or requiring ACID guarantees. Less common for new applications.
Other Engines
MySQL also supports engines like MEMORY
(for temporary in-memory tables) and NDB
(the engine powering MySQL NDB Cluster, a separate distributed, shared-nothing transactional database product).
For Connection and Thread Management
MySQL by default uses one-thread-per-connection, spinning up a server thread for each client session. Enterprise Edition’s thread pool plugin lets you group connections into thread groups, which can reduce overhead and improve throughput in high‑concurrency environments.
MySQL Key Features
MySQL offers a rich feature set, particularly strong for transactional applications:
1) Open Source and Cross-Platform — MySQL is open source and free to use under the GNU GPL(General Public License). You can modify, distribute, and use it for any purpose. And runs on Linux, Windows, macOS, and other platforms.
2) Client-Server Architecture — MySQL uses a client-server model. The server handles database operations, while clients send queries and receive results.
3) Storage Engine Flexibility — MySQL Supports multiple storage engines, including InnoDB (default, supports transactions, row-level locking, foreign keys) and MyISAM (legacy, fast for reads, no transactions). You can choose the most suitable storage engine for your workload.
4) ACID Compliance and Transactions — InnoDB engine provides ACID compliance (Atomicity, Consistency, Isolation, Durability) for reliable transactions. Supports transaction control with START TRANSACTION, COMMIT, and ROLLBACK.
5) Advanced SQL Support — MySQL provides full support for standard SQL, including SELECT, INSERT, UPDATE, DELETE, JOINs (INNER, LEFT, RIGHT), GROUP BY, ORDER BY, subqueries, and views. On top of that, it also supports stored procedures, triggers, and user-defined functions for complex business logic.
6) Performance and Scalability — MySQL is optimized for high performance with advanced indexing, query optimization, and partitioning. It can handle large databases and high-concurrency workloads.
7) Replication and High Availability — MySQL supports various replication types: asynchronous, semi-synchronous, and group replication. It also supports automatic failover and high availability options, including InnoDB Cluster and managed services with automated failover.
8) Security and Access Control — MySQL supports role-based access control (RBAC), fine-grained privileges, SSL/TLS encryption, Transparent Data Encryption (TDE with InnoDB), password policies, pluggable authentication modules.
9) Backup, Recovery, and Monitoring — MySQL supports logical (mysqldump) and physical backups, point-in-time recovery with daily backups and write-ahead logs (in managed offerings).
10) Extensive Data Type Support — MySQL supports a wide range of data types: numeric, string, date/time, spatial, JSON, and more.
11) Community and Ecosystem — MySQL has a large, active community with many third-party tools, libraries, and integrations.
… and so much more!
Pros and Cons of MySQL:
MySQL Pros:
- MySQL is highly optimized for transactional workloads, fast point lookups, and single-row modifications.
- MySQL relatively easy to install, configure, and use.
- MySQL supports ACID transactions through the InnoDB storage engine, safeguarding data consistency during crashes.
- MySQL offers multiple storage engines (InnoDB, MyISAM, NDB Cluster) to match different workload patterns.
- MySQL provides master‑slave and group replication for high availability and basic failover setups.
- MySQL has a broad range of official connectors.
- MySQL comes with detailed manuals and a large user community, so finding help or examples is straightforward.
- MySQL scales well for small to medium workloads and supports both vertical scaling and basic horizontal replication.
- Major cloud platforms (AWS RDS, Azure Database for MySQL, Google Cloud SQL) offer managed MySQL instances, reducing operational overhead.
MySQL Cons:
- Poor analytical (OLAP) performance. Row-based storage and single-threaded query execution (largely) make complex analytical queries scanning large datasets very slow compared to columnar OLAP systems.
- MySQL lacks parallel query execution capabilities. It lacks the massive intra-query parallelism of dedicated OLAP databases like ClickHouse.
- MySQL lacks built-in parallel query execution, which can slow complex analytical workloads compared to some competitors.
- MySQL’s native JSON support offers limited query operations, no direct JSON indexing, and basic validation only.
- MySQL doesn’t provide auto‑sharding; scaling across many nodes requires manual sharding and extra orchestration.
- MySQL caps JSON document size at around 1 GB, which may not suit heavy document‑store use cases.
- Storage engines like MyISAM rely on table‑level locking, causing write bottlenecks when many clients update the same table.
- MySQL lacks some advanced development and debugging tools found in other RDBMS offerings.
- Its non‑standard GROUP BY handling can lead to unexpected query results without strict mode enabled.
ClickHouse vs MySQL: What's Best for You? Different Tools for Different Jobs.
Now lets compare ClickHouse vs MySQL. But note that directly comparing them as direct replacements is generally inappropriate due to their fundamentally different designs and target workloads.
ClickHouse vs MySQL—Architecture & Design Philosophy
🔮 | MySQL (InnoDB Focus) | ClickHouse (MergeTree Focus) |
---|---|---|
Primary Model | Relational Row-Store Database Management System (RDBMS). | Relational Column-Store Database Management System (DBMS). |
Storage Format | Rows stored contiguously in pages. Optimized for retrieving entire records quickly. | Columns stored contiguously. Optimized for scanning subsets of columns over many rows. High compression. |
Primary Use Case | OLTP: Transaction processing, application backends, operational data storage. | OLAP: Analytical processing, reporting, BI dashboards, log/event analysis. |
Architecture | Client-Server, Pluggable Storage Engines (InnoDB default). | Client-Server (Distributed), specialized analytical storage engines (MergeTree family). |
Updates/Deletes | Efficient synchronous operations for single rows via indexes. | Inefficient asynchronous background mutations (ALTER TABLE... ). Best for append-heavy. |
Transactions | Full ACID compliance via InnoDB (Atomicity, Consistency, Isolation, Durability). | Limited: Atomic single-statement operations. No multi-statement ACID guarantees. Eventual consistency for replicas. |
MySQL is built for transactional integrity and fast row-level access (OLTP). ClickHouse is built for analytical speed through columnar storage and processing (OLAP).
ClickHouse vs MySQL—Performance Characteristics
🔮 | MySQL (InnoDB Focus) | ClickHouse (MergeTree Focus) |
---|---|---|
Analytical Queries | Slow: Reads entire rows from disk/cache; limited parallelism; struggles with large scans/aggregations. | Extremely Fast: Reads only needed columns; vectorized execution; MPP; high compression reduces I/O. Designed for large scans/aggregations. |
Transactional Ops | Fast: Efficient point lookups (get row by ID), single-row INSERTs/UPDATEs/DELETEs using B+Tree indexes and buffer pool. | Slow: Inefficient point lookups (sparse primary index). Single-row modifications are heavy background operations. |
Data Loading | Efficient single-row INSERTs. Bulk loading (LOAD DATA INFILE ) is effective but can lock tables (MyISAM) or stress InnoDB. |
Very Fast Bulk Inserts: Optimized for ingesting large batches of data concurrently. Less efficient for frequent, small, single-row inserts. |
Concurrency (Workload) | High for OLTP: Handles many concurrent short transactions well (MVCC, row-locking). High analytical query load can cause blocking/resource contention. | High for OLAP: Handles many concurrent analytical queries efficiently. High concurrent OLTP-style write/update load is problematic. |
Query Execution | Primarily single-threaded per query (though some minor parallelism exists). Relies on index lookups. | Massively Parallel (utilizes multiple cores/SIMD instructions) and Vectorized (processes data in batches). |
Indexing | B+Trees: Excellent for fast point lookups and selective range scans. Clustered primary key. | Sparse Primary Index: Excellent for large range scans based on sort key. Data Skipping Indexes: Prune data blocks efficiently. No traditional secondary key lookups. |
Performance profiles are inverted. MySQL excels at transactions; ClickHouse excels at analytics. Using one for the other's job leads to poor performance.
ClickHouse vs MySQL—Scalability Approach
🔮 | MySQL | ClickHouse |
---|---|---|
Horizontal Scaling | Complex for Writes/Analytics: Typically requires manual application-level sharding or external tools. Read Scaling: Easily achieved via Read Replicas. | Natively Designed for Analytics: Built for horizontal scaling via sharding and replication to distribute analytical load across multiple nodes. |
Vertical Scaling | Supports scaling up a single server (more CPU, RAM, faster disk). Performance eventually plateaus. | Supports vertical scaling, but horizontal scaling is the primary method for handling massive analytical datasets and query volumes. |
Large Datasets (Analytic) | Performance degrades significantly on TB/PB scale for analytical queries without complex sharding/offloading strategies. | Designed to efficiently handle analytical queries on datasets ranging from GBs to PBs. |
ClickHouse scales analytical workloads horizontally much more effectively and naturally than MySQL. MySQL scales OLTP reads easily with replicas; scaling OLTP writes or analytics horizontally is a significant challenge.
ClickHouse vs MySQL—Data Handling & Features
🔮 | MySQL | ClickHouse |
---|---|---|
SQL Dialect | Standard SQL, high compatibility. | Extended SQL dialect with powerful analytical functions (aggregations, array/map manipulation, window functions). Some non-standard behavior/syntax. |
Data Types | Standard SQL types, JSON (improved in 8.x), Spatial. |
Standard types + specialized analytical types (LowCardinality , AggregateFunction , SimpleAggregateFunction , Nested , Map , Tuple , Enum , UUID , IPv4 /IPv6 ). Native JSON object. |
Replication | Mature options for OLTP high availability and read scaling: Asynchronous, Semi-Synchronous, Group Replication (InnoDB Cluster). | Asynchronous replication optimized for analytical consistency. Supports multi-master setups. Includes MaterializedMySQL engine to replicate directly from MySQL binlogs. |
Joins | Efficient standard SQL JOINs, optimized for relational integrity using indexes. | Supports various JOIN algorithms; performance depends heavily on JOIN type, data size, and distribution. Less optimized for large, distributed fact-fact joins than some MPP DWs. |
Materialized Views | Supported natively (MySQL 8+), but refresh management often requires manual triggers or scheduling and can be resource-intensive. | Core optimization feature. Often incrementally updated during data insertion via MergeTree engine mechanisms. Powerful for pre-aggregating data. |
MySQL provides standard SQL and features tailored for transactional consistency and relational integrity. ClickHouse offers specialized types, functions, and features (like MaterializedMySQL, advanced MVs) explicitly designed to optimize analytical query performance.
When Would You Choose MySQL vs ClickHouse?
Choose MySQL if:
- You are building the backend for an operational application (like e-commerce site, CRM, CMS....) requiring transactional integrity (OLTP).
- ACID compliance is a strict requirement for data consistency.
- Your application involves frequent single-row inserts, updates, and deletes.
- Fast point lookups (retrieving individual records by primary key) are essential.
- Your team needs standard SQL and the stability of a mature RDBMS ecosystem.
- You need straightforward replication for read scaling or high availability in a transactional context.
Choose ClickHouse if:
- Your main goal is fast analytical query performance (OLAP) on large datasets.
- You are building real-time dashboards, reporting tools, log analysis platforms, or time-series databases.
- Queries primarily involve aggregating data or scanning large subsets of columns.
- The dominant write pattern is large batch inserts (append-heavy).
- High data compression is important for managing storage volume and cost.
- Horizontal scalability for analytical performance across many nodes is required.
- Data is largely immutable, or updates/deletes are infrequent and can be handled as background batch operations.
- You need to run analytics without impacting your primary OLTP database.
TL;DR: MySQL is not a viable ClickHouse alternative for analytics, nor is ClickHouse suitable for transactional workloads typically handled by MySQL. They are fundamentally different tools designed for different purposes. They are best used together: MySQL managing live operational data, and ClickHouse powering fast analytics on replicated data.
Continue reading...
ClickHouse Alternative 6—PostgreSQL
Like MySQL, PostgreSQL is primarily an OLTP workhorse but with distinct features, particularly its extensibility and robust support for complex data types like JSONB and geospatial data.
PostgreSQL, often just called Postgres, is a powerful, open source Object-Relational Database Management System (ORDBMS). It's earned a great reputation for being reliable, robust, feature-rich, and extensible, plus it sticks to SQL standards. While MySQL focuses on being simple and fast for common web work, PostgreSQL handles complex queries, varied data types (like JSONB and geospatial data with PostGIS), and keeps data integrity high. It works on almost all major operating systems and supports a wide range of applications.
PostgreSQL in 100 Seconds - ClickHouse Alternatives - ClickHouse Competitors
PostgreSQL Architecture—Process-Oriented, Extensible, and ACID-Compliant
PostgreSQL employs a multi-process client-server architecture, distinct from MySQL's often thread-based model. This process isolation contributes to stability but can have different resource implications under high connection counts.
PostgreSQL Architecture Diagram - ClickHouse Alternatives - ClickHouse Competitors
➥ Process Model
PostgreSQL uses a multi-process model. The main types of processes are:
a) Postmaster (Main Server Process) — Postmaster is the main coordinating process that starts when the server starts. It listens for client connections, authenticates them, and then forks a new backend process to handle each connection.
b) Backend Processes (postgres
) — Each client connection interacts exclusively with its own backend process. This process parses, plans, and executes the client's SQL queries, manages transaction state for that connection, reads data pages into shared memory (or its local memory), and writes changes via Write-Ahead Logging. This process-per-connection model provides strong isolation.
c) Background Processes — Alongside the postmaster
and backend processes, several background auxiliary processes for maintenance and performance:
- Checkpointer — Periodically writes dirty data pages from memory to disk, creating checkpoints to gurantee database consistency.
- Background Writer(BgWriter) — Also writes dirty pages from shared buffers to disk, but less aggressively than the checkpointer, reducing load during peak times.
- WAL Writer — Writes Write-Ahead Logging (WAL) records from memory buffers to persistent storage.
- Autovacuum Launcher — Starts worker processes to clean up dead rows left by updates or deletes and update statistics for the query planner.
➥ Memory Architecture
PostgreSQL uses memory divided into two main categories: shared memory and local memory.
a) Shared Memory — Shared memory area is accessible by the postmaster and all backend and background processes. Major components include:
- Shared Buffers (shared_buffers) — This is the main cache for table and index data pages read from disk. It reduces disk I/O by keeping frequently accessed data in memory.
- WAL Buffers — Temporarily holds transaction log records (changes) before the WAL Writer process writes them to permanent WAL files.
- Other areas manage locks, prepared transactions, and process information.
b) Local Memory — Each backend process allocates its own local memory for processing queries. Key areas include:
- Work Memory (work_mem) — Used for internal sort operations, hash tables (used in hash joins), and bitmap operations. Queries requiring large sorts or hashes might use significant
work_mem
. - Maintenance Work Memory (maintenance_work_mem) — A separate, usually larger, memory area allocated for maintenance tasks like
VACUUM
,CREATE INDEX
, andALTER TABLE ADD FOREIGN KEY
. - Temporary Buffers (temp_buffers) — Caches temporary tables used during complex query processing.
➥ Storage Architecture
PostgreSQL stores data persistently on disk within a defined data directory (PGDATA
).
- Data Files — Tables and indexes are stored as files within the operating system's filesystem.
- Tablespaces — Allow database administrators to define specific locations on the filesystem where database object files (like tables and indexes) should be stored. This helps manage storage across different physical drives.
- Pages — Data is stored in fixed-size blocks, usually 8KB, called pages. Tables are collections of these pages (heap pages).
- Write-Ahead Logging (WAL) — PostgreSQL first writes all database changes to WAL files before modifying the actual data files, which makes sures data durability and consistency; if the server crashes, the database can be recovered by replaying the WAL entries since the last checkpoint.
- TOAST (The Oversized Attribute Storage Technique) — Handles large data values (like text or byte arrays) that don't fit directly onto a single data page.
➥ Query Processing Lifecycle
When a user sends an SQL query to PostgreSQL, the connected backend process handles it through several stages:
- First, the parser checks the query's syntax and creates a parse tree.
- The system analyzes the parse tree, validating object names and data types. The rewrite system applies any rules (e.g: expanding views into their underlying table queries). This results in a query tree.
- The planner (or optimizer) generates potentially multiple ways (plans) to execute the query tree. It estimates the cost of each plan based on database statistics, available indexes, and configuration parameters. It selects the plan with the lowest estimated cost. The output is a plan tree.
- The executor takes the chosen plan tree and recursively processes its nodes. Nodes might involve scanning tables, joining data, sorting results, or aggregating data. The executor retrieves data pages from the shared buffer pool or reads them from disk if not buffered. It uses Multiversion Concurrency Control (MVCC) to ensure each transaction sees a consistent snapshot of the data, allowing concurrent operations without read locks blocking write locks, and vice versa.
- Finally, the executor sends the resulting rows back to the client.
PostgreSQL Key Features
PostgreSQL is renowned for its extensive feature set:
1) ACID compliance & MVCC — PostgreSQL implements full ACID transactions using multiversion concurrency control (MVCC), letting readers and writers run in parallel without blocking each other.
2) Extreme Extensibility — A core design philosophy. Postgres allows adding user-defined data types, functions (in multiple languages like SQL, PL/pgSQL, Python, C), operators, index access methods, and foreign data wrappers. Enables powerful extensions like PostGIS (geospatial), Citus (distributed), TimescaleDB (time-series) 👈 we will cover this later.
3) Rich Data Types—Postgres has extensive built-in data types including numerics, text, date/time, boolean, UUID, network addresses, geometric types, arrays, range types, and highly capable JSON/JSONB support (with indexing).
4) Advanced Indexing (Six index methods)—Postgres supports six indexing methods : B-Tree (default), Hash, GiST (Generalized Search Tree - for indexing complex types like geometric or text), SP-GiST (Space-Partitioned GiST), GIN (Generalized Inverted Index - for indexing elements within composite types like JSONB or arrays), and BRIN (Block Range Indexes - for very large, linearly correlated data). Supports expression indexes and partial indexes as well.
5) WAL‑based replication—PostgreSQL ships write‑ahead log (WAL) records to replicas asynchronously, enabling read scaling on standby nodes.
6) Synchronous & logical replication — You can require at least one standby to confirm each transaction or use logical replication for selective table syncs or zero‑downtime upgrades.
7) Parallel query execution — PostgreSQL can split large queries across CPU cores for faster analytics.
8) Declarative partitioning — PostgreSQL supports native range, list and hash partitioning to manage large tables efficiently.
9) Just‑In‑Time (JIT) compilation — With LLVM integration, PostgreSQL can compile hot query paths at runtime to speed up complex expressions
10) Rich native types — You get JSON/JSONB, XML, arrays (up to 1 GB), HStore, UUID, enums, geometric and network address types out of the box.
11) Extension framework — PostgreSQL lets you install community modules like PostGIS, TimescaleDB or PL/V8 without rebuilding the core.
12) Security — PostgreSQL provides role-based access control, row-level security (RLS) policies, SSL/TLS connections, various authentication methods (SCRAM, LDAP, PAM, etc.).
…and so much more!
Pros and Cons of PostgreSQL:
PostgreSQL Pros:
- PostgreSQL handles complex transactions, data integrity constraints, varied data types, and object-relational features very effectively.
- PostgreSQL uses MVCC to handle concurrent reads and writes without blocking.
- PostgreSQL lets you define custom data types, functions, and operators via extensions.
- PostgreSQL provides excellent support for complex types like JSONB (with GIN indexing), Arrays, Geospatial.
- PostgreSQL offers advanced index types such as GiST and GIN.
- PostgreSQL includes streaming and logical replication for high availability.
- PostgreSQL runs on Windows, Linux, macOS, and other Unix variants.
PostgreSQL Cons:
- Just like MySQL, the core row-oriented storage makes analytical queries that scan large portions of a few columns very inefficient compared to ClickHouse.
- PostgreSQL lacks managed‑hosting coverage on certain providers by default.
- PostgreSQL extension docs mainly appear in English.
- Major version upgrades often require a dump/restore cycle.
- PostgreSQL carries a steeper learning curve for new users.
- PostgreSQL demands careful tuning of numerous configuration parameters.
- PostgreSQL requires periodic VACUUM (mostly handled by autovacuum) to prevent table bloat.
- PostgreSQL has a bit more complex initial setup compared to simpler databases.
- PostgreSQL requires careful tuning of numerous configuration parameters (
shared_buffers
,work_mem
, checkpoint settings, autovacuum, ... etc.) for optimal performance.
Is ClickHouse better than PostgreSQL?
Similar to the MySQL comparison, PostgreSQL and ClickHouse aren't direct competitors, really. They're suited for different things, which makes comparisons tough. It's like weighing the strengths of OLTP against OLAP.
ClickHouse vs PostgreSQL—Which is Right for You?
Choosing between them boils down to understanding what you need your database to do. Are you building a standard web application needing reliable transaction handling, or are you crunching massive datasets for analytics?
ClickHouse vs PostgreSQL—Architecture & Design Philosophy
🔮 | PostgreSQL | ClickHouse |
---|---|---|
Main Model | Object-Relational Row-Store Database Management System (ORDBMS). | Columnar / Relational Column-Store Database Management System (DBMS). |
Storage Format | Rows stored contiguously in pages (Heap tables). Optimized for accessing/modifying entire records. TOAST for large objects. | Columns stored contiguously. Optimized for scanning subsets of columns. High compression. |
Primary Use Case | OLTP: Complex applications, system of record, data integrity focus. Geospatial, JSON document store. | OLAP: Real-time analytics, reporting, BI speed layer, log/event analysis. |
Concurrency | MVCC for high concurrent OLTP operations (reads don't block writes, writes don't block reads). | Lock-free reads; optimized for high concurrent analytical queries. |
Transactions | Full ACID Compliance. Mature transaction management. | Limited: Atomic single-statement operations. No multi-statement ACID. Eventual consistency for replicas. |
Extensibility | Very High: Core design principle. Extensive extension framework (PostGIS, TimescaleDB, Citus, FDWs, etc.). | Moderate: Supports UDFs (SQL, JS, executable), external dictionaries/models. Less focus on core engine extensions. |
PostgreSQL is a highly extensible, ACID-compliant ORDBMS optimized for complex OLTP and diverse data types. ClickHouse is a specialized columnar database hyper-optimized for OLAP query speed and efficiency.
ClickHouse vs PostgreSQL—Performance Characteristics
🔮 | PostgreSQL | ClickHouse (MergeTree Focus) |
---|---|---|
Analytical Queries | Slow to Moderate: Row reads are inefficient for large scans. Parallel query improves performance but is generally much slower than ClickHouse on large datasets. | Extremely Fast: Columnar storage, vectorized execution, MPP architecture deliver top-tier OLAP performance. |
Transactional Ops | Fast: Efficient single-record operations (INSERT, UPDATE, DELETE, SELECT by PK/indexed column) using B-Tree indexes and MVCC. | Slow: Point lookups are inefficient. Updates/Deletes are heavy background ALTER mutations. Not suitable for OLTP writes. |
Data Modification | Efficiently handles frequent updates/deletes via MVCC and VACUUM mechanism. | Less efficient for modifications. Optimized for immutable data or large, infrequent batch updates/deletes. |
Query Execution | Primarily row-by-row processing engine with added parallel capabilities for specific operations (scans, joins, aggregates). Sophisticated optimizer. JIT compilation option. | Vectorized (batch processing using SIMD) and Massively Parallel (multi-core, multi-node). Simpler optimizer focused on columnar operations. |
Indexing | Rich & Diverse: B-Tree, Hash, GIN (JSONB/Arrays), GiST (Spatial/Complex), BRIN (Large ordered data). Excellent for varied OLTP and specific data type queries. | Specialized for OLAP: Sparse Primary Index (sorting/range scans), Data Skipping Indexes (block filtering). Not designed for fast OLTP-style point lookups. |
ClickHouse offers vastly superior performance for OLAP workloads. PostgreSQL excels at transactional performance and queries leveraging its diverse indexing capabilities (like fast JSONB or geospatial searches).
ClickHouse vs PostgreSQL—Scalability Approach
🔮 | Core PostgreSQL | ClickHouse |
---|---|---|
Horizontal Scaling | Limited (Core): Read scaling via replicas. Write/analytic scaling typically needs partitioning or extensions like Citus Data for true distribution. | Natively Designed for Analytics: Built for horizontal scaling via sharding and replication across nodes to distribute analytical load. |
Vertical Scaling | Common approach; scales well vertically up to a point. | Supports vertical scaling, but horizontal scaling is key for massive data/query volume in OLAP scenarios. |
Large Datasets (Analytic) | Core engine struggles with performance on TB/PB analytical queries. Requires partitioning or extensions (Citus/TimescaleDB) for efficiency at scale. | Designed to efficiently handle analytical queries on datasets from GBs to PBs across a distributed cluster. |
ClickHouse scales analytical workloads horizontally by design. Core PostgreSQL relies more on vertical scaling, replication, and partitioning; significant horizontal scaling for analytics typically requires extensions like Citus.
ClickHouse vs PostgreSQL—Data Handling & Features
🔮 | PostgreSQL | ClickHouse |
---|---|---|
SQL Support | High ANSI SQL Compliance. Rich feature set including advanced window functions, CTEs, robust JOIN capabilities. | Extended SQL dialect optimized for analytics; powerful functions for arrays, maps, strings, approximate calculations. Some non-standard syntax/behavior. |
Data Types | Rich built-in types (Numeric, Text, Date/Time, etc.), User-Defined Types, excellent JSONB , Array , Range , UUID , Network types. Geospatial via PostGIS extension. |
Wide range including specialized analytical types (LowCardinality , AggregateFunction , SimpleAggregateFunction , Nested , Map , Tuple , Enum , UUID , IPv4 /IPv6 ). Native JSON object. |
Indexing | Extensive options: B-Tree, Hash, GIN (arrays, JSONB, text), GiST (spatial, complex), BRIN (large ordered data). Expression/Partial indexes. | Specialized for OLAP: Sparse Primary Index (sorting/ranges), Data Skipping Indexes (min/max, set, bloom, etc. for block filtering). |
Data Consistency | Strong ACID Compliance. | Eventual consistency for replicas; No multi-statement ACID guarantees. Focus on analytical query consistency. |
Compression | TOAST for large values; Filesystem compression or ZFS often used. TimescaleDB adds columnar compression. | Excellent, tunable native columnar compression (LZ4, ZSTD, Delta, Gorilla, etc.). |
Materialized Views | Supported; refresh typically requires manual scheduling (REFRESH MATERIALIZED VIEW ) or triggers. |
Supported; Core optimization feature. Often incrementally updated during data insertion via MergeTree engine capabilities or specific MV types (e.g: Aggregate ). |
Replication | Built-in physical streaming (async/sync) and logical replication. | Built-in asynchronous replication (ReplicatedMergeTree ). Can replicate from PostgreSQL via FDWs or CDC tools. |
PostgreSQL offers superior data type flexibility (esp. JSONB, GIS), diverse indexing for OLTP, and strong SQL compliance with full ACID. ClickHouse provides specialized types and functions for analytics, superior native compression, and more integrated/efficient Materialized Views for OLAP optimization.
When Would You Choose PostgreSQL vs ClickHouse?
Choose PostgreSQL if:
- You need a highly reliable transactional database (OLTP) with strict ACID guarantees.
- Your application demands complex relational modeling, foreign keys, triggers, and stored procedures.
- You require strong support for diverse data types like
JSONB
(with indexing) or Geospatial data (via the PostGIS extension). - Your queries benefit significantly from PostgreSQL's advanced indexing strategies (GIN, GiST, BRIN).
- SQL standards compliance and a mature, stable ecosystem are critical.
- Your primary scaling strategy involves vertical scaling or simple read replicas for OLTP workloads.
Choose ClickHouse if:
- Your primary goal is extreme speed and low latency for analytical queries (OLAP) on large datasets.
- You are building real-time analytics dashboards, log/event processing systems, or BI backends requiring fast aggregations.
- Queries predominantly involve scanning large portions of a few columns.
- The main write pattern is large batch inserts (append-heavy).
- High data compression and storage efficiency for analytical data are the highest priorities.
- Native horizontal scalability across many servers for analytical throughput is essential.
- Data is largely immutable, or updates/deletes are infrequent background operations.
In Short: PostgreSQL is good at handling complex transactions and a wide range of data types. However, when it comes to big data analytics, ClickHouse truly delivers. ClickHouse's speed and scalability make it an excellent choice for specific use cases, as it was designed from the ground up for OLAP. In many situations, the two systems can work together effectively.
Continue reading...
🚀 ClickHouse Alternatives: Category 3—ClickHouse vs Direct Real-time OLAP Competitors
Okay, now we now enter the territory of databases designed with goals very similar to ClickHouse: achieving extremely fast analytical query performance on large datasets, often ingested in real-time. These systems directly compete with ClickHouse for use cases like real-time analytics, observability platforms, operational intelligence, and user-facing interactive dashboards. First in this category is Apache Druid.
ClickHouse Alternative 7—Apache Druid
Apache Druid is a high-performance, open source, distributed real-time analytics database. It's specifically engineered for rapid "slice-and-dice" analytics (filtering, grouping by dimensions, and aggregating metrics) on massive datasets, particularly excelling with event-driven and time-series data. Apache Druid's core design priorities are low-latency data ingestion (both streaming and batch) and sub-second query response times for interactive analytical exploration.
What is Apache Druid - ClickHouse Alternatives - ClickHouse Competitors
Apache Druid Architecture
Apache Druid uses a distributed, microservice-based architecture where distinct processes handle specialized roles. This design allows for independent scaling of different functions (ingestion, querying, coordination, data storage) but introduces higher operational complexity compared to more monolithic designs.
Here is the detailed breakdown of Apache Druid architecture:
Apache Druid splits its functionality across several service types to isolate responsibilities and scale each function independently:
- Coordinator — Manages segment availability and balances segments across Historical nodes.
- Overlord — Schedules ingestion tasks on MiddleManagers and coordinates segment publishing.
- Broker — Receives client queries, routes subqueries to data nodes, merges results, and returns the final output.
- Router — Acts as the API gateway for all external requests and hosts the web console.
- Historical — Loads committed segments from deep storage, caches them locally, and serves queries on read-only data.
- MiddleManager & Peon — MiddleManagers spawn Peon processes to read external sources, build new segments, and publish them.
- Indexer (experimental) — Runs ingestion tasks as threads in a single JVM instead of separate Peon processes for simpler deployment and resource sharing.
➥ Coordinator and Overlord
Coordinator services watch Historical nodes, assign segments to specific servers, and rebalance segments to keep work even. Overlord services monitor MiddleManagers, assign ingestion tasks, and manage segment publication to deep storage and metadata store.
➥ Broker and Router
Brokers handle queries from clients, determine which segments satisfy the query, fan out subqueries to Historical or real‑time nodes, and merge partial results. Routers provide a single endpoint for queries, coordinators, and overlords, simplifying client connections and running the Apache Druid web console.
For Data Ingestion and Storage:
➥ Historical
Historical nodes download segments from deep storage, cache segments on local disk and in memory, and serve queries on immutable, committed data.
➥ MiddleManager and Peon
MiddleManagers read from streaming or batch sources, spawn Peon JVMs for each ingestion task, build segments, and push them to deep storage and metadata storage.
➥ Indexer (Optional)
Indexer services run ingestion tasks as threads within a single JVM, offering a simpler setup and better resource sharing than the MiddleManager + Peon model. They remain experimental but can streamline streaming workloads.
For External Dependencies:
Apache Druid relies on external systems for crucial functions:
➥ Deep Storage
Apache Druid uses deep storage (such as Amazon S3, HDFS, or network file systems) to store all ingested segments for backup, recovery, and segment transfer between cluster nodes.
➥ Metadata Storage
Apache Druid keeps system metadata—including segment usage and task information—in a relational database like PostgreSQL, MySQL, or Derby.
➥ ZooKeeper
Apache ZooKeeper provides service discovery, coordination, and leader election for Druid processes, ensuring cluster state consistency.
Apache Druid Key Features
1) Low-Latency Interactive Queries — Apache Druid is designed for sub-second responses for OLAP queries (filtering, grouping, especially TopN and time-based aggregations).
2) Real-time & Batch Ingestion — Apache Druid native connectors for streaming sources (Kafka, Kinesis) allow data to be queryable within seconds. Also supports batch ingestion from Deep Storage (S3, HDFS, etc.).
3) Columnar Storage & Optimization — Apache Druid stores data columnarly within segments. It uses dictionary encoding for string dimensions, bitmap indexes for fast filtering, and various compression techniques.
4) Optional Ingestion-Time Roll-up — Apache Druid pre-aggregates data to reduce storage footprint and accelerate queries on summarized data.
5) Distributed & Scalable Architecture — Microservice architecture of Druid allows independent scaling of ingestion, query serving, and data management by adding nodes of specific types.
6) Elastic scaling and self‑healing — You can add or remove nodes on the fly; the cluster rebalances itself and recovers from failures without downtime.
7) Interactive query engine — Apache Druid uses a scatter/gather query engine that preloads segments in memory or local storage, delivering sub‑second query responses.
8) Druid SQL Interface — Apache Druid provides a SQL query layer (using Apache Calcite) over its native JSON-based API, enabling use with standard SQL tools (with some limitations).
9) High Availability & Fault Tolerance — Apache Druid relies on deep storage for segment durability, replication of segments across Historicals, and ZooKeeper for coordination to handle node failures.
10) Data sketches and approximate algorithms — Apache Druid supports approximate query processing using libraries like Apache DataSketches for fast estimations of distinct counts, quantiles, etc., on high-cardinality data.
11) High availability — Apache Druid supports multi‑node replication, continuous backups, and automated recovery to keep data available and durable.
… and so much more!
Pros and Cons of Apache Druid:
Apache Druid Pros:
- Apache Druid provides real‑time analytics with sub‑second queries on streaming and batch data
- Apache Druid delivers low latency that suits interactive dashboards and ad hoc queries
- Apache Druid scales horizontally to handle growing data volumes and concurrent queries
- Apache Druid uses columnar storage with indexing and pre‑aggregation for efficient scans
- Apache Druid supports flexible ingestion for both real‑time streams and batch loads
- Apache Druid integrates with cloud storage, prefetching segments to cut storage costs
- Apache Druid offers data sketching tools for approximate counts and fast aggregation on high‑cardinality data
- Apache Druid maintains high availability with near‑zero planned downtime and continuous backup
- Apache Druid uses a share‑nothing architecture to isolate failures and separate compute from storage
Apache Druid Cons:
- Apache Druid has a complex setup requiring multiple services and external systems
- Apache Druid offers weak JOIN support
- Apache Druid lacks native support for updates and deletes, making data mutations tricky
- Its performance trails newer OLAP databases on some benchmarks
- Apache Druid carries operational overhead in cluster management and task troubleshooting
- Apache Druid involves complex security configuration that can lead to misconfigurations
- Apache Druid limits cloud elasticity since data must load locally before queries.
- Druid SQL is powerful but not fully ANSI SQL compliant; certain complex queries, functions, or join types may not be supported or perform well
- Apache Druid demands high resources for indexing tasks and segment storage
- Apache Druid faces challenging upgrades and migrations due to interdependent services
- Apache Druid has a smaller ecosystem compared to alternatives like ClickHouse or Apache Pinot
- Apache Druid is fast for its target queries, but it can be slower than ClickHouse for full scans, complex aggregations, or queries not aligning well with its indexing/data model.
ClickHouse vs Druid: What's Best for You?
Apache Druid is a very direct competitor to ClickHouse in the real-time analytics space. Here’s how they compare:
We'll look at how they're built, how they perform, how they scale, and where each one really shines.
ClickHouse vs Druid—Architecture & Complexity
🔮 | Apache Druid | ClickHouse |
---|---|---|
Architecture Overview | Microservices-based: Specialized node roles (Coordinator, Overlord, Broker, Historical, Ingest). Distributed coordination. | Simpler Core: Server process handles multiple roles. Distributed via clustering (shards/replicas). Relies on internal Keeper/ZooKeeper for replication. |
Storage/Compute | Separated: Compute on Historicals/Ingest nodes. Persistence via external Deep Storage (S3, HDFS). Local caching. | Flexible: Coupled (OSS default, shared-nothing) or Separated (ClickHouse Cloud / Object Storage config using internal mechanisms). |
Data Organization | Datasources -> Time-partitioned immutable Segments (columnar). Mandatory timestamp. | Tables -> Mutable Data Parts (columnar, sorted by primary key, merged in background). |
Complexity | High: Multiple service types + mandatory external dependencies (ZK, Metadata DB, Deep Storage) increase operational burden. | Lower (Core): Simpler initial setup. Distributed cluster configuration/tuning still requires expertise. |
Dependencies | High: Requires managing ZooKeeper, Relational DB (Metadata), and Deep Storage infrastructure externally. | Lower: Fewer external dependencies for core operation (ZK needed for replication). |
Indexing | Automatic Bitmap Indexes: Creates indexes (dictionary encoded bitmaps) automatically per dimension column. | Manual Configuration: Sparse Primary Key Index (sorting) + optional secondary Data Skipping Indexes require schema definition. |
Apache Druid's microservices offer granular scaling but demand significantly more operational management due to complexity and external dependencies. ClickHouse has a simpler core architecture but relies on careful configuration and its internal replication (ReplicatedMergeTree
) for HA/scaling.
ClickHouse vs Druid—Performance Profile
🔮 | Apache Druid | ClickHouse |
---|---|---|
Query Speed (OLAP) | Excellent (Targeted): Sub-second for interactive slice-and-dice, time filters, TopNs. Optimized for filtering via bitmap indexes. | Excellent (Broad): Extremely fast across a wider range of OLAP queries including large scans, complex aggregations, JOINs. Often lower latency on computationally heavy queries. |
Ingestion Speed & Latency | Excellent (Streaming): Optimized for native Kafka/Kinesis ingestion with data queryable in seconds (exactly-once possible). Good batch support. | Excellent (Batch): Optimized for very high-throughput batch loads. Streaming via Kafka engine is efficient micro-batching (seconds/low-minutes latency). |
Joins | Very Limited: Primarily supports broadcast lookup joins (small dimension table to large fact table). Requires denormalization for most other cases. | More Robust: Supports standard SQL JOIN types (INNER, LEFT, RIGHT, FULL). Performance varies, but significantly more capable than Druid. Denormalization still sometimes advised. |
Concurrency | Very High: Designed to handle thousands of concurrent interactive queries efficiently. | Very High: Handles high query concurrency well; specific limits depend on configuration and resources. |
Data Updates/Deletes | Impractical: Segments are immutable. Requires re-indexing/re-ingestion of entire time chunks. | Inefficient: Supported via asynchronous ALTER TABLE... mutations (background rewrites). Not suitable for frequent updates but possible for corrections. |
Approximate Queries | Strong: Native support for Apache DataSketches as metric types for fast approximations. | Strong: Provides aggregate functions for approximate calculations. |
Apache Druid excels at low-latency interactive queries on real-time streams, especially time-based filtering and TopNs, leveraging bitmap indexes and optional roll-up. ClickHouse offers superior performance across a broader range of complex OLAP queries, including better JOIN support and handling large scans/aggregations, and is exceptionally fast for batch loads.
ClickHouse vs Druid—Scalability & Operations
🔮 | Apache Druid | ClickHouse |
---|---|---|
Horizontal Scaling | Granular: Scale different node types (Broker, Historical, Ingest) independently based on bottlenecks. Automatic segment rebalancing. | Node-based: Scale by adding nodes (shards/replicas). Requires config updates. Manual data rebalancing often needed for sharding changes (OSS). |
Storage/Compute Scaling | Independent: Add query/historical nodes without changing deep storage. Storage scales with deep storage provider (e.g: S3). | Flexible: Coupled (OSS default) scales together. Decoupled (Cloud/Object Storage) allows independent scaling similar to Druid's concept. |
Ease of Scaling | Adding nodes is technically simple, but managing the state and performance of the complex, multi-component system can be challenging. | Adding nodes requires configuration changes and potentially manual data rebalancing (OSS). Managed Cloud services significantly simplify scaling. |
Operational Overhead | High: Managing multiple service types, JVM tuning, plus external dependencies (ZK, Metadata DB, Deep Storage) is operationally intensive. | Moderate-to-High (OSS): Simpler core, fewer dependencies, but distributed setup, tuning, and upgrades still require expertise. Low (Cloud): Managed service. |
Apache Druid offers more granular scaling due to its microservice architecture and inherent storage/compute separation via deep storage but comes with much higher operational complexity. ClickHouse scaling is effective, potentially less granular (OSS), but the overall system is often simpler to manage, especially with managed services.
ClickHouse vs Druid—Data Ingestion & Querying
🔮 | Apache Druid | ClickHouse |
---|---|---|
Real-time Ingestion | Native Streaming: Low latency (seconds), high throughput, exactly-once possible via Kafka/Kinesis indexing service. Queryable immediately. | Near Real-time (Micro-batch): Kafka engine provides efficient micro-batching (seconds-to-minutes latency). |
Batch Ingestion | Supported from Deep Storage (S3, HDFS). Can be complex to configure optimal batch tasks. | Primary Strength: Extremely fast and efficient parallel batch ingestion from various sources and formats. |
Ingestion Transformations | Supports filtering, transformations, and optional roll-up (pre-aggregation) during ingestion. | Supports transformations via SQL INSERT INTO ... SELECT ... or input functions. Pre-aggregation typically handled via Materialized Views (post-ingestion). |
Schema Handling | More flexible; can often handle evolving schemas dynamically during ingestion. | Typically requires predefined schema. ALTER TABLE operations possible but can be heavy. |
Query Language | Druid SQL (via Apache Calcite, good coverage but some limitations) or Native JSON DSL. | ClickHouse SQL (Extended standard SQL dialect, very powerful analytical functions). |
Nested Data | Limited native support; often requires flattening complex structures into separate dimensions during ingestion. | Excellent native support: Nested data type, Array , Map , Tuple , JSON object type with powerful query functions. |
Apache Druid excels at native, low-latency streaming ingestion with optional pre-aggregation (roll-up). ClickHouse is exceptionally strong at batch ingestion and offers more powerful SQL, including better JOINs and superior handling of complex/nested data types.
When Would You Choose Druid vs ClickHouse?
Choose Apache Druid if:
- Your absolute priority is sub-second query latency for user-facing interactive dashboards heavily focused on time-based filtering, slicing/dicing dimensions, and TopN results.
- You require true real-time ingestion from Kafka/Kinesis where data must be queryable within seconds of arrival.
- Ingestion-time roll-up (pre-aggregation) is acceptable and beneficial for reducing data volume and accelerating queries on summarized views.
- You need granular, independent scaling of ingestion, querying, and historical data nodes and have the operational capacity to manage a complex distributed system with external dependencies (ZK, Metadata DB, Deep Storage).
- Your query patterns align well with Druid's strengths (filtered aggregations) and its JOIN limitations are acceptable (or data can be denormalized).
Choose ClickHouse if:
- You need the fastest possible performance across a broader range of complex OLAP queries, including large table scans, heavy aggregations, and more complex SQL JOINs.
- High-throughput batch ingestion is your primary data loading method, or near-real-time via efficient micro-batching (Kafka engine) meets requirements.
- You require powerful and flexible SQL capabilities, including robust JOIN support and advanced functions for handling complex or nested data types (Arrays, Maps, JSON).
- You prefer a relatively simpler core architectural design with fewer external dependencies, accepting that distributed setup still requires expertise (especially if self-hosting).
- Cost-efficiency through superior data compression and high performance per resource unit is a major factor.
- You are building analytical backends for general BI tools, log analysis platforms requiring deep querying, or data warehousing tasks beyond basic dashboards.
TL;DR: Apache Druid is a database built for real-time analytics and super-fast querying of streaming data, especially time-series data or data with events. It can scale really precisely but it's a bit of a hassle to manage. ClickHouse, on the other hand, handles a lot more types of queries and loads of data, often faster than Druid. It's also got more advanced SQL features and data type support, not to mention a simpler underlying architecture. That makes it a really versatile and high-performance engine for analyzing data.
Continue reading...
ClickHouse Alternative 8—Apache Pinot
Apache Pinot is an open source, distributed, real-time analytical database engineered specifically for scenarios demanding ultra-low query latency (often milliseconds) at very high query throughput (thousands of queries per second). It's frequently chosen for user-facing analytical applications, such as real-time dashboards, personalization engines, or anomaly detection systems where responsiveness is critical. Apache Pinot began inside LinkedIn in 2013 and went open source in June 2015 under Apache 2.0 license.
Apache Pinot 101 - ClickHouse Alternatives - ClickHouse Competitors
Apache Pinot Architecture
Apache Pinot Architecture organizes a distributed OLAP database into four key components—Controller, Broker, Server, and Minion—to deliver low‑latency, high‑concurrency analytics. Apache Pinot uses Apache Helix with ZooKeeper for cluster management and stores data in immutable columnar segments optimized with multiple index types. Brokers route queries to server nodes for parallel execution and merge results before returning.
[Apache Pinot Architecture Diagram]Alt: ClickHouse Alternatives - ClickHouse Competitors - Online Analytical Processing - OLAP System - ClickHouse vs Pinot
Here is the detailed component breakdown of Apache Pinot's architecture:
➥ Controller
Controllers is the brain of the cluster. It manage the overall cluster state, metadata (schemas, table configurations), segment assignments to Servers, and coordinates tasks. It utilizes Apache Helix for distributed coordination and state management, which in turn relies on Apache ZooKeeper. Controllers provide REST APIs for cluster administration, schema management, and table configuration.
➥ Broker
Brokers receive queries from clients (via SQL or PQL). It consults routing information (managed by Helix/Controller) to determine which servers host the relevant data segments for the query's scope. It scatters query fragments to the appropriate Servers, gathers partial results, merges them, and returns the final response. Brokers are stateless and horizontally scalable.
➥ Server
Servers stores data segments locally and executes query fragments received from Brokers. There are traditionally two types, though they can be combined in a single process (Hybrid):
- Realtime Servers — Primarily ingest data directly from streaming sources (like Apache Kafka, Kinesis, Pulsar). They build segments in memory based on the incoming stream, making data queryable quickly, and periodically persist these segments.
- Offline Servers — Load pre-built, immutable segments generated by external batch processing jobs (e.g: Spark, Hadoop) from Deep Storage. They serve queries against this historical batch data.
➥ Minion(Optional)
Minion is a framework for running background operational tasks on Pinot segments, such as segment merging, purging expired data according to retention policies, or building indexes offline, without impacting the real-time ingestion or query paths.
For Data Model and Storage:
Apache Pinot breaks tables into segments, which are columnar files that store rows for specific time or partition ranges. It uses bitmap, inverted, star‑tree, and range indexes inside segments to speed up filtering, aggregation, and group‑by operations. The system treats segments as immutable units, simplifying replication and recovery.
➥ Hybrid and Table Types
Apache Pinot supports offline tables for batch data and realtime tables for streaming ingest, and merges both in hybrid tables. Hybrid queries combine segments from both table types at query time.
For Cluster Management:
Apache Pinot embeds Apache Helix to manage partition assignments, replication, and node failures. Helix stores cluster state in ZooKeeper and triggers rebalance when servers join or leave. This setup removes single points of failure and scales horizontally by adding more nodes.
Query Flow
- Client sends SQL/PQL query to a Broker.
- Broker identifies target Servers and segments based on routing data.
- Broker sends query fragments to relevant Servers.
- Servers execute fragments using local segments and indexes, returning partial results.
- Broker merges partial results and sends the final response to the client (this process is optimized for millisecond latency).
Apache Pinot Key Features
Pinot is engineered for specific low-latency, high-throughput scenarios:
1) Real‑time and Batch Ingestion — Apache Pinot is optimized low-level consumers for streaming sources (Kafka, Kinesis, Pulsar) enabling data visibility often within seconds. It also supports batch loading from HDFS, S3, GCS, ADLS.
2) Ultra-Low Latency Queries— Apache Pinot is architected to deliver P99 query latencies in the millisecond range, even at high throughput.
3) High Query Throughput — Apache Pinot is designed to handle very high query concurrency, often at tens or hundreds of thousands of queries per second (QPS) on adequately sized clusters.
4) Columnar Storage — Apache Pinot efficiently stores data column-wise with various compression options.
5) Indexing Options — Apache Pinot supports Sorted, Inverted, Range, Text (Lucene), JSON, Geospatial, Star-Tree (a pre-aggregated cube-like index), and Bloom filter indexes.
6) SQL Interface — Apache Pinot provides a SQL interface (via Apache Calcite) for querying.
7) Multi-Stage Query Engine (v2) — Apache Pinot’s “v2” engine breaks queries into stages, lets it push down filters, reuse expressions across stages, and run joins and window functions at low cost.
8) Cluster Management via Helix/ZK — Apache Pinot uses Apache Helix with ZooKeeper to track segment assignments, replicas, and node health, and to handle rebalancing and failover.
9) High Availability — Apache Pinot replicates data segments across servers. Brokers reroute queries on node failure to keep analytics online without gaps.
10) Pluggable Connectors — Apache Pinot provides lots of Out‑of‑the‑box connectors like Kafka, Pulsar, Kinesis, HDFS, S3, ADLS, and GCS and more!
…and so much more!
Pros and Cons of Apache Pinot:
Apache Pinot Pros:
- Apache Pinot excels at delivering single-to-double-digit millisecond query responses
- Apache Pinot handles massive query concurrency (thousands to 100k+ QPS).
- Apache Pinot supports near‑real‑time ingestion of both stream and batch data sources
- Apache Pinot efficiently handles real-time updates/inserts based on primary keys, unlike many other OLAP systems.
- Apache Pinot offers flexible indexing types (star‑tree, Bloom filter, inverted, geospatial, JSON, range, text, timestamp)
- Apache Pinot integrates seamlessly with Apache Kafka, Kinesis, Pulsar and popular batch frameworks (Spark, Hadoop, Flink)
- Apache Pinot lets you run SQL‑style queries out of the box
- Apache Pinot is fully battle-tested in demanding production environments.
Apache Pinot Cons:
- Apache Pinot lacks built‑in workload isolation or query priority lanes
- SQL support via Calcite is good but may not cover the full ANSI SQL standard
- Apache Pinot setup and cluster management can get complex, especially at scale
- Apache Pinot does not support point updates or deletes on ingested data
- Requires careful configuration of tables, schemas, and particularly the selection and tuning of appropriate indexes per column to achieve optimal performance
- Apache Pinot community and ecosystem remain smaller than some rivals like Apache Druid.
- Apache Pinot offers limited built‑in monitoring and management console in OSS
ClickHouse vs Pinot: What's Best for You?
Apache Pinot is another very direct ClickHouse alternative focused squarely on real-time, low-latency analytics. How do they compare?
Alright, let's break down the ClickHouse vs Pinot comparison.
ClickHouse vs Pinot—Architecture & Complexity
🔮 | Apache Pinot | ClickHouse |
---|---|---|
Core Architecture | Multi-component: Specialized roles (Controller, Broker, Server - RT/Offline/Hybrid). Relies heavily on external ZooKeeper/Helix. | Simpler Core (Relatively): Server process handles multiple roles. Distributed via clustering. Relies on internal Keeper/ZooKeeper for replication. |
Node Roles | Explicit separation of metadata (Controller), query routing (Broker), data serving/ingestion (Server types). | Nodes typically act as shards/replicas, handling queries, ingestion, and storage. Distributed engine handles routing. |
Data Organization | Tables -> Time/Partition-based immutable Segments (columnar). Explicit Realtime/Offline table types. | Tables -> Mutable Data Parts (columnar, sorted by primary key), managed/merged by MergeTree engines. |
Metadata Mgmt | Centralized coordination via Controller/Helix backed by ZooKeeper. | Distributed state management via ClickHouse Keeper (or ZooKeeper) primarily for replication and DDL queue. |
Storage Reliance | Servers cache/serve segments locally. Deep Storage (S3, HDFS) often used for offline segment persistence and backup. | Servers store parts locally (OSS default). Deep Storage (Object Storage) integration is optional via specific engines or Cloud offering. |
Complexity | High: Multiple stateful components + mandatory external ZooKeeper dependency. Requires expertise to operate reliably. | Moderate-to-High (Distributed): Simpler core concepts but distributed setup, tuning (sharding, replication) still requires expertise. |
Indexing | Pluggable & Diverse: User configures indexes per column (Inverted, Range, Text, JSON, Geo, Star-Tree, Bloom). Key for performance. | Simpler & Sort-based: Sparse Primary Key Index (sorting) is fundamental. Optional secondary Data Skipping indexes. Less configuration variety. |
Pinot's architecture provides functional separation and relies on robust external coordination (Helix/ZK) but is inherently complex to manage. ClickHouse offers a simpler core architecture with fewer external dependencies but requires careful configuration for distributed deployments.Pinot's performance hinges heavily on choosing the right pluggable indexes; ClickHouse relies more on its core engine, sorting, and simpler skipping indexes.
ClickHouse vs Pinot—Data Ingestion Capabilities
🔮 | Apache Pinot | ClickHouse |
---|---|---|
Real-time Ingestion | Excellent: Optimized low-level consumers (Kafka, etc.) for very low latency (seconds) visibility. High throughput. Designed for real-time streams. | Near Real-time: Kafka Engine uses Consumer Groups (micro-batching), resulting in efficient ingestion but slightly higher latency (seconds to low minutes). |
Batch Ingestion | Supported via Offline Servers loading pre-built segments from Deep Storage. Can also use Minion tasks. | Primary Strength: Exceptionally fast and efficient parallel batch ingestion (INSERT INTO ... SELECT ... ) from numerous sources/formats. |
Ingestion Latency | Very Low: Design goal is sub-second data visibility for streams. | Low (Batch) / Moderate (Streaming): Batch data available after commit. Streaming visibility depends on micro-batch interval and part merging. |
Upserts/Updates | Strong Native Support: Realtime tables support efficient upserts based on a primary key. Crucial for tracking latest entity state. | Limited/Inefficient: No native primary key upserts. Requires background ALTER TABLE... mutations or specialized engines (e.g: ReplacingMergeTree), less efficient. |
Exactly-Once Semantics | Strong Support: Provides exactly-once guarantees for stream ingestion, particularly with Kafka integration. | Possible: Achievable but often requires more complex application-side logic or specific configurations (e.g: managing Kafka offsets explicitly). |
Pinot is purpose-built for the lowest possible latency stream ingestion and uniquely offers efficient real-time upserts.ClickHouse excels at massive batch ingestion throughput and provides solid near-real-time streaming capabilities.
ClickHouse vs Pinot—Scalability & Operations
🔮 | Apache Pinot | ClickHouse |
---|---|---|
Horizontal Scaling | Yes: Designed for horizontal scaling. Brokers and Servers (Realtime/Offline) can be scaled independently based on load. | Yes: Designed for horizontal scaling via adding nodes (shards/replicas) for distributed analytical processing. |
Ingestion Scaling | Highly Scalable (Streaming): Realtime server consumption can scale independently of source partitions (using low-level Kafka consumers). | Scalable (Batch): Scales batch ingestion horizontally very well. Scalable (Streaming): Kafka Engine scaling typically tied to the number of source Kafka partitions. |
Data Rebalancing | Generally Easier: Segment-based architecture + Helix orchestration often simplifies moving data/rebalancing load when scaling servers compared to ClickHouse parts. | More Manual (OSS): Adding/removing nodes in a sharded cluster often requires manual data rebalancing procedures, which can be complex and time-consuming. |
Replication | Handled via segment assignment and replication strategies managed by Helix/Controller. Coordination can be simpler conceptually. | Handled internally by ReplicatedMergeTree engines using ClickHouse Keeper (or ZooKeeper) for consensus and log replication. Robust but configuration requires care. |
Multi-tenancy | Built-in Support: Offers tenant tagging for isolating resources (e.g: assigning specific tenants to specific Brokers/Servers). | Less Built-in: Typically achieved via configuration (separate databases, users with quotas, potentially separate clusters) rather than native tagging. |
Elasticity | Adding/removing stateless Brokers is easy. Adding/removing Servers is facilitated by Helix rebalancing segments automatically. | Adding/removing nodes can be operationally more complex (OSS), especially needing data movement for sharded tables. Managed Cloud services greatly improve elasticity. |
Pinot often provides greater operational ease for scaling (especially stream ingestion) and data rebalancing due to its segment management via Helix. ClickHouse offers high scalability but typically requires more manual operational effort for cluster topology changes when self-hosted. Pinot has more explicit multi-tenancy features.
ClickHouse vs Pinot—Performance & Querying
🔮 | Apache Pinot | ClickHouse |
---|---|---|
Query Language | SQL (via Apache Calcite, good coverage but some limitations) or native PQL (Pinot Query Language). | ClickHouse SQL (Extended standard SQL dialect, very powerful analytical functions). |
Query Latency | Ultra-Low (Targeted): Hyper-optimized for P99 millisecond latency on queries hitting indexes well. Design goal for user-facing apps. | Very Low (Broad): Extremely fast across a wide range of OLAP queries, including heavy scans/aggregations. May have slightly higher latency on simple indexed lookups than optimized Pinot. |
Indexing | Pluggable & Diverse: Sorted, Inverted, Range, Text, JSON, Geospatial, Star-Tree (pre-aggregation), Bloom filter. Requires careful configuration per column based on query patterns. | Simpler & Sort-Focused: Sparse Primary Key (sorting) is key. Secondary Data Skipping Indexes (minmax, set, bloom, etc.). Less index variety, simpler configuration. |
Query Execution | Broker routes to Servers -> Servers execute using columnar format + selected pluggable indexes. Multi-stage engine (v2) evolving for complex queries. | Vectorized Engine: Processes data in batches using CPU SIMD instructions. Parallel execution across cores/nodes. Efficient for scans and aggregations. |
Joins | Limited: Historically focused on lookups. Multi-stage engine (v2) adds support for some broadcast and collocated hash joins, but still evolving and often less performant/flexible than CH. | More Robust: Supports standard SQL JOINs (INNER, LEFT, RIGHT, FULL, CROSS). Performance depends on join type/size/distribution, but significantly more capable than Pinot. |
Updates/Deletes | Real-time Upserts: Strong support via primary keys on Realtime tables. No easy way to mutate historical (Offline) segment data. | Inefficient Mutations: No native upserts. ALTER TABLE... UPDATE/DELETE runs as heavy background operations. Best for append-only or infrequent batch corrections. |
Pinot is laser-focused on achieving the lowest possible latency for specific, index-optimized query patterns common in user-facing applications, leveraging its diverse indexing. ClickHouse provides extreme performance across a broader spectrum of analytical queries, relies more on its vectorized engine and data sorting, and offers significantly better JOIN support.
When Would You Choose Pinot vs ClickHouse?
Choose Apache Pinot if:
- Your absolute critical requirement is P99 query latency in the millisecond range for high-throughput, user-facing analytical applications.
- You need low-latency real-time ingestion from streams (Kafka, etc.) with data queryable almost instantly.
- Efficient real-time upserts based on a primary key are essential for your use case (e.g: tracking the latest user status).
- Your specific query patterns can be heavily accelerated by Pinot's specialized pluggable indexes (especially Star-Tree, inverted, range indexes) and you can invest in configuring them correctly.
- You need highly scalable stream ingestion or benefit from built-in multi-tenancy resource tagging.
- You have the operational resources and expertise to manage its complex multi-component architecture and external ZooKeeper dependency.
Choose ClickHouse if:
- Your primary need is maximum raw performance across a broad range of complex OLAP queries, including large scans, heavy aggregations, and more sophisticated SQL JOINs.
- High-throughput batch data ingestion is a major part of your workload, or near-real-time ingestion via micro-batching (Kafka engine) is sufficient.
- You value a relatively simpler core architecture and deployment model with fewer mandatory external dependencies.
- Your queries benefit significantly from data sorting (via the primary key index) and efficient vectorized execution for scans.
- You prefer a more standard, extended SQL dialect with robust support for various JOIN types and complex/nested data structures (Array, Map, JSON).
- Cost-performance (performance per resource unit) and high data compression are key considerations.
TL;DR: Apache Pinot is a specialized engine targeting ultra-low latency for high-concurrency user-facing analytics, excelling with real-time streams and upserts but requiring significant operational investment and careful index tuning. ClickHouse offers broader high-performance OLAP capabilities, superior batch ingestion, more powerful SQL/JOINs, and a potentially simpler operational model, making it a versatile choice for diverse analytical tasks.
Continue reading...
⏱️ ClickHouse Alternatives: Category 4—ClickHouse vs Specialized Time-Series Databases
ClickHouse is fast and uses a columnar design, which is what makes it great. This category, though, is all about databases that are tailor-made for time-series data, meaning they can handle storing, processing, and querying it efficiently. TimescaleDB is a strong example of this type of database, and it's often compared to ClickHouse.
ClickHouse Alternative 9—TimescaleDB
TimescaleDB is a popular open source database for time-series data. It builds on PostgreSQL, inheriting its reliability, mature ecosystem, and standard SQL compliance, then adds specialized optimizations. These optimizations are key to handling large amounts of time-series data efficiently. With TimescaleDB, you get the familiarity and flexibility of a relational database, along with the performance and scalability needed for time-series analysis. This makes it a compelling choice for people already using or comfortable with PostgreSQL.
TimescaleDB in 100 Seconds - ClickHouse Alternatives - ClickHouse Competitors
TimescaleDB Architecture
TimescaleDB doesn't replace PostgreSQL; it integrates deeply within it, modifying and extending core functionalities via PostgreSQL's powerful extension API.
TimescaleDB operates as an extension within PostgreSQL, meaning it modifies and adds capabilities to a standard PostgreSQL instance rather than replacing it. The TimescaleDB architecture is specifically designed for time-series data, using hypertables as its core concept to efficiently manage large datasets over time.
➥ Hypertables and Chunking
TimescaleDB introduces hypertables. These look and act like standard PostgreSQL tables to the user but automatically partition data underneath. Data is divided into smaller, manageable tables called chunks, primarily based on time intervals. You can add secondary partitioning dimensions based on keys like IDs or location. This chunking improves insert and query performance by allowing operations to focus on smaller data sets, often residing in memory for recent data.
➥ Compression and Retention
TimescaleDB provides built-in compression capabilities. You can configure policies to automatically convert older data chunks from a standard row format to a compressed columnar format. This reduces storage footprint. Queries accessing compressed data decompress it automatically as needed. You can also set data retention policies to automatically delete chunks older than a specified age, helping manage storage use.
➥ Query Optimization
TimescaleDB uses PostgreSQL's query planner but adds time-series specific optimizations. A key technique is constraint exclusion, where the planner uses query conditions (like time ranges) to avoid scanning chunks that cannot contain relevant data. TimescaleDB also offers continuous aggregates. These automatically maintain materialized views containing pre-aggregated summary data, speeding up common aggregation queries by avoiding repeated computations over raw data.
➥ Deployment Options
You can deploy TimescaleDB within a standard PostgreSQL installation. Common deployment environments include local machines (using Docker or direct installs on Linux, macOS, Windows), cloud virtual machines (like AWS EC2), or managed Kubernetes platforms using PostgreSQL operators. Cloud providers also offer managed TimescaleDB services.
TimescaleDB Key Features
TimescaleDB, which is built on PostgreSQL, has particular time-series capabilities:
1) Hypertables — TimescaleDB shards time-series data into manageable chunks for efficient ingestion and querying.
2) Continuous aggregates — TimescaleDB refreshes materialized views automatically to speed up time-based analytics.
3) Native compression — TimescaleDB compresses historical data with columnar storage to cut disk usage by up to ~90 percent.
4) Time-series hyperfunctions — TimescaleDB provides SQL functions like time_bucket for flexible time-based grouping and analysis.
5) Data retention policies — TimescaleDB automates dropping or moving old data based on user-defined retention rules.
6) Bottomless storage — TimescaleDB offloads warm data to external storage like Amazon S3 for near-unlimited retention.
7) PostgreSQL compatibility — TimescaleDB keeps full SQL support and works with existing Postgres tools without extra layers.
8) High Ingest Performance — TimescaleDB is optimized for handling high rates of time-series writes, often outperforming vanilla PostgreSQL for these workloads.
9) Distributed Hypertables — TimescaleDB enables horizontal scaling across multiple PostgreSQL nodes for larger datasets and higher query throughput.
10) Automated job scheduler — TimescaleDB runs background jobs to manage tasks like retention, compression, and continuous aggregate refresh.
Pros and Cons of TimescaleDB:
TimescaleDB Pros:
- TimescaleDB delivers much higher and stable ingest rates for time‑series workloads compared to vanilla PostgreSQL.
- TimescaleDB enables up to ~1000× faster time‑series queries and 90 % data compression versus PostgreSQL.
- TimescaleDB automates data partitioning and indexing through hypertables.
- TimescaleDB offers continuous aggregates for pre‑computed query results, speeding up analytics.
- TimescaleDB includes SQL functions and table structures specialized for time‑series analysis, such as time_bucket.
- TimescaleDB is open source under the Apache 2.0 license, with community and enterprise editions.
- TimescaleDB extends PostgreSQL, retaining full SQL compatibility and ecosystem support.
- TimescaleDB handles high‑scale data ingestion and complex queries with consistent performance.
- TimescaleDB supports blob data via PostgreSQL, enabling storage of unstructured time‑series blobs.
- TimescaleDB supports distributed hypertables across nodes for horizontal scaling (enterprise feature).
TimescaleDB Cons:
- TimescaleDB doesn’t support distributed scheduling of background jobs; jobs run only on the access node.
- TimescaleDB doesn’t support reordering chunks, which limits some maintenance workflows.
- TimescaleDB doesn’t support parallel‑aware scans and appends on distributed hypertables.
- TimescaleDB doesn’t enforce role and permission consistency across nodes in a cluster.
- TimescaleDB’s ingestion rate suffers with high‑cardinality datasets due to its row‑based design.
- TimescaleDB inserts data slower than vanilla PostgreSQL and uses more memory for chunk management.
- TimescaleDB performs poorly on tables with frequent updates, as it optimizes for append‑only workloads.
- TimescaleDB sorts by time slower than standard PostgreSQL in large tables.
- TimescaleDB requires non‑null partitioning columns; time columns used for partitioning can’t be NULL.
- TimescaleDB’s continuous aggregates live only on the access node, which can limit distributed scalability.
ClickHouse vs TimescaleDB: What's Best for You?
Now let's see how ClickHouse and TimescaleDB differ from each other:
ClickHouse vs TimescaleDB—Architecture & Design Philosophy
🔮 | TimescaleDB | ClickHouse |
---|---|---|
Core Design | PostgreSQL Extension: Enhances a mature Object-Relational DBMS (ORDBMS) specifically for time-series workloads. | Standalone Columnar OLAP DBMS: Built from the ground up for maximum analytical query speed and efficiency. |
Primary Model | Time-Series Database integrated with Relational. Strong support for both time-series and standard relational data/queries. | Columnar / Relational DBMS optimized for OLAP. Handles time-series well due to speed but not its sole focus. |
Storage Format | Hybrid: Row-oriented (recent/uncompressed chunks) + Columnar (older/compressed chunks, policy-driven). | Primarily Columnar: Data stored column-wise inherently. High compression applied consistently. |
Data Organization | Hypertables automatically partitioned into Chunks based on time (mandatory) and optional space dimensions. | Tables with data stored in Parts, sorted by a Primary Key (often including time), managed by MergeTree engines. |
Execution Engine | PostgreSQL's row-based executor with TimescaleDB optimizations (chunk pruning, specialized scans on compressed data). | Vectorized execution engine (processes data in batches using SIMD instructions). Optimized for columnar scans and aggregations. |
Concurrency | Inherits PostgreSQL MVCC for handling concurrent reads and writes according to ACID principles. | Lock-free reads; optimistic concurrency control. Designed for high analytical query concurrency. |
Transactions | Full ACID compliance inherited from PostgreSQL. | Limited: Atomic single-statement operations. No multi-statement ACID guarantees. Eventual consistency for replication. |
TimescaleDB builds upon the versatile and reliable PostgreSQL foundation, offering a hybrid storage model and strong relational capabilities alongside time-series features. ClickHouse uses a specialized columnar/vectorized architecture focused purely on maximizing analytical performance.
ClickHouse vs TimescaleDB—Performance Profile
🔮 | TimescaleDB | ClickHouse |
---|---|---|
Analytical Query Speed | Good to Fast: Especially strong with time filtering (chunk pruning), continuous aggregates, and queries on compressed data. Can outperform ClickHouse on selective queries or JOINs with relational tables. | Extremely Fast: Generally faster for broad OLAP queries involving large scans across many rows, high-cardinality aggregations, or complex calculations due to vectorized engine. |
Ingestion Rate | High & Stable: Optimized INSERT performance for time-series patterns, handles concurrent small writes well. Often considered very reliable ingest. |
Very High (Batch): Exceptionally high throughput for large batch inserts. Kafka engine provides efficient near-real-time micro-batching. Less ideal for frequent tiny inserts. |
Query Types Favored | Time-series specific analysis (time_bucket ), queries needing JOINs with relational metadata, queries requiring updates/deletes. Queries benefiting from Continuous Aggregates. |
Wide aggregations (GROUP BY on high cardinality), large range scans, queries touching few columns over many rows, approximate queries. Queries on raw event data. |
Updates/Deletes | Efficient: Supports standard SQL UPDATE /DELETE effectively, especially on uncompressed (recent) data chunks. Leverages PostgreSQL mechanisms. |
Inefficient: No native upserts. ALTER TABLE... mutations are heavy background operations. Best suited for append-only or immutable datasets. |
Real-time Analytics | Good: Continuous Aggregates provide fast pre-computed results with real-time merging. Query performance on recent (uncompressed) data is good. | Excellent: Extreme speed allows fast aggregations directly on raw, recent data. Materialized Views also enable pre-computation. |
Disk Usage/Compression | Excellent (Compressed): Native columnar compression on older chunks achieves high ratios. Overall usage depends on mix of compressed/uncompressed data. | Excellent (Inherent): Native columnar format provides high compression ratios across all data consistently. Configurable per column. |
ClickHouse generally offers superior raw speed for large-scale OLAP scans and aggregations. TimescaleDB provides strong time-series query performance, excels where relational capabilities (JOINs, Updates, ACID) are needed alongside time-series data, and simplifies common aggregations via Continuous Aggregates.
ClickHouse vs TimescaleDB—Scalability & Operations
🔮 | TimescaleDB | ClickHouse |
---|---|---|
Horizontal Scaling | Yes (Multi-Node): Distributed hypertables allow sharding across multiple PostgreSQL instances. Architecture relies on Postgres streaming replication between nodes. | Natively Designed for Analytics: Built for horizontal scaling via sharding data and distributing query processing across nodes. |
Scaling Model | Supports both Vertical Scaling (inherent in Postgres) and Horizontal Scaling (via multi-node features). | Primarily designed for Horizontal Scaling for analytical workloads, though vertical scaling is also possible. |
Cluster Management | Leverages standard PostgreSQL tools and practices for HA (e.g: Patroni, pg_auto_failover), backup (pg_dump, pg_basebackup), monitoring. Multi-node adds coordination needs. | Requires managing ClickHouse Keeper (or ZooKeeper) for replication and distributed DDL. Configuration often via XML/YAML files. Operational tooling ecosystem is maturing. |
Operational Ease | Higher (for Postgres users): Leverages existing Postgres knowledge, tools, and operational practices. | Steeper Learning Curve: Requires learning ClickHouse-specific operations, configuration (XML), and cluster management (Keeper, sharding/replication setup). |
ClickHouse is designed ground-up for distributed analytics. TimescaleDB adds distributed capabilities to PostgreSQL, leveraging its existing operational ecosystem, which can be an advantage for existing Postgres teams but may have different scaling characteristics than purpose-built distributed systems.
ClickHouse vs TimescaleDB—Data Model, Querying, & Ecosystem
🔮 | TimescaleDB | ClickHouse |
---|---|---|
Data Model | Relational + Time-Series: Inherits PostgreSQL's rich relational model (constraints, foreign keys) combined with hypertables optimized for time. Strict schema enforcement. | Primarily Relational/Columnar for OLAP: Focus on simple, often denormalized schemas for analytical speed. Less emphasis on strict relational integrity enforcement. |
Storage Engine | Uses PostgreSQL's storage (heap tables, indexes) plus TimescaleDB's chunk management, metadata, and columnar compression layer for older chunks. | Primarily uses specialized MergeTree family engines (MergeTree, ReplacingMergeTree, SummingMergeTree, etc.) optimized solely for columnar storage, merging, and querying. |
Indexing | Leverages all PostgreSQL index types (B-tree, BRIN, GIN, GiST) on uncompressed chunks. Automatically creates time index. Optimizations for compressed chunks. | Specialized OLAP Indexing: Sparse Primary Index (sorting/range scans). Limited secondary Data Skipping indexes. Not designed for efficient point lookups. |
Query Language | Standard PostgreSQL SQL + specialized time-series Hyperfunctions (time_bucket , first , last , approx_percentile , etc.). High compatibility. |
Extended SQL Dialect: Optimized for analytics. Powerful functions for arrays, maps, strings, approximate calculations. Some non-standard syntax/behavior. |
SQL Support/ACID | Full standard SQL support. Full ACID compliance. Handles complex JOINs, CTEs, window functions robustly. | Analytical SQL Focus: Excellent for aggregations/scans. Good JOIN support (improving). No multi-statement ACID transactions. |
Ecosystem | Benefits from vast PostgreSQL ecosystem: Tools (psql, pgAdmin), connectors (JDBC, ODBC, Python, etc.), libraries, community support, and extensions (PostGIS, etc.). | Growing Ecosystem: Strong integrations in observability (Grafana, Prometheus). Standard connectors (JDBC/ODBC, Python). Large open source community. |
TimescaleDB shines with its standard SQL compliance, full ACID support, rich PostgreSQL indexing, and seamless integration with the vast Postgres ecosystem. ClickHouse offers a powerful, analytics-focused SQL dialect and specialized MergeTree engines but deviates more from SQL standards and lacks full ACID properties.
When Would You Choose TimescaleDB vs ClickHouse?
Choose TimescaleDB if:
- You are already using PostgreSQL and want to add high-performance time-series capabilities without migrating to a completely different system.
- You need to store and query time-series data alongside traditional relational data and require efficient SQL JOINs between them.
- Full standard SQL compliance and ACID transactional guarantees are mandatory for your application logic or data integrity requirements.
- Your application requires frequent updates or deletes to recent or historical time-series records.
- You want to leverage the mature and extensive PostgreSQL ecosystem, including tools, connectors, and other extensions like PostGIS for geospatial analysis.
- Built-in, specialized time-series functions (hyperfunctions) and automated continuous aggregates significantly simplify your analytical workload.
- Your team has strong PostgreSQL operational expertise.
Choose ClickHouse if:
- Your primary objective is maximum raw query speed and throughput for broad analytical (OLAP) queries over massive datasets (time-series or otherwise).
- Data is largely immutable (append-only focus), and updates/deletes are rare or handled via batch processes.
- Workloads involve complex, high-cardinality aggregations, large-scale scans, or analysis directly on raw event data.
- Extremely high batch ingestion throughput is a critical requirement.
- Storage efficiency through consistently high columnar compression across all data is are the highest priorities.
- Native horizontal scalability designed specifically for distributing analytical query load is the primary scaling strategy.
- You need ClickHouse's specialized analytical functions or data types (e.g: for arrays, maps, approximate calculations).
In short: TimescaleDB offers a powerful and convenient way to manage time-series data within the robust, familiar, and ACID-compliant PostgreSQL environment, excelling where relational capabilities need to coexist with time-series analysis. ClickHouse remains the specialized champion for extreme OLAP performance, particularly on large-scale, append-heavy analytical workloads where raw speed, compression, and native distributed query processing are the top priorities.
Both TimescaleDB and ClickHouse address the time-series challenge from different architectural starting points.
Continue reading...
🦆 ClickHouse Alternatives: Category 5—ClickHouse vs In-Process / Embedded Database
Sometimes, the requirement isn't for a large-scale, distributed analytical database server cluster. Instead, you might need light weight and fast analytical query capabilities directly within an application, for local data processing, or for interactive exploration without the overhead of configuring and managing a separate server. This is where in-process analytical databases comes in. DuckDB is the leading example in this space and represents a unique type of ClickHouse alternative, often serving different but sometimes overlapping needs.
ClickHouse Alternative 10—DuckDB
DuckDB is an open source, high-performance in-process analytical data management system. Often termed "the SQLite for analytics", its core distinction is that it runs as a library linked directly into a host application process. (e.g: Python, R, Java, C++, Node.js, Rust, Wasm) rather than as a standalone server. This eliminates network overhead and simplifies deployment for local or embedded analytical tasks.
DuckDB Tutorial For Beginners - ClickHouse Alternatives - ClickHouse Competitors
DuckDB Architecture
DuckDB's architecture is designed for high analytical performance within the constraints and benefits of an embedded, single-node environment.
➥ Storage Layer
DuckDB Architecture stores data in a single file using row groups, which group rows into horizontal partitions similar to Parquet. Each row group holds a fixed number of tuples, which lets the engine parallelize scans and compression across threads.
➥ Query Processing Pipeline
The engine parses SQL into a parse tree of SQLStatement and QueryNode, using a parser derived from PostgreSQL. The Binder resolves table names, column types, and functions, turning parsed nodes into typed expressions. DuckDB applies both rule‑based and cost‑based rewrites to the logical plan using simple heuristics. It pushes down filters to scans and picks join orders with basic cost estimates.
➥ Execution Engine
DuckDB processes data in DataChunks, each holding Vectors of a fixed size (2048 tuples by default), and pushes these chunks through operators. It loops over each vector to work on batches of values, which lowers per‑tuple overhead. The engine parallelizes work at the row‑group level, assigning DataChunks to worker threads for scans and joins. It spills intermediate results to disk when memory runs low, letting it handle data sets larger than RAM.
➥ Concurrency and Transactions
DuckDB uses multi‑version concurrency control to handle concurrent operations from multiple threads within the same process. Provides snapshot isolation and ACID compliance for transactions, using a Write-Ahead Log (WAL) for durability when persisting to its single-file storage format.
DuckDB Key Features
DuckDB packs a remarkable set of analytical features:
1) In‑process analytics engine — DuckDB runs entirely inside the host application process; no server setup or network latency.
2) Columnar storage with vectorized execution — DuckDB stores data column‑wise and processes it in vector batches, enabling parallel, CPU‑efficient scans over large datasets.
3) Zero‑config single‑file storage — DuckDB stores entire database in a single, portable file. Also supports pure in-memory operation.
4) Rich file format support — DuckDB can read and write CSV, Parquet, JSON and query remote files over HTTP(S) or S3, without external ETL steps.
5) Rich SQL dialect — DuckDB supports a large subset of SQL, including window functions, CTEs, complex data types (STRUCT, MAP, LIST), correlated subqueries, UPSERT syntax, full-text search extensions, and more.
7) ACID‑compliant transactions — DuckDB provides full ACID compliance with snapshot isolation via MVCC for operations within a single process.
8) Multi‑language client APIs — DuckDB seamlessly integrates with Python (Pandas, Polars, Arrow), R, Java, C/C++, Node.js, Rust, Go, etc.
9) Ultra‑portable — With zero dependencies, DuckDB compiles to Linux, macOS, Windows, ARM and even runs in web browsers.
10) Lightweight deployment — Delivered as a small header and implementation file, DuckDB has no external dependencies, letting you drop it into any application easily.
… and so much more!
Pros and Cons of DuckDB:
DuckDB Pros:
- DuckDB is an embedded analytical database with no external dependencies.
- DuckDB uses columnar storage and vectorized execution, delivering high performance for analytical queries.
- DuckDB processes large datasets in Parquet and CSV formats directly, avoiding lengthy data loading steps.
- DuckDB integrates with multiple languages, letting users query data frames via SQL without moving data between tools.
- DuckDB offers full ACID compliance through its MVCC engine.
- DuckDB is open source.
- DuckDB supports advanced SQL features like window functions and complex joins for flexible analytics.
- DuckDB runs in-process and eliminates network overhead, enabling fast local query performance.
- DuckDB runs virtually anywhere.
DuckDB Cons:
- DuckDB does not support distributed query execution and cannot scale across multiple nodes.
- DuckDB’s write and update operations are slower compared to its optimized read performance.
- DuckDB’s performance may degrade when dataset sizes exceed available memory, as it is not purely in-memory.
- DuckDB has limited concurrency: only one writer can access the database at a time, requiring exclusive locks.
- DuckDB lacks built-in role-based access controls and comprehensive user management features.
- DuckDB provides no native client‑server architecture, limiting remote and multi‑user deployments.
- DuckDB is relatively new, so its ecosystem of third-party tools and integrations is smaller than more mature systems.
- DuckDB doesn’t optimize for transaction-heavy workloads, making it less suitable for OLTP use cases.
- DuckDB connector can only access a single database instance, complicating multi‑database scenarios.
- DuckDB focuses on OLAP (Online Analytical Processing) workloads and doesn’t suit transaction-heavy applications.
ClickHouse vs DuckDB: What's Best for You?
Finally, we have reached the last ClickHouse alternative. Let's dig into the ClickHouse vs DuckDB comparison and see which one is better suited for your needs.
ClickHouse vs DuckDB—Architecture & Deployment Model
🔮 | DuckDB | ClickHouse |
---|---|---|
Core Model | In-Process OLAP Library: Runs inside the host application process. No separate server. | Client-Server OLAP DBMS: Runs as a standalone server process, potentially forming a distributed cluster. Clients connect over network/IPC. |
Deployment | Embedded: Linked as a library. Data stored in single file or :memory: . NO server management. |
Server-Based: Requires installation, configuration, management (OSS) or using a Managed Cloud service. |
Storage | Columnar; Single-file persistence (portable) or in-memory. Optimized for local access. | Columnar (MergeTree engines); Disk-based (local FS or object storage). Designed for persistent, large-scale server storage. |
Processing | Single-Node Vectorized Execution: Parallelism across CPU cores within the host process. | Distributed Vectorized Execution: Designed for parallel processing across multiple nodes in a cluster. |
Concurrency | Handles multi-threading within a single process (MVCC). Limited inter-process write concurrency (typically 1 writer). | Designed for high inter-process/network concurrency (many clients connecting to the server/cluster). |
Distribution | Single-Node: Core engine is not distributed. (Related projects like MotherDuck offer serverless services based on DuckDB). | Natively Distributed: Built for sharding, replication, and distributed query execution across a cluster managed via Keeper/ZooKeeper. |
DuckDB provides analytical power within an application for local tasks, prioritizing ease of deployment and low-latency local access. ClickHouse provides a dedicated, persistent, potentially distributed analytical service accessible by multiple clients/applications over a network.
ClickHouse vs DuckDB—Performance & Scalability
🔮 | DuckDB | ClickHouse |
---|---|---|
Analytical Performance | Excellent (Single-Node): Highly optimized vectorized engine delivers exceptional speed for complex SQL analytics within the limits of a single machine's resources. | Excellent (Distributed): Vectorized engine combined with distributed architecture allows scaling performance to handle massive datasets (PBs) across a cluster. |
Large Datasets | Performance degrades if working set significantly exceeds available RAM (though can process via spilling). Practically limited by single-node resources. | Excels: Distributed architecture specifically designed to handle datasets far exceeding single-node memory/disk capacity by parallelizing work across the cluster. |
Querying Files | Outstanding: Optimized C++ readers directly query Parquet, CSV, JSON, Arrow files (local, HTTP, S3) very efficiently, often without explicit loading. Can query file collections. | Good: Can query external sources via table engines (e.g: S3 , URL , File ) or table functions, but often less seamless or performant than DuckDB for direct file interaction. |
Horizontal Scaling | No: Not designed for distributed query execution across multiple nodes. | Yes: Natively supports sharding and replication for horizontal scale-out. |
Vertical Scaling | Primary scaling method: Performance directly benefits from more CPU cores, RAM, faster disk on the host machine. | Supports vertical scaling, but horizontal scaling is the key strategy for massive datasets. |
Resource Usage | Lightweight: Designed for efficiency within a single process. Low startup overhead. | Can be Resource-Intensive: Server processes and distributed clusters require significant CPU, RAM, network bandwidth, and disk resources. |
DuckDB offers state-of-the-art single-node analytical performance, especially for querying files directly. ClickHouse provides scalable performance for datasets far exceeding single-node capabilities through its distributed architecture.
ClickHouse vs DuckDB—Data Handling, Features, & Ecosystem
🔮 | DuckDB | ClickHouse |
---|---|---|
Data Formats Access | Excellent Direct Read: Optimized readers for Parquet, CSV, JSON, Arrow, etc., are a core strength. | Broad Ingest/Export Support: Supports many formats for loading/unloading data. Optimized for its native MergeTree format. External access via engines/functions. |
SQL Dialect | Rich, Postgres-compatible SQL dialect. Supports window functions, complex types (STRUCT, MAP, LIST), CTEs, UPSERT. | Extended Analytical SQL: Powerful functions for aggregations, arrays, maps, strings, approximate calculations. Some non-standard syntax. |
Updates/Deletes/ACID | ACID compliant within its single-process scope. Supports standard SQL DML (UPDATE , DELETE ), though not performance-optimized for high volume. |
Limited Mutations: No full ACID transactions. ALTER TABLE... UPDATE/DELETE are heavy background operations. Optimized for append-only. |
Compression | Good standard columnar compression techniques (dictionary, RLE, FOR) + integrates external codecs (ZSTD, Snappy). | Excellent & Tunable: Native columnar compression with advanced codecs (LZ4, ZSTD, Delta, Gorilla, etc.), configurable per column. Often achieves higher ratios. |
Extensibility | Growing ecosystem of extensions (Spatial, FTS, connectivity). Simple loading mechanism. | Integrations via interfaces (HTTP, TCP, JDBC/ODBC), UDFs (multiple languages via executable), external dictionaries/models. |
JSON Handling | Good support for querying JSON type. |
Highly optimized native JSON object type and extensive dedicated functions. |
Ecosystem Focus | Strong in Data Science (Python/R notebooks, Pandas/Polars/Arrow interop), embedded applications, local analytics tools, Wasm (in-browser). | Focused on Server-based Analytics: BI tools (Grafana, Tableau, Superset), data pipelines (Kafka, ETL tools), large-scale logging/observability, Cloud offerings. |
DuckDB excels in ease of use, direct file access, data science integration, and providing ACID guarantees within its embedded context. ClickHouse offers server-grade features, best in class compression, specialized analytical types/functions, and is built for large-scale, persistent analytical infrastructure.
ClickHouse vs DuckDB—When Would You Pick One Over the Other?
So, after all that, when should you reach for DuckDB and when for ClickHouse?
Choose DuckDB if:
- You need fast OLAP capabilities embedded directly within an application (Python, R, Java, C++, Node.js, Rust,....).
- You are performing local data analysis, exploration, or prototyping on a single machine.
- Your analytical datasets fit reasonably within the memory and disk resources of a single node (or can tolerate performance impact of disk spilling).
- You need to efficiently query various file formats (Parquet, CSV, JSON, Arrow) directly without setting up a database server or complex ETL pipelines.
- Simplicity of deployment and zero server management are primary requirements.
- You are working heavily within the Python or R data science ecosystems (Jupyter/IPython notebooks, Pandas/Polars/Arrow dataframes).
- You require ACID transactional guarantees for your local analytical data manipulations.
- You want to perform analytics directly in a web browser using WebAssembly.
Choose ClickHouse if:
- You are building a shared, persistent analytical database server or cluster accessed by multiple users, applications, or services over a network.
- You are dealing with very large datasets (hundreds of GBs to Petabytes) that require distributed storage and processing across multiple nodes.
- Horizontal scalability, high availability, and fault tolerance through replication are essential requirements for your analytical infrastructure.
- You need a high-performance backend for real-time analytics dashboards (BI tools), large-scale log/metric analysis, or data warehousing.
- Optimized storage efficiency via industry-leading compression is critical for managing costs at scale.
- You have the operational capacity to manage a server-based deployment (or opt for a managed ClickHouse Cloud service).
- Your application requires a dedicated, standalone analytical database service separate from application processes.
TL;DR: DuckDB is not a direct replacement of ClickHouse but acts as an outstanding ClickHouse alternative for local or embedded analytical tasks. It brings high-performance OLAP capabilities directly into applications and data science workflows with unparalleled ease of use, especially for querying files. ClickHouse remains the choice for building scalable, persistent, server-based analytical database infrastructure. They often complement each other well in a larger data ecosystem.
Continue reading...
Conclusion
And that's a wrap! It's clear that ClickHouse is a seriously powerful contender, especially when you need blazing-fast OLAP query performance, efficient data compression, and the ability to handle real-time data streams. Its columnar architecture, vectorized execution, and clever MergeTree engine make it a beast for specific analytical tasks. But, as we saw, it's not without its limitations—the lack of strong OLTP support, tricky JOINs, and operational overhead for self-hosting mean it isn't always the perfect fit. There's no single "best" database, only the best one for your specific problem.
In this article, we have covered:
- What Is Clickhouse Used For?
- What Is Clickhouse Best For?
- What Are the Drawbacks of Clickhouse?
- 10 True ClickHouse Alternatives: Which One Wins?
- 1) ClickHouse Alternative 1—Snowflake
- 2) ClickHouse Alternative 2—Databricks
- 3) ClickHouse Alternative 3—Google BigQuery
- 4) ClickHouse Alternative 4—Amazon Redshift
- 5) ClickHouse Alternative 5—MySQL
- 6) ClickHouse Alternative 6—PostgreSQL
- 7) ClickHouse Alternative 7—Apache Druid
- 8) ClickHouse Alternative 8—Apache Pinot
… and so much more!
FAQs
What is ClickHouse used for?
Primarily OLAP: real-time dashboards, log/event analysis, time-series analysis, large-scale ad-hoc querying, user behavior tracking. Excels at fast aggregation/filtering on large datasets. If these aren't your primary needs, consider ClickHouse alternatives.
Is ClickHouse an OLAP or OLTP database?
Definitely OLAP. Designed for analytical queries, lacks mechanisms for efficient OLTP.
What is the difference between OLAP and OLTP?
OLTP handles frequent, small operational transactions (inserts, updates, deletes) prioritizing consistency (ACID) and single-record speed (row-stores like MySQL/Postgres). OLAP handles complex analytical queries on historical data prioritizing read speed for scans/aggregations (columnar stores like ClickHouse/Snowflake).
Can ClickHouse replace traditional data warehouses (like Snowflake / Google BigQuery / Amazon Redshift)?
Sometimes. Yes, if raw speed, real-time ingest, and cost-efficiency are essential and its model fits. No, if the ease of managed services, elasticity, broader platform features, or mature complex JOIN handling are more important.
Which ClickHouse alternative is best for real-time analytics?
Besides ClickHouse: Apache Druid & Apache Pinot (ultra-low latency focus), Cloud Platforms like BigQuery/Databricks (strong streaming ingest), TSDBs like InfluxDB/TimescaleDB (for metrics/events). Each ClickHouse alternatives has trade-offs.
Is ClickHouse suitable for transactional workloads?
No. Its mutation mechanism (background ALTER TABLE...) is slow and inefficient for single-row operations, and it lacks strict ACID guarantees needed for OLTP.
Is Clickhouse free? What about its alternatives?
ClickHouse OSS is free (pay infra/ops); ClickHouse Cloud is paid. Many ClickHouse alternatives (Apache Druid, Apache Pinot, ES, InfluxDB, TimescaleDB, DuckDB, MySQL, Postgres) have OSS cores + paid options. Cloud DW ClickHouse alternatives (Snowflake, Google BigQuery, Amazon Redshift) are proprietary managed services. Databricks platform is commercial over OSS components.
How does ClickHouse handle JOINs compared to other systems?
Supports SQL JOINs. Performs well joining large tables to small (dictionary) tables or via GLOBAL JOINs. Can be less performant than MPP DWs for large distributed JOINs (requires data shuffling). Denormalization is often recommended.
What kind of hardware does ClickHouse typically need?
Benefits from multi-core CPUs (with SIMD), sufficient RAM (for query working sets), fast local storage (NVMe SSDs preferred), and good network bandwidth for distributed queries. Efficient but scales with better hardware.
Why are MySQL and PostgreSQL generally poor choices for ClickHouse's main job?
Their core row-oriented storage engines read entire rows even for queries needing few columns, making analytical scans vastly inefficient compared to ClickHouse's columnar reads. They lack optimized columnar compression and vectorized execution for analytics.
How does DuckDB differ fundamentally from ClickHouse Server?
Deployment model: DuckDB is an embedded in-process library; ClickHouse is typically a client-server database. DuckDB is single-node focused; ClickHouse is designed for distributed clusters. DuckDB excels at local/embedded tasks and querying files directly.
What is "vectorized execution" and why does it matter for ClickHouse and others?
Vectorized execution means processing data in batches (vectors) instead of row-by-row. It allows efficient use of CPU caches and SIMD instructions, significantly speeding up analytical computations. Used by ClickHouse and many modern analytical ClickHouse alternatives.
When to choose Apache Druid or Apache Pinot over ClickHouse?
When the absolute top priority is minimum query latency (milliseconds P99) for high-concurrency, user-facing interactive applications/dashboards, and you can manage their higher operational complexity and leverage their specific indexing strategies.
Can I migrate easily between these different analytical databases?
No, migration is usually complex. Challenges include schema redesign, SQL dialect translation, data export/import time/cost, feature differences, and different performance tuning needs. It's typically a major project.
What's the role of ClickHouse Keeper?
ClickHouse Keeper is ClickHouse's built-in Raft-based coordination service (alternative to ZooKeeper). Used by ReplicatedMergeTree tables for managing replication metadata, leader election, distributed DDL execution, and maintaining consistency across replicas.